first commit
This commit is contained in:
parent
6f5b9e3145
commit
713dd2cd90
675
README.md
675
README.md
|
@ -1,3 +1,674 @@
|
|||
# InternVL2_5-8B_a14123014239023104738976
|
||||
---
|
||||
license: mit
|
||||
pipeline_tag: image-text-to-text
|
||||
library_name: transformers
|
||||
base_model:
|
||||
- OpenGVLab/InternViT-300M-448px-V2_5
|
||||
- internlm/internlm2_5-7b-chat
|
||||
base_model_relation: merge
|
||||
language:
|
||||
- multilingual
|
||||
tags:
|
||||
- internvl
|
||||
- custom_code
|
||||
---
|
||||
|
||||
InternVL2_5-8B
|
||||
# InternVL2_5-8B
|
||||
|
||||
[\[📂 GitHub\]](https://github.com/OpenGVLab/InternVL) [\[📜 InternVL 1.0\]](https://huggingface.co/papers/2312.14238) [\[📜 InternVL 1.5\]](https://huggingface.co/papers/2404.16821) [\[📜 Mini-InternVL\]](https://arxiv.org/abs/2410.16261) [\[📜 InternVL 2.5\]](https://huggingface.co/papers/2412.05271)
|
||||
|
||||
[\[🆕 Blog\]](https://internvl.github.io/blog/) [\[🗨️ Chat Demo\]](https://internvl.opengvlab.com/) [\[🤗 HF Demo\]](https://huggingface.co/spaces/OpenGVLab/InternVL) [\[🚀 Quick Start\]](#quick-start) [\[📖 Documents\]](https://internvl.readthedocs.io/en/latest/)
|
||||
|
||||
<div align="center">
|
||||
<img width="500" alt="image" src="https://cdn-uploads.huggingface.co/production/uploads/64006c09330a45b03605bba3/zJsd2hqd3EevgXo6fNgC-.png">
|
||||
</div>
|
||||
|
||||
## Introduction
|
||||
|
||||
We are excited to introduce **InternVL 2.5**, an advanced multimodal large language model (MLLM) series that builds upon InternVL 2.0, maintaining its core model architecture while introducing significant enhancements in training and testing strategies as well as data quality.
|
||||
|
||||

|
||||
|
||||
## InternVL 2.5 Family
|
||||
|
||||
In the following table, we provide an overview of the InternVL 2.5 series.
|
||||
|
||||
| Model Name | Vision Part | Language Part | HF Link |
|
||||
| :-------------: | :-------------------------------------------------------------------------------------: | :----------------------------------------------------------------------------: | :---------------------------------------------------------: |
|
||||
| InternVL2_5-1B | [InternViT-300M-448px-V2_5](https://huggingface.co/OpenGVLab/InternViT-300M-448px-V2_5) | [Qwen2.5-0.5B-Instruct](https://huggingface.co/Qwen/Qwen2.5-0.5B-Instruct) | [🤗 link](https://huggingface.co/OpenGVLab/InternVL2_5-1B) |
|
||||
| InternVL2_5-2B | [InternViT-300M-448px-V2_5](https://huggingface.co/OpenGVLab/InternViT-300M-448px-V2_5) | [internlm2_5-1_8b-chat](https://huggingface.co/internlm/internlm2_5-1_8b-chat) | [🤗 link](https://huggingface.co/OpenGVLab/InternVL2_5-2B) |
|
||||
| InternVL2_5-4B | [InternViT-300M-448px-V2_5](https://huggingface.co/OpenGVLab/InternViT-300M-448px-V2_5) | [Qwen2.5-3B-Instruct](https://huggingface.co/Qwen/Qwen2.5-3B-Instruct) | [🤗 link](https://huggingface.co/OpenGVLab/InternVL2_5-4B) |
|
||||
| InternVL2_5-8B | [InternViT-300M-448px-V2_5](https://huggingface.co/OpenGVLab/InternViT-300M-448px-V2_5) | [internlm2_5-7b-chat](https://huggingface.co/internlm/internlm2_5-7b-chat) | [🤗 link](https://huggingface.co/OpenGVLab/InternVL2_5-8B) |
|
||||
| InternVL2_5-26B | [InternViT-6B-448px-V2_5](https://huggingface.co/OpenGVLab/InternViT-6B-448px-V2_5) | [internlm2_5-20b-chat](https://huggingface.co/internlm/internlm2_5-20b-chat) | [🤗 link](https://huggingface.co/OpenGVLab/InternVL2_5-26B) |
|
||||
| InternVL2_5-38B | [InternViT-6B-448px-V2_5](https://huggingface.co/OpenGVLab/InternViT-6B-448px-V2_5) | [Qwen2.5-32B-Instruct](https://huggingface.co/Qwen/Qwen2.5-32B-Instruct) | [🤗 link](https://huggingface.co/OpenGVLab/InternVL2_5-38B) |
|
||||
| InternVL2_5-78B | [InternViT-6B-448px-V2_5](https://huggingface.co/OpenGVLab/InternViT-6B-448px-V2_5) | [Qwen2.5-72B-Instruct](https://huggingface.co/Qwen/Qwen2.5-72B-Instruct) | [🤗 link](https://huggingface.co/OpenGVLab/InternVL2_5-78B) |
|
||||
|
||||
## Model Architecture
|
||||
|
||||
As shown in the following figure, InternVL 2.5 retains the same model architecture as its predecessors, InternVL 1.5 and 2.0, following the "ViT-MLP-LLM" paradigm. In this new version, we integrate a newly incrementally pre-trained InternViT with various pre-trained LLMs, including InternLM 2.5 and Qwen 2.5, using a randomly initialized MLP projector.
|
||||
|
||||

|
||||
|
||||
As in the previous version, we applied a pixel unshuffle operation, reducing the number of visual tokens to one-quarter of the original. Besides, we adopted a similar dynamic resolution strategy as InternVL 1.5, dividing images into tiles of 448×448 pixels. The key difference, starting from InternVL 2.0, is that we additionally introduced support for multi-image and video data.
|
||||
|
||||
## Training Strategy
|
||||
|
||||
### Dynamic High-Resolution for Multimodal Data
|
||||
|
||||
In InternVL 2.0 and 2.5, we extend the dynamic high-resolution training approach, enhancing its capabilities to handle multi-image and video datasets.
|
||||
|
||||

|
||||
|
||||
- For single-image datasets, the total number of tiles `n_max` are allocated to a single image for maximum resolution. Visual tokens are enclosed in `<img>` and `</img>` tags.
|
||||
|
||||
- For multi-image datasets, the total number of tiles `n_max` are distributed across all images in a sample. Each image is labeled with auxiliary tags like `Image-1` and enclosed in `<img>` and `</img>` tags.
|
||||
|
||||
- For videos, each frame is resized to 448×448. Frames are labeled with tags like `Frame-1` and enclosed in `<img>` and `</img>` tags, similar to images.
|
||||
|
||||
### Single Model Training Pipeline
|
||||
|
||||
The training pipeline for a single model in InternVL 2.5 is structured across three stages, designed to enhance the model's visual perception and multimodal capabilities.
|
||||
|
||||

|
||||
|
||||
- **Stage 1: MLP Warmup.** In this stage, only the MLP projector is trained while the vision encoder and language model are frozen. A dynamic high-resolution training strategy is applied for better performance, despite increased cost. This phase ensures robust cross-modal alignment and prepares the model for stable multimodal training.
|
||||
|
||||
- **Stage 1.5: ViT Incremental Learning (Optional).** This stage allows incremental training of the vision encoder and MLP projector using the same data as Stage 1. It enhances the encoder’s ability to handle rare domains like multilingual OCR and mathematical charts. Once trained, the encoder can be reused across LLMs without retraining, making this stage optional unless new domains are introduced.
|
||||
|
||||
- **Stage 2: Full Model Instruction Tuning.** The entire model is trained on high-quality multimodal instruction datasets. Strict data quality controls are enforced to prevent degradation of the LLM, as noisy data can cause issues like repetitive or incorrect outputs. After this stage, the training process is complete.
|
||||
|
||||
### Progressive Scaling Strategy
|
||||
|
||||
We introduce a progressive scaling strategy to align the vision encoder with LLMs efficiently. This approach trains with smaller LLMs first (e.g., 20B) to optimize foundational visual capabilities and cross-modal alignment before transferring the vision encoder to larger LLMs (e.g., 72B) without retraining. This reuse skips intermediate stages for larger models.
|
||||
|
||||

|
||||
|
||||
Compared to Qwen2-VL's 1.4 trillion tokens, InternVL2.5-78B uses only 120 billion tokens—less than one-tenth. This strategy minimizes redundancy, maximizes pre-trained component reuse, and enables efficient training for complex vision-language tasks.
|
||||
|
||||
### Training Enhancements
|
||||
|
||||
To improve real-world adaptability and performance, we introduce two key techniques:
|
||||
|
||||
- **Random JPEG Compression**: Random JPEG compression with quality levels between 75 and 100 is applied as a data augmentation technique. This simulates image degradation from internet sources, enhancing the model's robustness to noisy images.
|
||||
|
||||
- **Loss Reweighting**: To balance the NTP loss across responses of different lengths, we use a reweighting strategy called **square averaging**. This method balances contributions from responses of varying lengths, mitigating biases toward longer or shorter responses.
|
||||
|
||||
### Data Organization
|
||||
|
||||
#### Dataset Configuration
|
||||
|
||||
In InternVL 2.0 and 2.5, the organization of the training data is controlled by several key parameters to optimize the balance and distribution of datasets during training.
|
||||
|
||||

|
||||
|
||||
- **Data Augmentation:** JPEG compression is applied conditionally: enabled for image datasets to enhance robustness and disabled for video datasets to maintain consistent frame quality.
|
||||
|
||||
- **Maximum Tile Number:** The parameter `n_max` controls the maximum tiles per dataset. For example, higher values (24–36) are used for multi-image or high-resolution data, lower values (6–12) for standard images, and 1 for videos.
|
||||
|
||||
- **Repeat Factor:** The repeat factor `r` adjusts dataset sampling frequency. Values below 1 reduce a dataset's weight, while values above 1 increase it. This ensures balanced training across tasks and prevents overfitting or underfitting.
|
||||
|
||||
#### Data Filtering Pipeline
|
||||
|
||||
During development, we found that LLMs are highly sensitive to data noise, with even small anomalies—like outliers or repetitive data—causing abnormal behavior during inference. Repetitive generation, especially in long-form or CoT reasoning tasks, proved particularly harmful.
|
||||
|
||||

|
||||
|
||||
To address this challenge and support future research, we designed an efficient data filtering pipeline to remove low-quality samples.
|
||||
|
||||

|
||||
|
||||
The pipeline includes two modules, for **pure-text data**, three key strategies are used:
|
||||
|
||||
1. **LLM-Based Quality Scoring**: Each sample is scored (0–10) using a pre-trained LLM with domain-specific prompts. Samples scoring below a threshold (e.g., 7) are removed to ensure high-quality data.
|
||||
2. **Repetition Detection**: Repetitive samples are flagged using LLM-based prompts and manually reviewed. Samples scoring below a stricter threshold (e.g., 3) are excluded to avoid repetitive patterns.
|
||||
3. **Heuristic Rule-Based Filtering**: Anomalies like abnormal sentence lengths or duplicate lines are detected using rules. Flagged samples undergo manual verification to ensure accuracy before removal.
|
||||
|
||||
For **multimodal data**, two strategies are used:
|
||||
|
||||
1. **Repetition Detection**: Repetitive samples in non-academic datasets are flagged and manually reviewed to prevent pattern loops. High-quality datasets are exempt from this process.
|
||||
2. **Heuristic Rule-Based Filtering**: Similar rules are applied to detect visual anomalies, with flagged data verified manually to maintain integrity.
|
||||
|
||||
#### Training Data
|
||||
|
||||
As shown in the following figure, from InternVL 1.5 to 2.0 and then to 2.5, the fine-tuning data mixture has undergone iterative improvements in scale, quality, and diversity. For more information about the training data, please refer to our technical report.
|
||||
|
||||

|
||||
|
||||
## Evaluation on Multimodal Capability
|
||||
|
||||
### Multimodal Reasoning and Mathematics
|
||||
|
||||

|
||||
|
||||

|
||||
|
||||
### OCR, Chart, and Document Understanding
|
||||
|
||||

|
||||
|
||||
### Multi-Image & Real-World Comprehension
|
||||
|
||||

|
||||
|
||||
### Comprehensive Multimodal & Hallucination Evaluation
|
||||
|
||||

|
||||
|
||||
### Visual Grounding
|
||||
|
||||

|
||||
|
||||
### Multimodal Multilingual Understanding
|
||||
|
||||

|
||||
|
||||
### Video Understanding
|
||||
|
||||

|
||||
|
||||
## Evaluation on Language Capability
|
||||
|
||||
Training InternVL 2.0 models led to a decline in pure language capabilities. InternVL 2.5 addresses this by collecting more high-quality open-source data and filtering out low-quality data, achieving better preservation of pure language performance.
|
||||
|
||||
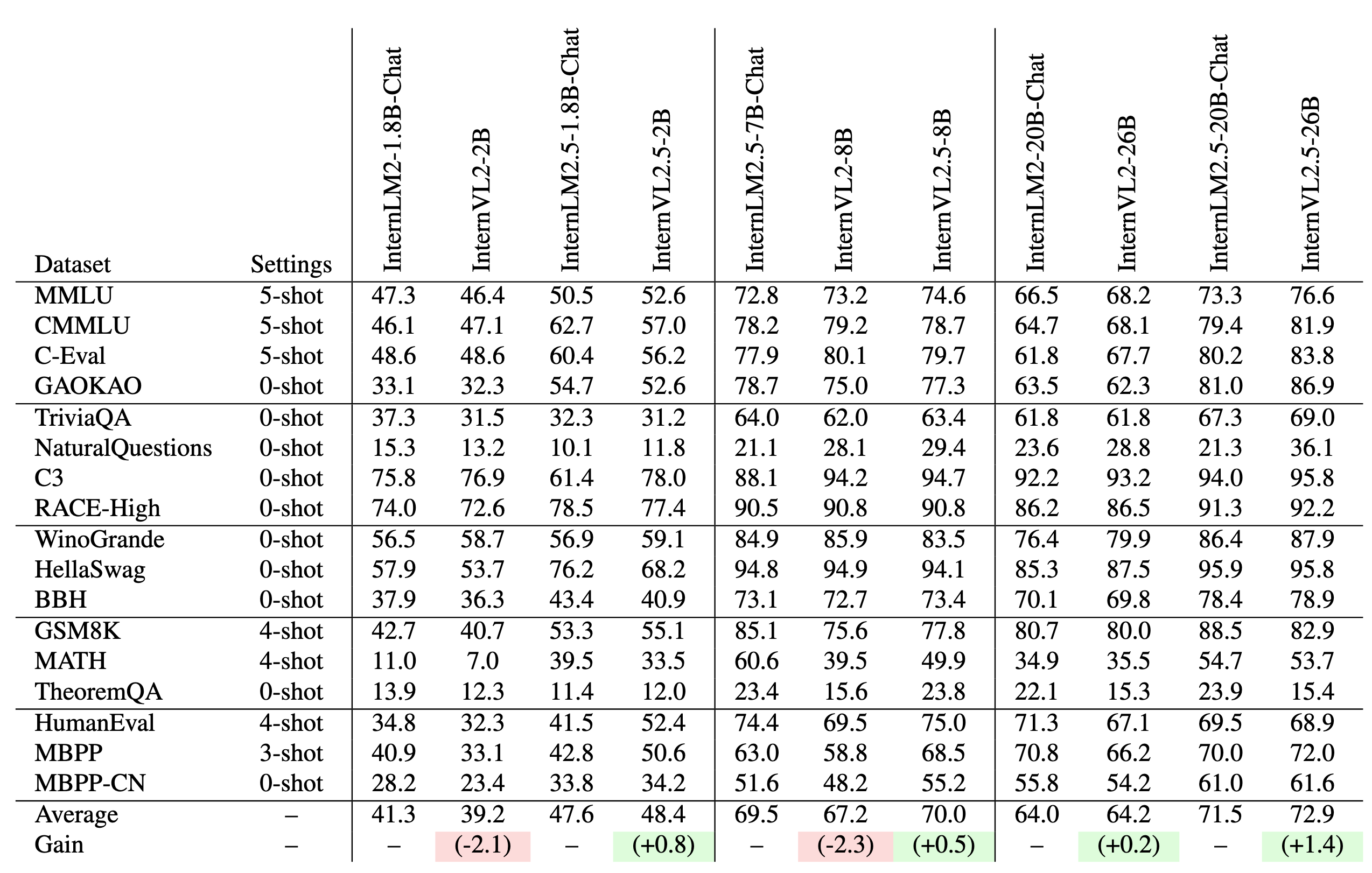
|
||||
|
||||
## Quick Start
|
||||
|
||||
We provide an example code to run `InternVL2_5-8B` using `transformers`.
|
||||
|
||||
> Please use transformers>=4.37.2 to ensure the model works normally.
|
||||
|
||||
### Model Loading
|
||||
|
||||
#### 16-bit (bf16 / fp16)
|
||||
|
||||
```python
|
||||
import torch
|
||||
from transformers import AutoTokenizer, AutoModel
|
||||
path = "OpenGVLab/InternVL2_5-8B"
|
||||
model = AutoModel.from_pretrained(
|
||||
path,
|
||||
torch_dtype=torch.bfloat16,
|
||||
low_cpu_mem_usage=True,
|
||||
use_flash_attn=True,
|
||||
trust_remote_code=True).eval().cuda()
|
||||
```
|
||||
|
||||
#### BNB 8-bit Quantization
|
||||
|
||||
```python
|
||||
import torch
|
||||
from transformers import AutoTokenizer, AutoModel
|
||||
path = "OpenGVLab/InternVL2_5-8B"
|
||||
model = AutoModel.from_pretrained(
|
||||
path,
|
||||
torch_dtype=torch.bfloat16,
|
||||
load_in_8bit=True,
|
||||
low_cpu_mem_usage=True,
|
||||
use_flash_attn=True,
|
||||
trust_remote_code=True).eval()
|
||||
```
|
||||
|
||||
#### Multiple GPUs
|
||||
|
||||
The reason for writing the code this way is to avoid errors that occur during multi-GPU inference due to tensors not being on the same device. By ensuring that the first and last layers of the large language model (LLM) are on the same device, we prevent such errors.
|
||||
|
||||
```python
|
||||
import math
|
||||
import torch
|
||||
from transformers import AutoTokenizer, AutoModel
|
||||
|
||||
def split_model(model_name):
|
||||
device_map = {}
|
||||
world_size = torch.cuda.device_count()
|
||||
num_layers = {
|
||||
'InternVL2_5-1B': 24, 'InternVL2_5-2B': 24, 'InternVL2_5-4B': 36, 'InternVL2_5-8B': 32,
|
||||
'InternVL2_5-26B': 48, 'InternVL2_5-38B': 64, 'InternVL2_5-78B': 80}[model_name]
|
||||
# Since the first GPU will be used for ViT, treat it as half a GPU.
|
||||
num_layers_per_gpu = math.ceil(num_layers / (world_size - 0.5))
|
||||
num_layers_per_gpu = [num_layers_per_gpu] * world_size
|
||||
num_layers_per_gpu[0] = math.ceil(num_layers_per_gpu[0] * 0.5)
|
||||
layer_cnt = 0
|
||||
for i, num_layer in enumerate(num_layers_per_gpu):
|
||||
for j in range(num_layer):
|
||||
device_map[f'language_model.model.layers.{layer_cnt}'] = i
|
||||
layer_cnt += 1
|
||||
device_map['vision_model'] = 0
|
||||
device_map['mlp1'] = 0
|
||||
device_map['language_model.model.tok_embeddings'] = 0
|
||||
device_map['language_model.model.embed_tokens'] = 0
|
||||
device_map['language_model.output'] = 0
|
||||
device_map['language_model.model.norm'] = 0
|
||||
device_map['language_model.lm_head'] = 0
|
||||
device_map[f'language_model.model.layers.{num_layers - 1}'] = 0
|
||||
|
||||
return device_map
|
||||
|
||||
path = "OpenGVLab/InternVL2_5-8B"
|
||||
device_map = split_model('InternVL2_5-8B')
|
||||
model = AutoModel.from_pretrained(
|
||||
path,
|
||||
torch_dtype=torch.bfloat16,
|
||||
low_cpu_mem_usage=True,
|
||||
use_flash_attn=True,
|
||||
trust_remote_code=True,
|
||||
device_map=device_map).eval()
|
||||
```
|
||||
|
||||
### Inference with Transformers
|
||||
|
||||
```python
|
||||
import numpy as np
|
||||
import torch
|
||||
import torchvision.transforms as T
|
||||
from decord import VideoReader, cpu
|
||||
from PIL import Image
|
||||
from torchvision.transforms.functional import InterpolationMode
|
||||
from transformers import AutoModel, AutoTokenizer
|
||||
|
||||
IMAGENET_MEAN = (0.485, 0.456, 0.406)
|
||||
IMAGENET_STD = (0.229, 0.224, 0.225)
|
||||
|
||||
def build_transform(input_size):
|
||||
MEAN, STD = IMAGENET_MEAN, IMAGENET_STD
|
||||
transform = T.Compose([
|
||||
T.Lambda(lambda img: img.convert('RGB') if img.mode != 'RGB' else img),
|
||||
T.Resize((input_size, input_size), interpolation=InterpolationMode.BICUBIC),
|
||||
T.ToTensor(),
|
||||
T.Normalize(mean=MEAN, std=STD)
|
||||
])
|
||||
return transform
|
||||
|
||||
def find_closest_aspect_ratio(aspect_ratio, target_ratios, width, height, image_size):
|
||||
best_ratio_diff = float('inf')
|
||||
best_ratio = (1, 1)
|
||||
area = width * height
|
||||
for ratio in target_ratios:
|
||||
target_aspect_ratio = ratio[0] / ratio[1]
|
||||
ratio_diff = abs(aspect_ratio - target_aspect_ratio)
|
||||
if ratio_diff < best_ratio_diff:
|
||||
best_ratio_diff = ratio_diff
|
||||
best_ratio = ratio
|
||||
elif ratio_diff == best_ratio_diff:
|
||||
if area > 0.5 * image_size * image_size * ratio[0] * ratio[1]:
|
||||
best_ratio = ratio
|
||||
return best_ratio
|
||||
|
||||
def dynamic_preprocess(image, min_num=1, max_num=12, image_size=448, use_thumbnail=False):
|
||||
orig_width, orig_height = image.size
|
||||
aspect_ratio = orig_width / orig_height
|
||||
|
||||
# calculate the existing image aspect ratio
|
||||
target_ratios = set(
|
||||
(i, j) for n in range(min_num, max_num + 1) for i in range(1, n + 1) for j in range(1, n + 1) if
|
||||
i * j <= max_num and i * j >= min_num)
|
||||
target_ratios = sorted(target_ratios, key=lambda x: x[0] * x[1])
|
||||
|
||||
# find the closest aspect ratio to the target
|
||||
target_aspect_ratio = find_closest_aspect_ratio(
|
||||
aspect_ratio, target_ratios, orig_width, orig_height, image_size)
|
||||
|
||||
# calculate the target width and height
|
||||
target_width = image_size * target_aspect_ratio[0]
|
||||
target_height = image_size * target_aspect_ratio[1]
|
||||
blocks = target_aspect_ratio[0] * target_aspect_ratio[1]
|
||||
|
||||
# resize the image
|
||||
resized_img = image.resize((target_width, target_height))
|
||||
processed_images = []
|
||||
for i in range(blocks):
|
||||
box = (
|
||||
(i % (target_width // image_size)) * image_size,
|
||||
(i // (target_width // image_size)) * image_size,
|
||||
((i % (target_width // image_size)) + 1) * image_size,
|
||||
((i // (target_width // image_size)) + 1) * image_size
|
||||
)
|
||||
# split the image
|
||||
split_img = resized_img.crop(box)
|
||||
processed_images.append(split_img)
|
||||
assert len(processed_images) == blocks
|
||||
if use_thumbnail and len(processed_images) != 1:
|
||||
thumbnail_img = image.resize((image_size, image_size))
|
||||
processed_images.append(thumbnail_img)
|
||||
return processed_images
|
||||
|
||||
def load_image(image_file, input_size=448, max_num=12):
|
||||
image = Image.open(image_file).convert('RGB')
|
||||
transform = build_transform(input_size=input_size)
|
||||
images = dynamic_preprocess(image, image_size=input_size, use_thumbnail=True, max_num=max_num)
|
||||
pixel_values = [transform(image) for image in images]
|
||||
pixel_values = torch.stack(pixel_values)
|
||||
return pixel_values
|
||||
|
||||
# If you want to load a model using multiple GPUs, please refer to the `Multiple GPUs` section.
|
||||
path = 'OpenGVLab/InternVL2_5-8B'
|
||||
model = AutoModel.from_pretrained(
|
||||
path,
|
||||
torch_dtype=torch.bfloat16,
|
||||
low_cpu_mem_usage=True,
|
||||
use_flash_attn=True,
|
||||
trust_remote_code=True).eval().cuda()
|
||||
tokenizer = AutoTokenizer.from_pretrained(path, trust_remote_code=True, use_fast=False)
|
||||
|
||||
# set the max number of tiles in `max_num`
|
||||
pixel_values = load_image('./examples/image1.jpg', max_num=12).to(torch.bfloat16).cuda()
|
||||
generation_config = dict(max_new_tokens=1024, do_sample=True)
|
||||
|
||||
# pure-text conversation (纯文本对话)
|
||||
question = 'Hello, who are you?'
|
||||
response, history = model.chat(tokenizer, None, question, generation_config, history=None, return_history=True)
|
||||
print(f'User: {question}\nAssistant: {response}')
|
||||
|
||||
question = 'Can you tell me a story?'
|
||||
response, history = model.chat(tokenizer, None, question, generation_config, history=history, return_history=True)
|
||||
print(f'User: {question}\nAssistant: {response}')
|
||||
|
||||
# single-image single-round conversation (单图单轮对话)
|
||||
question = '<image>\nPlease describe the image shortly.'
|
||||
response = model.chat(tokenizer, pixel_values, question, generation_config)
|
||||
print(f'User: {question}\nAssistant: {response}')
|
||||
|
||||
# single-image multi-round conversation (单图多轮对话)
|
||||
question = '<image>\nPlease describe the image in detail.'
|
||||
response, history = model.chat(tokenizer, pixel_values, question, generation_config, history=None, return_history=True)
|
||||
print(f'User: {question}\nAssistant: {response}')
|
||||
|
||||
question = 'Please write a poem according to the image.'
|
||||
response, history = model.chat(tokenizer, pixel_values, question, generation_config, history=history, return_history=True)
|
||||
print(f'User: {question}\nAssistant: {response}')
|
||||
|
||||
# multi-image multi-round conversation, combined images (多图多轮对话,拼接图像)
|
||||
pixel_values1 = load_image('./examples/image1.jpg', max_num=12).to(torch.bfloat16).cuda()
|
||||
pixel_values2 = load_image('./examples/image2.jpg', max_num=12).to(torch.bfloat16).cuda()
|
||||
pixel_values = torch.cat((pixel_values1, pixel_values2), dim=0)
|
||||
|
||||
question = '<image>\nDescribe the two images in detail.'
|
||||
response, history = model.chat(tokenizer, pixel_values, question, generation_config,
|
||||
history=None, return_history=True)
|
||||
print(f'User: {question}\nAssistant: {response}')
|
||||
|
||||
question = 'What are the similarities and differences between these two images.'
|
||||
response, history = model.chat(tokenizer, pixel_values, question, generation_config,
|
||||
history=history, return_history=True)
|
||||
print(f'User: {question}\nAssistant: {response}')
|
||||
|
||||
# multi-image multi-round conversation, separate images (多图多轮对话,独立图像)
|
||||
pixel_values1 = load_image('./examples/image1.jpg', max_num=12).to(torch.bfloat16).cuda()
|
||||
pixel_values2 = load_image('./examples/image2.jpg', max_num=12).to(torch.bfloat16).cuda()
|
||||
pixel_values = torch.cat((pixel_values1, pixel_values2), dim=0)
|
||||
num_patches_list = [pixel_values1.size(0), pixel_values2.size(0)]
|
||||
|
||||
question = 'Image-1: <image>\nImage-2: <image>\nDescribe the two images in detail.'
|
||||
response, history = model.chat(tokenizer, pixel_values, question, generation_config,
|
||||
num_patches_list=num_patches_list,
|
||||
history=None, return_history=True)
|
||||
print(f'User: {question}\nAssistant: {response}')
|
||||
|
||||
question = 'What are the similarities and differences between these two images.'
|
||||
response, history = model.chat(tokenizer, pixel_values, question, generation_config,
|
||||
num_patches_list=num_patches_list,
|
||||
history=history, return_history=True)
|
||||
print(f'User: {question}\nAssistant: {response}')
|
||||
|
||||
# batch inference, single image per sample (单图批处理)
|
||||
pixel_values1 = load_image('./examples/image1.jpg', max_num=12).to(torch.bfloat16).cuda()
|
||||
pixel_values2 = load_image('./examples/image2.jpg', max_num=12).to(torch.bfloat16).cuda()
|
||||
num_patches_list = [pixel_values1.size(0), pixel_values2.size(0)]
|
||||
pixel_values = torch.cat((pixel_values1, pixel_values2), dim=0)
|
||||
|
||||
questions = ['<image>\nDescribe the image in detail.'] * len(num_patches_list)
|
||||
responses = model.batch_chat(tokenizer, pixel_values,
|
||||
num_patches_list=num_patches_list,
|
||||
questions=questions,
|
||||
generation_config=generation_config)
|
||||
for question, response in zip(questions, responses):
|
||||
print(f'User: {question}\nAssistant: {response}')
|
||||
|
||||
# video multi-round conversation (视频多轮对话)
|
||||
def get_index(bound, fps, max_frame, first_idx=0, num_segments=32):
|
||||
if bound:
|
||||
start, end = bound[0], bound[1]
|
||||
else:
|
||||
start, end = -100000, 100000
|
||||
start_idx = max(first_idx, round(start * fps))
|
||||
end_idx = min(round(end * fps), max_frame)
|
||||
seg_size = float(end_idx - start_idx) / num_segments
|
||||
frame_indices = np.array([
|
||||
int(start_idx + (seg_size / 2) + np.round(seg_size * idx))
|
||||
for idx in range(num_segments)
|
||||
])
|
||||
return frame_indices
|
||||
|
||||
def load_video(video_path, bound=None, input_size=448, max_num=1, num_segments=32):
|
||||
vr = VideoReader(video_path, ctx=cpu(0), num_threads=1)
|
||||
max_frame = len(vr) - 1
|
||||
fps = float(vr.get_avg_fps())
|
||||
|
||||
pixel_values_list, num_patches_list = [], []
|
||||
transform = build_transform(input_size=input_size)
|
||||
frame_indices = get_index(bound, fps, max_frame, first_idx=0, num_segments=num_segments)
|
||||
for frame_index in frame_indices:
|
||||
img = Image.fromarray(vr[frame_index].asnumpy()).convert('RGB')
|
||||
img = dynamic_preprocess(img, image_size=input_size, use_thumbnail=True, max_num=max_num)
|
||||
pixel_values = [transform(tile) for tile in img]
|
||||
pixel_values = torch.stack(pixel_values)
|
||||
num_patches_list.append(pixel_values.shape[0])
|
||||
pixel_values_list.append(pixel_values)
|
||||
pixel_values = torch.cat(pixel_values_list)
|
||||
return pixel_values, num_patches_list
|
||||
|
||||
video_path = './examples/red-panda.mp4'
|
||||
pixel_values, num_patches_list = load_video(video_path, num_segments=8, max_num=1)
|
||||
pixel_values = pixel_values.to(torch.bfloat16).cuda()
|
||||
video_prefix = ''.join([f'Frame{i+1}: <image>\n' for i in range(len(num_patches_list))])
|
||||
question = video_prefix + 'What is the red panda doing?'
|
||||
# Frame1: <image>\nFrame2: <image>\n...\nFrame8: <image>\n{question}
|
||||
response, history = model.chat(tokenizer, pixel_values, question, generation_config,
|
||||
num_patches_list=num_patches_list, history=None, return_history=True)
|
||||
print(f'User: {question}\nAssistant: {response}')
|
||||
|
||||
question = 'Describe this video in detail.'
|
||||
response, history = model.chat(tokenizer, pixel_values, question, generation_config,
|
||||
num_patches_list=num_patches_list, history=history, return_history=True)
|
||||
print(f'User: {question}\nAssistant: {response}')
|
||||
```
|
||||
|
||||
#### Streaming Output
|
||||
|
||||
Besides this method, you can also use the following code to get streamed output.
|
||||
|
||||
```python
|
||||
from transformers import TextIteratorStreamer
|
||||
from threading import Thread
|
||||
|
||||
# Initialize the streamer
|
||||
streamer = TextIteratorStreamer(tokenizer, skip_prompt=True, skip_special_tokens=True, timeout=10)
|
||||
# Define the generation configuration
|
||||
generation_config = dict(max_new_tokens=1024, do_sample=False, streamer=streamer)
|
||||
# Start the model chat in a separate thread
|
||||
thread = Thread(target=model.chat, kwargs=dict(
|
||||
tokenizer=tokenizer, pixel_values=pixel_values, question=question,
|
||||
history=None, return_history=False, generation_config=generation_config,
|
||||
))
|
||||
thread.start()
|
||||
|
||||
# Initialize an empty string to store the generated text
|
||||
generated_text = ''
|
||||
# Loop through the streamer to get the new text as it is generated
|
||||
for new_text in streamer:
|
||||
if new_text == model.conv_template.sep:
|
||||
break
|
||||
generated_text += new_text
|
||||
print(new_text, end='', flush=True) # Print each new chunk of generated text on the same line
|
||||
```
|
||||
|
||||
## Finetune
|
||||
|
||||
Many repositories now support fine-tuning of the InternVL series models, including [InternVL](https://github.com/OpenGVLab/InternVL), [SWIFT](https://github.com/modelscope/ms-swift), [XTurner](https://github.com/InternLM/xtuner), and others. Please refer to their documentation for more details on fine-tuning.
|
||||
|
||||
## Deployment
|
||||
|
||||
### LMDeploy
|
||||
|
||||
LMDeploy is a toolkit for compressing, deploying, and serving LLMs & VLMs.
|
||||
|
||||
```sh
|
||||
pip install lmdeploy>=0.6.4
|
||||
```
|
||||
|
||||
LMDeploy abstracts the complex inference process of multi-modal Vision-Language Models (VLM) into an easy-to-use pipeline, similar to the Large Language Model (LLM) inference pipeline.
|
||||
|
||||
#### A 'Hello, world' Example
|
||||
|
||||
```python
|
||||
from lmdeploy import pipeline, TurbomindEngineConfig
|
||||
from lmdeploy.vl import load_image
|
||||
|
||||
model = 'OpenGVLab/InternVL2_5-8B'
|
||||
image = load_image('https://raw.githubusercontent.com/open-mmlab/mmdeploy/main/tests/data/tiger.jpeg')
|
||||
pipe = pipeline(model, backend_config=TurbomindEngineConfig(session_len=8192))
|
||||
response = pipe(('describe this image', image))
|
||||
print(response.text)
|
||||
```
|
||||
|
||||
If `ImportError` occurs while executing this case, please install the required dependency packages as prompted.
|
||||
|
||||
#### Multi-images Inference
|
||||
|
||||
When dealing with multiple images, you can put them all in one list. Keep in mind that multiple images will lead to a higher number of input tokens, and as a result, the size of the context window typically needs to be increased.
|
||||
|
||||
```python
|
||||
from lmdeploy import pipeline, TurbomindEngineConfig
|
||||
from lmdeploy.vl import load_image
|
||||
from lmdeploy.vl.constants import IMAGE_TOKEN
|
||||
|
||||
model = 'OpenGVLab/InternVL2_5-8B'
|
||||
pipe = pipeline(model, backend_config=TurbomindEngineConfig(session_len=8192))
|
||||
|
||||
image_urls=[
|
||||
'https://raw.githubusercontent.com/open-mmlab/mmdeploy/main/demo/resources/human-pose.jpg',
|
||||
'https://raw.githubusercontent.com/open-mmlab/mmdeploy/main/demo/resources/det.jpg'
|
||||
]
|
||||
|
||||
images = [load_image(img_url) for img_url in image_urls]
|
||||
# Numbering images improves multi-image conversations
|
||||
response = pipe((f'Image-1: {IMAGE_TOKEN}\nImage-2: {IMAGE_TOKEN}\ndescribe these two images', images))
|
||||
print(response.text)
|
||||
```
|
||||
|
||||
#### Batch Prompts Inference
|
||||
|
||||
Conducting inference with batch prompts is quite straightforward; just place them within a list structure:
|
||||
|
||||
```python
|
||||
from lmdeploy import pipeline, TurbomindEngineConfig
|
||||
from lmdeploy.vl import load_image
|
||||
|
||||
model = 'OpenGVLab/InternVL2_5-8B'
|
||||
pipe = pipeline(model, backend_config=TurbomindEngineConfig(session_len=8192))
|
||||
|
||||
image_urls=[
|
||||
"https://raw.githubusercontent.com/open-mmlab/mmdeploy/main/demo/resources/human-pose.jpg",
|
||||
"https://raw.githubusercontent.com/open-mmlab/mmdeploy/main/demo/resources/det.jpg"
|
||||
]
|
||||
prompts = [('describe this image', load_image(img_url)) for img_url in image_urls]
|
||||
response = pipe(prompts)
|
||||
print(response)
|
||||
```
|
||||
|
||||
#### Multi-turn Conversation
|
||||
|
||||
There are two ways to do the multi-turn conversations with the pipeline. One is to construct messages according to the format of OpenAI and use above introduced method, the other is to use the `pipeline.chat` interface.
|
||||
|
||||
```python
|
||||
from lmdeploy import pipeline, TurbomindEngineConfig, GenerationConfig
|
||||
from lmdeploy.vl import load_image
|
||||
|
||||
model = 'OpenGVLab/InternVL2_5-8B'
|
||||
pipe = pipeline(model, backend_config=TurbomindEngineConfig(session_len=8192))
|
||||
|
||||
image = load_image('https://raw.githubusercontent.com/open-mmlab/mmdeploy/main/demo/resources/human-pose.jpg')
|
||||
gen_config = GenerationConfig(top_k=40, top_p=0.8, temperature=0.8)
|
||||
sess = pipe.chat(('describe this image', image), gen_config=gen_config)
|
||||
print(sess.response.text)
|
||||
sess = pipe.chat('What is the woman doing?', session=sess, gen_config=gen_config)
|
||||
print(sess.response.text)
|
||||
```
|
||||
|
||||
#### Service
|
||||
|
||||
LMDeploy's `api_server` enables models to be easily packed into services with a single command. The provided RESTful APIs are compatible with OpenAI's interfaces. Below are an example of service startup:
|
||||
|
||||
```shell
|
||||
lmdeploy serve api_server OpenGVLab/InternVL2_5-8B --server-port 23333
|
||||
```
|
||||
|
||||
To use the OpenAI-style interface, you need to install OpenAI:
|
||||
|
||||
```shell
|
||||
pip install openai
|
||||
```
|
||||
|
||||
Then, use the code below to make the API call:
|
||||
|
||||
```python
|
||||
from openai import OpenAI
|
||||
|
||||
client = OpenAI(api_key='YOUR_API_KEY', base_url='http://0.0.0.0:23333/v1')
|
||||
model_name = client.models.list().data[0].id
|
||||
response = client.chat.completions.create(
|
||||
model=model_name,
|
||||
messages=[{
|
||||
'role':
|
||||
'user',
|
||||
'content': [{
|
||||
'type': 'text',
|
||||
'text': 'describe this image',
|
||||
}, {
|
||||
'type': 'image_url',
|
||||
'image_url': {
|
||||
'url':
|
||||
'https://modelscope.oss-cn-beijing.aliyuncs.com/resource/tiger.jpeg',
|
||||
},
|
||||
}],
|
||||
}],
|
||||
temperature=0.8,
|
||||
top_p=0.8)
|
||||
print(response)
|
||||
```
|
||||
|
||||
## License
|
||||
|
||||
This project is released under the MIT License. This project uses the pre-trained internlm2_5-7b-chat as a component, which is licensed under the Apache License 2.0.
|
||||
|
||||
## Citation
|
||||
|
||||
If you find this project useful in your research, please consider citing:
|
||||
|
||||
```BibTeX
|
||||
@article{chen2024expanding,
|
||||
title={Expanding Performance Boundaries of Open-Source Multimodal Models with Model, Data, and Test-Time Scaling},
|
||||
author={Chen, Zhe and Wang, Weiyun and Cao, Yue and Liu, Yangzhou and Gao, Zhangwei and Cui, Erfei and Zhu, Jinguo and Ye, Shenglong and Tian, Hao and Liu, Zhaoyang and others},
|
||||
journal={arXiv preprint arXiv:2412.05271},
|
||||
year={2024}
|
||||
}
|
||||
@article{gao2024mini,
|
||||
title={Mini-internvl: A flexible-transfer pocket multimodal model with 5\% parameters and 90\% performance},
|
||||
author={Gao, Zhangwei and Chen, Zhe and Cui, Erfei and Ren, Yiming and Wang, Weiyun and Zhu, Jinguo and Tian, Hao and Ye, Shenglong and He, Junjun and Zhu, Xizhou and others},
|
||||
journal={arXiv preprint arXiv:2410.16261},
|
||||
year={2024}
|
||||
}
|
||||
@article{chen2024far,
|
||||
title={How Far Are We to GPT-4V? Closing the Gap to Commercial Multimodal Models with Open-Source Suites},
|
||||
author={Chen, Zhe and Wang, Weiyun and Tian, Hao and Ye, Shenglong and Gao, Zhangwei and Cui, Erfei and Tong, Wenwen and Hu, Kongzhi and Luo, Jiapeng and Ma, Zheng and others},
|
||||
journal={arXiv preprint arXiv:2404.16821},
|
||||
year={2024}
|
||||
}
|
||||
@inproceedings{chen2024internvl,
|
||||
title={Internvl: Scaling up vision foundation models and aligning for generic visual-linguistic tasks},
|
||||
author={Chen, Zhe and Wu, Jiannan and Wang, Wenhai and Su, Weijie and Chen, Guo and Xing, Sen and Zhong, Muyan and Zhang, Qinglong and Zhu, Xizhou and Lu, Lewei and others},
|
||||
booktitle={Proceedings of the IEEE/CVF Conference on Computer Vision and Pattern Recognition},
|
||||
pages={24185--24198},
|
||||
year={2024}
|
||||
}
|
||||
```
|
||||
|
|
|
@ -0,0 +1,11 @@
|
|||
{
|
||||
"</box>": 92552,
|
||||
"</img>": 92545,
|
||||
"</quad>": 92548,
|
||||
"</ref>": 92550,
|
||||
"<IMG_CONTEXT>": 92546,
|
||||
"<box>": 92551,
|
||||
"<img>": 92544,
|
||||
"<quad>": 92547,
|
||||
"<ref>": 92549
|
||||
}
|
|
@ -0,0 +1,145 @@
|
|||
{
|
||||
"_commit_hash": null,
|
||||
"architectures": [
|
||||
"InternVLChatModel"
|
||||
],
|
||||
"auto_map": {
|
||||
"AutoConfig": "configuration_internvl_chat.InternVLChatConfig",
|
||||
"AutoModel": "modeling_internvl_chat.InternVLChatModel",
|
||||
"AutoModelForCausalLM": "modeling_internvl_chat.InternVLChatModel"
|
||||
},
|
||||
"downsample_ratio": 0.5,
|
||||
"dynamic_image_size": true,
|
||||
"force_image_size": 448,
|
||||
"llm_config": {
|
||||
"_name_or_path": "internlm/internlm2_5-7b-chat",
|
||||
"add_cross_attention": false,
|
||||
"architectures": [
|
||||
"InternLM2ForCausalLM"
|
||||
],
|
||||
"attn_implementation": "flash_attention_2",
|
||||
"auto_map": {
|
||||
"AutoConfig": "configuration_internlm2.InternLM2Config",
|
||||
"AutoModel": "modeling_internlm2.InternLM2ForCausalLM",
|
||||
"AutoModelForCausalLM": "modeling_internlm2.InternLM2ForCausalLM",
|
||||
"AutoModelForSequenceClassification": "modeling_internlm2.InternLM2ForSequenceClassification"
|
||||
},
|
||||
"bad_words_ids": null,
|
||||
"begin_suppress_tokens": null,
|
||||
"bias": false,
|
||||
"bos_token_id": 1,
|
||||
"chunk_size_feed_forward": 0,
|
||||
"cross_attention_hidden_size": null,
|
||||
"decoder_start_token_id": null,
|
||||
"diversity_penalty": 0.0,
|
||||
"do_sample": false,
|
||||
"early_stopping": false,
|
||||
"encoder_no_repeat_ngram_size": 0,
|
||||
"eos_token_id": 2,
|
||||
"exponential_decay_length_penalty": null,
|
||||
"finetuning_task": null,
|
||||
"forced_bos_token_id": null,
|
||||
"forced_eos_token_id": null,
|
||||
"hidden_act": "silu",
|
||||
"hidden_size": 4096,
|
||||
"id2label": {
|
||||
"0": "LABEL_0",
|
||||
"1": "LABEL_1"
|
||||
},
|
||||
"initializer_range": 0.02,
|
||||
"intermediate_size": 14336,
|
||||
"is_decoder": false,
|
||||
"is_encoder_decoder": false,
|
||||
"label2id": {
|
||||
"LABEL_0": 0,
|
||||
"LABEL_1": 1
|
||||
},
|
||||
"length_penalty": 1.0,
|
||||
"max_length": 20,
|
||||
"max_position_embeddings": 32768,
|
||||
"min_length": 0,
|
||||
"model_type": "internlm2",
|
||||
"no_repeat_ngram_size": 0,
|
||||
"num_attention_heads": 32,
|
||||
"num_beam_groups": 1,
|
||||
"num_beams": 1,
|
||||
"num_hidden_layers": 32,
|
||||
"num_key_value_heads": 8,
|
||||
"num_return_sequences": 1,
|
||||
"output_attentions": false,
|
||||
"output_hidden_states": false,
|
||||
"output_scores": false,
|
||||
"pad_token_id": 2,
|
||||
"prefix": null,
|
||||
"pretraining_tp": 1,
|
||||
"problem_type": null,
|
||||
"pruned_heads": {},
|
||||
"remove_invalid_values": false,
|
||||
"repetition_penalty": 1.0,
|
||||
"return_dict": true,
|
||||
"return_dict_in_generate": false,
|
||||
"rms_norm_eps": 1e-05,
|
||||
"rope_scaling": {
|
||||
"factor": 2.0,
|
||||
"type": "dynamic"
|
||||
},
|
||||
"rope_theta": 1000000,
|
||||
"sep_token_id": null,
|
||||
"suppress_tokens": null,
|
||||
"task_specific_params": null,
|
||||
"temperature": 1.0,
|
||||
"tf_legacy_loss": false,
|
||||
"tie_encoder_decoder": false,
|
||||
"tie_word_embeddings": false,
|
||||
"tokenizer_class": null,
|
||||
"top_k": 50,
|
||||
"top_p": 1.0,
|
||||
"torch_dtype": "bfloat16",
|
||||
"torchscript": false,
|
||||
"transformers_version": "4.37.2",
|
||||
"typical_p": 1.0,
|
||||
"use_bfloat16": true,
|
||||
"use_cache": true,
|
||||
"vocab_size": 92553
|
||||
},
|
||||
"max_dynamic_patch": 12,
|
||||
"min_dynamic_patch": 1,
|
||||
"model_type": "internvl_chat",
|
||||
"ps_version": "v2",
|
||||
"select_layer": -1,
|
||||
"template": "internvl2_5",
|
||||
"torch_dtype": "bfloat16",
|
||||
"use_backbone_lora": 0,
|
||||
"use_llm_lora": 0,
|
||||
"use_thumbnail": true,
|
||||
"vision_config": {
|
||||
"architectures": [
|
||||
"InternVisionModel"
|
||||
],
|
||||
"attention_dropout": 0.0,
|
||||
"drop_path_rate": 0.0,
|
||||
"dropout": 0.0,
|
||||
"hidden_act": "gelu",
|
||||
"hidden_size": 1024,
|
||||
"image_size": 448,
|
||||
"initializer_factor": 1.0,
|
||||
"initializer_range": 0.02,
|
||||
"intermediate_size": 4096,
|
||||
"layer_norm_eps": 1e-06,
|
||||
"model_type": "intern_vit_6b",
|
||||
"norm_type": "layer_norm",
|
||||
"num_attention_heads": 16,
|
||||
"num_channels": 3,
|
||||
"num_hidden_layers": 24,
|
||||
"output_attentions": false,
|
||||
"output_hidden_states": false,
|
||||
"patch_size": 14,
|
||||
"qk_normalization": false,
|
||||
"qkv_bias": true,
|
||||
"return_dict": true,
|
||||
"torch_dtype": "bfloat16",
|
||||
"transformers_version": "4.37.2",
|
||||
"use_bfloat16": true,
|
||||
"use_flash_attn": true
|
||||
}
|
||||
}
|
|
@ -0,0 +1 @@
|
|||
{"framework": "pytorch", "task": "image-text-to-text", "allow_remote": true}
|
|
@ -0,0 +1,120 @@
|
|||
# --------------------------------------------------------
|
||||
# InternVL
|
||||
# Copyright (c) 2024 OpenGVLab
|
||||
# Licensed under The MIT License [see LICENSE for details]
|
||||
# --------------------------------------------------------
|
||||
|
||||
import os
|
||||
from typing import Union
|
||||
|
||||
from transformers.configuration_utils import PretrainedConfig
|
||||
from transformers.utils import logging
|
||||
|
||||
logger = logging.get_logger(__name__)
|
||||
|
||||
|
||||
class InternVisionConfig(PretrainedConfig):
|
||||
r"""
|
||||
This is the configuration class to store the configuration of a [`InternVisionModel`]. It is used to
|
||||
instantiate a vision encoder according to the specified arguments, defining the model architecture.
|
||||
|
||||
Configuration objects inherit from [`PretrainedConfig`] and can be used to control the model outputs. Read the
|
||||
documentation from [`PretrainedConfig`] for more information.
|
||||
|
||||
Args:
|
||||
num_channels (`int`, *optional*, defaults to 3):
|
||||
Number of color channels in the input images (e.g., 3 for RGB).
|
||||
patch_size (`int`, *optional*, defaults to 14):
|
||||
The size (resolution) of each patch.
|
||||
image_size (`int`, *optional*, defaults to 224):
|
||||
The size (resolution) of each image.
|
||||
qkv_bias (`bool`, *optional*, defaults to `False`):
|
||||
Whether to add a bias to the queries and values in the self-attention layers.
|
||||
hidden_size (`int`, *optional*, defaults to 3200):
|
||||
Dimensionality of the encoder layers and the pooler layer.
|
||||
num_attention_heads (`int`, *optional*, defaults to 25):
|
||||
Number of attention heads for each attention layer in the Transformer encoder.
|
||||
intermediate_size (`int`, *optional*, defaults to 12800):
|
||||
Dimensionality of the "intermediate" (i.e., feed-forward) layer in the Transformer encoder.
|
||||
qk_normalization (`bool`, *optional*, defaults to `True`):
|
||||
Whether to normalize the queries and keys in the self-attention layers.
|
||||
num_hidden_layers (`int`, *optional*, defaults to 48):
|
||||
Number of hidden layers in the Transformer encoder.
|
||||
use_flash_attn (`bool`, *optional*, defaults to `True`):
|
||||
Whether to use flash attention mechanism.
|
||||
hidden_act (`str` or `function`, *optional*, defaults to `"gelu"`):
|
||||
The non-linear activation function (function or string) in the encoder and pooler. If string, `"gelu"`,
|
||||
`"relu"`, `"selu"` and `"gelu_new"` ``"gelu"` are supported.
|
||||
layer_norm_eps (`float`, *optional*, defaults to 1e-6):
|
||||
The epsilon used by the layer normalization layers.
|
||||
dropout (`float`, *optional*, defaults to 0.0):
|
||||
The dropout probability for all fully connected layers in the embeddings, encoder, and pooler.
|
||||
drop_path_rate (`float`, *optional*, defaults to 0.0):
|
||||
Dropout rate for stochastic depth.
|
||||
attention_dropout (`float`, *optional*, defaults to 0.0):
|
||||
The dropout ratio for the attention probabilities.
|
||||
initializer_range (`float`, *optional*, defaults to 0.02):
|
||||
The standard deviation of the truncated_normal_initializer for initializing all weight matrices.
|
||||
initializer_factor (`float`, *optional*, defaults to 0.1):
|
||||
A factor for layer scale.
|
||||
"""
|
||||
|
||||
model_type = 'intern_vit_6b'
|
||||
|
||||
def __init__(
|
||||
self,
|
||||
num_channels=3,
|
||||
patch_size=14,
|
||||
image_size=224,
|
||||
qkv_bias=False,
|
||||
hidden_size=3200,
|
||||
num_attention_heads=25,
|
||||
intermediate_size=12800,
|
||||
qk_normalization=True,
|
||||
num_hidden_layers=48,
|
||||
use_flash_attn=True,
|
||||
hidden_act='gelu',
|
||||
norm_type='rms_norm',
|
||||
layer_norm_eps=1e-6,
|
||||
dropout=0.0,
|
||||
drop_path_rate=0.0,
|
||||
attention_dropout=0.0,
|
||||
initializer_range=0.02,
|
||||
initializer_factor=0.1,
|
||||
**kwargs,
|
||||
):
|
||||
super().__init__(**kwargs)
|
||||
|
||||
self.hidden_size = hidden_size
|
||||
self.intermediate_size = intermediate_size
|
||||
self.dropout = dropout
|
||||
self.drop_path_rate = drop_path_rate
|
||||
self.num_hidden_layers = num_hidden_layers
|
||||
self.num_attention_heads = num_attention_heads
|
||||
self.num_channels = num_channels
|
||||
self.patch_size = patch_size
|
||||
self.image_size = image_size
|
||||
self.initializer_range = initializer_range
|
||||
self.initializer_factor = initializer_factor
|
||||
self.attention_dropout = attention_dropout
|
||||
self.layer_norm_eps = layer_norm_eps
|
||||
self.hidden_act = hidden_act
|
||||
self.norm_type = norm_type
|
||||
self.qkv_bias = qkv_bias
|
||||
self.qk_normalization = qk_normalization
|
||||
self.use_flash_attn = use_flash_attn
|
||||
|
||||
@classmethod
|
||||
def from_pretrained(cls, pretrained_model_name_or_path: Union[str, os.PathLike], **kwargs) -> 'PretrainedConfig':
|
||||
config_dict, kwargs = cls.get_config_dict(pretrained_model_name_or_path, **kwargs)
|
||||
|
||||
if 'vision_config' in config_dict:
|
||||
config_dict = config_dict['vision_config']
|
||||
|
||||
if 'model_type' in config_dict and hasattr(cls, 'model_type') and config_dict['model_type'] != cls.model_type:
|
||||
logger.warning(
|
||||
f"You are using a model of type {config_dict['model_type']} to instantiate a model of type "
|
||||
f'{cls.model_type}. This is not supported for all configurations of models and can yield errors.'
|
||||
)
|
||||
|
||||
return cls.from_dict(config_dict, **kwargs)
|
|
@ -0,0 +1,150 @@
|
|||
# Copyright (c) The InternLM team and The HuggingFace Inc. team. All rights reserved.
|
||||
#
|
||||
# This code is based on transformers/src/transformers/models/llama/configuration_llama.py
|
||||
#
|
||||
# Licensed under the Apache License, Version 2.0 (the "License");
|
||||
# you may not use this file except in compliance with the License.
|
||||
# You may obtain a copy of the License at
|
||||
#
|
||||
# http://www.apache.org/licenses/LICENSE-2.0
|
||||
#
|
||||
# Unless required by applicable law or agreed to in writing, software
|
||||
# distributed under the License is distributed on an "AS IS" BASIS,
|
||||
# WITHOUT WARRANTIES OR CONDITIONS OF ANY KIND, either express or implied.
|
||||
# See the License for the specific language governing permissions and
|
||||
# limitations under the License.
|
||||
""" InternLM2 model configuration"""
|
||||
|
||||
from transformers.configuration_utils import PretrainedConfig
|
||||
from transformers.utils import logging
|
||||
|
||||
logger = logging.get_logger(__name__)
|
||||
|
||||
INTERNLM2_PRETRAINED_CONFIG_ARCHIVE_MAP = {}
|
||||
|
||||
|
||||
# Modified from transformers.model.llama.configuration_llama.LlamaConfig
|
||||
class InternLM2Config(PretrainedConfig):
|
||||
r"""
|
||||
This is the configuration class to store the configuration of a [`InternLM2Model`]. It is used to instantiate
|
||||
an InternLM2 model according to the specified arguments, defining the model architecture. Instantiating a
|
||||
configuration with the defaults will yield a similar configuration to that of the InternLM2-7B.
|
||||
|
||||
Configuration objects inherit from [`PretrainedConfig`] and can be used to control the model outputs. Read the
|
||||
documentation from [`PretrainedConfig`] for more information.
|
||||
|
||||
|
||||
Args:
|
||||
vocab_size (`int`, *optional*, defaults to 32000):
|
||||
Vocabulary size of the InternLM2 model. Defines the number of different tokens that can be represented by the
|
||||
`inputs_ids` passed when calling [`InternLM2Model`]
|
||||
hidden_size (`int`, *optional*, defaults to 4096):
|
||||
Dimension of the hidden representations.
|
||||
intermediate_size (`int`, *optional*, defaults to 11008):
|
||||
Dimension of the MLP representations.
|
||||
num_hidden_layers (`int`, *optional*, defaults to 32):
|
||||
Number of hidden layers in the Transformer encoder.
|
||||
num_attention_heads (`int`, *optional*, defaults to 32):
|
||||
Number of attention heads for each attention layer in the Transformer encoder.
|
||||
num_key_value_heads (`int`, *optional*):
|
||||
This is the number of key_value heads that should be used to implement Grouped Query Attention. If
|
||||
`num_key_value_heads=num_attention_heads`, the model will use Multi Head Attention (MHA), if
|
||||
`num_key_value_heads=1 the model will use Multi Query Attention (MQA) otherwise GQA is used. When
|
||||
converting a multi-head checkpoint to a GQA checkpoint, each group key and value head should be constructed
|
||||
by meanpooling all the original heads within that group. For more details checkout [this
|
||||
paper](https://arxiv.org/pdf/2305.13245.pdf). If it is not specified, will default to
|
||||
`num_attention_heads`.
|
||||
hidden_act (`str` or `function`, *optional*, defaults to `"silu"`):
|
||||
The non-linear activation function (function or string) in the decoder.
|
||||
max_position_embeddings (`int`, *optional*, defaults to 2048):
|
||||
The maximum sequence length that this model might ever be used with. Typically set this to something large
|
||||
just in case (e.g., 512 or 1024 or 2048).
|
||||
initializer_range (`float`, *optional*, defaults to 0.02):
|
||||
The standard deviation of the truncated_normal_initializer for initializing all weight matrices.
|
||||
rms_norm_eps (`float`, *optional*, defaults to 1e-12):
|
||||
The epsilon used by the rms normalization layers.
|
||||
use_cache (`bool`, *optional*, defaults to `True`):
|
||||
Whether or not the model should return the last key/values attentions (not used by all models). Only
|
||||
relevant if `config.is_decoder=True`.
|
||||
tie_word_embeddings(`bool`, *optional*, defaults to `False`):
|
||||
Whether to tie weight embeddings
|
||||
Example:
|
||||
|
||||
"""
|
||||
model_type = 'internlm2'
|
||||
_auto_class = 'AutoConfig'
|
||||
|
||||
def __init__( # pylint: disable=W0102
|
||||
self,
|
||||
vocab_size=103168,
|
||||
hidden_size=4096,
|
||||
intermediate_size=11008,
|
||||
num_hidden_layers=32,
|
||||
num_attention_heads=32,
|
||||
num_key_value_heads=None,
|
||||
hidden_act='silu',
|
||||
max_position_embeddings=2048,
|
||||
initializer_range=0.02,
|
||||
rms_norm_eps=1e-6,
|
||||
use_cache=True,
|
||||
pad_token_id=0,
|
||||
bos_token_id=1,
|
||||
eos_token_id=2,
|
||||
tie_word_embeddings=False,
|
||||
bias=True,
|
||||
rope_theta=10000,
|
||||
rope_scaling=None,
|
||||
attn_implementation='eager',
|
||||
**kwargs,
|
||||
):
|
||||
self.vocab_size = vocab_size
|
||||
self.max_position_embeddings = max_position_embeddings
|
||||
self.hidden_size = hidden_size
|
||||
self.intermediate_size = intermediate_size
|
||||
self.num_hidden_layers = num_hidden_layers
|
||||
self.num_attention_heads = num_attention_heads
|
||||
self.bias = bias
|
||||
|
||||
if num_key_value_heads is None:
|
||||
num_key_value_heads = num_attention_heads
|
||||
self.num_key_value_heads = num_key_value_heads
|
||||
|
||||
self.hidden_act = hidden_act
|
||||
self.initializer_range = initializer_range
|
||||
self.rms_norm_eps = rms_norm_eps
|
||||
self.use_cache = use_cache
|
||||
self.rope_theta = rope_theta
|
||||
self.rope_scaling = rope_scaling
|
||||
self._rope_scaling_validation()
|
||||
|
||||
self.attn_implementation = attn_implementation
|
||||
if self.attn_implementation is None:
|
||||
self.attn_implementation = 'eager'
|
||||
super().__init__(
|
||||
pad_token_id=pad_token_id,
|
||||
bos_token_id=bos_token_id,
|
||||
eos_token_id=eos_token_id,
|
||||
tie_word_embeddings=tie_word_embeddings,
|
||||
**kwargs,
|
||||
)
|
||||
|
||||
def _rope_scaling_validation(self):
|
||||
"""
|
||||
Validate the `rope_scaling` configuration.
|
||||
"""
|
||||
if self.rope_scaling is None:
|
||||
return
|
||||
|
||||
if not isinstance(self.rope_scaling, dict) or len(self.rope_scaling) != 2:
|
||||
raise ValueError(
|
||||
'`rope_scaling` must be a dictionary with with two fields, `type` and `factor`, '
|
||||
f'got {self.rope_scaling}'
|
||||
)
|
||||
rope_scaling_type = self.rope_scaling.get('type', None)
|
||||
rope_scaling_factor = self.rope_scaling.get('factor', None)
|
||||
if rope_scaling_type is None or rope_scaling_type not in ['linear', 'dynamic']:
|
||||
raise ValueError(
|
||||
f"`rope_scaling`'s type field must be one of ['linear', 'dynamic'], got {rope_scaling_type}"
|
||||
)
|
||||
if rope_scaling_factor is None or not isinstance(rope_scaling_factor, float) or rope_scaling_factor < 1.0:
|
||||
raise ValueError(f"`rope_scaling`'s factor field must be a float >= 1, got {rope_scaling_factor}")
|
|
@ -0,0 +1,96 @@
|
|||
# --------------------------------------------------------
|
||||
# InternVL
|
||||
# Copyright (c) 2024 OpenGVLab
|
||||
# Licensed under The MIT License [see LICENSE for details]
|
||||
# --------------------------------------------------------
|
||||
|
||||
import copy
|
||||
|
||||
from transformers import AutoConfig, LlamaConfig
|
||||
from transformers.configuration_utils import PretrainedConfig
|
||||
from transformers.utils import logging
|
||||
|
||||
from .configuration_intern_vit import InternVisionConfig
|
||||
from .configuration_internlm2 import InternLM2Config
|
||||
|
||||
logger = logging.get_logger(__name__)
|
||||
|
||||
|
||||
class InternVLChatConfig(PretrainedConfig):
|
||||
model_type = 'internvl_chat'
|
||||
is_composition = True
|
||||
|
||||
def __init__(
|
||||
self,
|
||||
vision_config=None,
|
||||
llm_config=None,
|
||||
use_backbone_lora=0,
|
||||
use_llm_lora=0,
|
||||
select_layer=-1,
|
||||
force_image_size=None,
|
||||
downsample_ratio=0.5,
|
||||
template=None,
|
||||
dynamic_image_size=False,
|
||||
use_thumbnail=False,
|
||||
ps_version='v1',
|
||||
min_dynamic_patch=1,
|
||||
max_dynamic_patch=6,
|
||||
**kwargs):
|
||||
super().__init__(**kwargs)
|
||||
|
||||
if vision_config is None:
|
||||
vision_config = {'architectures': ['InternVisionModel']}
|
||||
logger.info('vision_config is None. Initializing the InternVisionConfig with default values.')
|
||||
|
||||
if llm_config is None:
|
||||
llm_config = {'architectures': ['InternLM2ForCausalLM']}
|
||||
logger.info('llm_config is None. Initializing the LlamaConfig config with default values (`LlamaConfig`).')
|
||||
|
||||
self.vision_config = InternVisionConfig(**vision_config)
|
||||
if llm_config.get('architectures')[0] == 'LlamaForCausalLM':
|
||||
self.llm_config = LlamaConfig(**llm_config)
|
||||
elif llm_config.get('architectures')[0] == 'InternLM2ForCausalLM':
|
||||
self.llm_config = InternLM2Config(**llm_config)
|
||||
else:
|
||||
raise ValueError('Unsupported architecture: {}'.format(llm_config.get('architectures')[0]))
|
||||
self.use_backbone_lora = use_backbone_lora
|
||||
self.use_llm_lora = use_llm_lora
|
||||
self.select_layer = select_layer
|
||||
self.force_image_size = force_image_size
|
||||
self.downsample_ratio = downsample_ratio
|
||||
self.template = template
|
||||
self.dynamic_image_size = dynamic_image_size
|
||||
self.use_thumbnail = use_thumbnail
|
||||
self.ps_version = ps_version # pixel shuffle version
|
||||
self.min_dynamic_patch = min_dynamic_patch
|
||||
self.max_dynamic_patch = max_dynamic_patch
|
||||
|
||||
logger.info(f'vision_select_layer: {self.select_layer}')
|
||||
logger.info(f'ps_version: {self.ps_version}')
|
||||
logger.info(f'min_dynamic_patch: {self.min_dynamic_patch}')
|
||||
logger.info(f'max_dynamic_patch: {self.max_dynamic_patch}')
|
||||
|
||||
def to_dict(self):
|
||||
"""
|
||||
Serializes this instance to a Python dictionary. Override the default [`~PretrainedConfig.to_dict`].
|
||||
|
||||
Returns:
|
||||
`Dict[str, any]`: Dictionary of all the attributes that make up this configuration instance,
|
||||
"""
|
||||
output = copy.deepcopy(self.__dict__)
|
||||
output['vision_config'] = self.vision_config.to_dict()
|
||||
output['llm_config'] = self.llm_config.to_dict()
|
||||
output['model_type'] = self.__class__.model_type
|
||||
output['use_backbone_lora'] = self.use_backbone_lora
|
||||
output['use_llm_lora'] = self.use_llm_lora
|
||||
output['select_layer'] = self.select_layer
|
||||
output['force_image_size'] = self.force_image_size
|
||||
output['downsample_ratio'] = self.downsample_ratio
|
||||
output['template'] = self.template
|
||||
output['dynamic_image_size'] = self.dynamic_image_size
|
||||
output['use_thumbnail'] = self.use_thumbnail
|
||||
output['ps_version'] = self.ps_version
|
||||
output['min_dynamic_patch'] = self.min_dynamic_patch
|
||||
output['max_dynamic_patch'] = self.max_dynamic_patch
|
||||
|
||||
return output
|
|
@ -0,0 +1,391 @@
|
|||
"""
|
||||
Conversation prompt templates.
|
||||
|
||||
We kindly request that you import fastchat instead of copying this file if you wish to use it.
|
||||
If you have changes in mind, please contribute back so the community can benefit collectively and continue to maintain these valuable templates.
|
||||
|
||||
Modified from https://github.com/lm-sys/FastChat/blob/main/fastchat/conversation.py
|
||||
"""
|
||||
|
||||
import dataclasses
|
||||
from enum import IntEnum, auto
|
||||
from typing import Dict, List, Tuple, Union
|
||||
|
||||
|
||||
class SeparatorStyle(IntEnum):
|
||||
"""Separator styles."""
|
||||
|
||||
ADD_COLON_SINGLE = auto()
|
||||
ADD_COLON_TWO = auto()
|
||||
ADD_COLON_SPACE_SINGLE = auto()
|
||||
NO_COLON_SINGLE = auto()
|
||||
NO_COLON_TWO = auto()
|
||||
ADD_NEW_LINE_SINGLE = auto()
|
||||
LLAMA2 = auto()
|
||||
CHATGLM = auto()
|
||||
CHATML = auto()
|
||||
CHATINTERN = auto()
|
||||
DOLLY = auto()
|
||||
RWKV = auto()
|
||||
PHOENIX = auto()
|
||||
ROBIN = auto()
|
||||
FALCON_CHAT = auto()
|
||||
CHATGLM3 = auto()
|
||||
INTERNVL_ZH = auto()
|
||||
MPT = auto()
|
||||
|
||||
|
||||
@dataclasses.dataclass
|
||||
class Conversation:
|
||||
"""A class that manages prompt templates and keeps all conversation history."""
|
||||
|
||||
# The name of this template
|
||||
name: str
|
||||
# The template of the system prompt
|
||||
system_template: str = '{system_message}'
|
||||
# The system message
|
||||
system_message: str = ''
|
||||
# The names of two roles
|
||||
roles: Tuple[str] = ('USER', 'ASSISTANT')
|
||||
# All messages. Each item is (role, message).
|
||||
messages: List[List[str]] = ()
|
||||
# The number of few shot examples
|
||||
offset: int = 0
|
||||
# The separator style and configurations
|
||||
sep_style: SeparatorStyle = SeparatorStyle.ADD_COLON_SINGLE
|
||||
sep: str = '\n'
|
||||
sep2: str = None
|
||||
# Stop criteria (the default one is EOS token)
|
||||
stop_str: Union[str, List[str]] = None
|
||||
# Stops generation if meeting any token in this list
|
||||
stop_token_ids: List[int] = None
|
||||
|
||||
def get_prompt(self) -> str:
|
||||
"""Get the prompt for generation."""
|
||||
system_prompt = self.system_template.format(system_message=self.system_message)
|
||||
if self.sep_style == SeparatorStyle.ADD_COLON_SINGLE:
|
||||
ret = system_prompt + self.sep
|
||||
for role, message in self.messages:
|
||||
if message:
|
||||
ret += role + ': ' + message + self.sep
|
||||
else:
|
||||
ret += role + ':'
|
||||
return ret
|
||||
elif self.sep_style == SeparatorStyle.ADD_COLON_TWO:
|
||||
seps = [self.sep, self.sep2]
|
||||
ret = system_prompt + seps[0]
|
||||
for i, (role, message) in enumerate(self.messages):
|
||||
if message:
|
||||
ret += role + ': ' + message + seps[i % 2]
|
||||
else:
|
||||
ret += role + ':'
|
||||
return ret
|
||||
elif self.sep_style == SeparatorStyle.ADD_COLON_SPACE_SINGLE:
|
||||
ret = system_prompt + self.sep
|
||||
for role, message in self.messages:
|
||||
if message:
|
||||
ret += role + ': ' + message + self.sep
|
||||
else:
|
||||
ret += role + ': ' # must be end with a space
|
||||
return ret
|
||||
elif self.sep_style == SeparatorStyle.ADD_NEW_LINE_SINGLE:
|
||||
ret = '' if system_prompt == '' else system_prompt + self.sep
|
||||
for role, message in self.messages:
|
||||
if message:
|
||||
ret += role + '\n' + message + self.sep
|
||||
else:
|
||||
ret += role + '\n'
|
||||
return ret
|
||||
elif self.sep_style == SeparatorStyle.NO_COLON_SINGLE:
|
||||
ret = system_prompt
|
||||
for role, message in self.messages:
|
||||
if message:
|
||||
ret += role + message + self.sep
|
||||
else:
|
||||
ret += role
|
||||
return ret
|
||||
elif self.sep_style == SeparatorStyle.NO_COLON_TWO:
|
||||
seps = [self.sep, self.sep2]
|
||||
ret = system_prompt
|
||||
for i, (role, message) in enumerate(self.messages):
|
||||
if message:
|
||||
ret += role + message + seps[i % 2]
|
||||
else:
|
||||
ret += role
|
||||
return ret
|
||||
elif self.sep_style == SeparatorStyle.RWKV:
|
||||
ret = system_prompt
|
||||
for i, (role, message) in enumerate(self.messages):
|
||||
if message:
|
||||
ret += (
|
||||
role
|
||||
+ ': '
|
||||
+ message.replace('\r\n', '\n').replace('\n\n', '\n')
|
||||
)
|
||||
ret += '\n\n'
|
||||
else:
|
||||
ret += role + ':'
|
||||
return ret
|
||||
elif self.sep_style == SeparatorStyle.LLAMA2:
|
||||
seps = [self.sep, self.sep2]
|
||||
if self.system_message:
|
||||
ret = system_prompt
|
||||
else:
|
||||
ret = '[INST] '
|
||||
for i, (role, message) in enumerate(self.messages):
|
||||
tag = self.roles[i % 2]
|
||||
if message:
|
||||
if i == 0:
|
||||
ret += message + ' '
|
||||
else:
|
||||
ret += tag + ' ' + message + seps[i % 2]
|
||||
else:
|
||||
ret += tag
|
||||
return ret
|
||||
elif self.sep_style == SeparatorStyle.CHATGLM:
|
||||
# source: https://huggingface.co/THUDM/chatglm-6b/blob/1d240ba371910e9282298d4592532d7f0f3e9f3e/modeling_chatglm.py#L1302-L1308
|
||||
# source2: https://huggingface.co/THUDM/chatglm2-6b/blob/e186c891cf64310ac66ef10a87e6635fa6c2a579/modeling_chatglm.py#L926
|
||||
round_add_n = 1 if self.name == 'chatglm2' else 0
|
||||
if system_prompt:
|
||||
ret = system_prompt + self.sep
|
||||
else:
|
||||
ret = ''
|
||||
|
||||
for i, (role, message) in enumerate(self.messages):
|
||||
if i % 2 == 0:
|
||||
ret += f'[Round {i//2 + round_add_n}]{self.sep}'
|
||||
|
||||
if message:
|
||||
ret += f'{role}:{message}{self.sep}'
|
||||
else:
|
||||
ret += f'{role}:'
|
||||
return ret
|
||||
elif self.sep_style == SeparatorStyle.CHATML:
|
||||
ret = '' if system_prompt == '' else system_prompt + self.sep + '\n'
|
||||
for role, message in self.messages:
|
||||
if message:
|
||||
ret += role + '\n' + message + self.sep + '\n'
|
||||
else:
|
||||
ret += role + '\n'
|
||||
return ret
|
||||
elif self.sep_style == SeparatorStyle.CHATGLM3:
|
||||
ret = ''
|
||||
if self.system_message:
|
||||
ret += system_prompt
|
||||
for role, message in self.messages:
|
||||
if message:
|
||||
ret += role + '\n' + ' ' + message
|
||||
else:
|
||||
ret += role
|
||||
return ret
|
||||
elif self.sep_style == SeparatorStyle.CHATINTERN:
|
||||
# source: https://huggingface.co/internlm/internlm-chat-7b-8k/blob/bd546fa984b4b0b86958f56bf37f94aa75ab8831/modeling_internlm.py#L771
|
||||
seps = [self.sep, self.sep2]
|
||||
ret = system_prompt
|
||||
for i, (role, message) in enumerate(self.messages):
|
||||
# if i % 2 == 0:
|
||||
# ret += "<s>"
|
||||
if message:
|
||||
ret += role + ':' + message + seps[i % 2] + '\n'
|
||||
else:
|
||||
ret += role + ':'
|
||||
return ret
|
||||
elif self.sep_style == SeparatorStyle.DOLLY:
|
||||
seps = [self.sep, self.sep2]
|
||||
ret = system_prompt
|
||||
for i, (role, message) in enumerate(self.messages):
|
||||
if message:
|
||||
ret += role + ':\n' + message + seps[i % 2]
|
||||
if i % 2 == 1:
|
||||
ret += '\n\n'
|
||||
else:
|
||||
ret += role + ':\n'
|
||||
return ret
|
||||
elif self.sep_style == SeparatorStyle.PHOENIX:
|
||||
ret = system_prompt
|
||||
for role, message in self.messages:
|
||||
if message:
|
||||
ret += role + ': ' + '<s>' + message + '</s>'
|
||||
else:
|
||||
ret += role + ': ' + '<s>'
|
||||
return ret
|
||||
elif self.sep_style == SeparatorStyle.ROBIN:
|
||||
ret = system_prompt + self.sep
|
||||
for role, message in self.messages:
|
||||
if message:
|
||||
ret += role + ':\n' + message + self.sep
|
||||
else:
|
||||
ret += role + ':\n'
|
||||
return ret
|
||||
elif self.sep_style == SeparatorStyle.FALCON_CHAT:
|
||||
ret = ''
|
||||
if self.system_message:
|
||||
ret += system_prompt + self.sep
|
||||
for role, message in self.messages:
|
||||
if message:
|
||||
ret += role + ': ' + message + self.sep
|
||||
else:
|
||||
ret += role + ':'
|
||||
|
||||
return ret
|
||||
elif self.sep_style == SeparatorStyle.INTERNVL_ZH:
|
||||
seps = [self.sep, self.sep2]
|
||||
ret = self.system_message + seps[0]
|
||||
for i, (role, message) in enumerate(self.messages):
|
||||
if message:
|
||||
ret += role + ': ' + message + seps[i % 2]
|
||||
else:
|
||||
ret += role + ':'
|
||||
return ret
|
||||
elif self.sep_style == SeparatorStyle.MPT:
|
||||
ret = system_prompt + self.sep
|
||||
for role, message in self.messages:
|
||||
if message:
|
||||
if type(message) is tuple:
|
||||
message, _, _ = message
|
||||
ret += role + message + self.sep
|
||||
else:
|
||||
ret += role
|
||||
return ret
|
||||
else:
|
||||
raise ValueError(f'Invalid style: {self.sep_style}')
|
||||
|
||||
def set_system_message(self, system_message: str):
|
||||
"""Set the system message."""
|
||||
self.system_message = system_message
|
||||
|
||||
def append_message(self, role: str, message: str):
|
||||
"""Append a new message."""
|
||||
self.messages.append([role, message])
|
||||
|
||||
def update_last_message(self, message: str):
|
||||
"""Update the last output.
|
||||
|
||||
The last message is typically set to be None when constructing the prompt,
|
||||
so we need to update it in-place after getting the response from a model.
|
||||
"""
|
||||
self.messages[-1][1] = message
|
||||
|
||||
def to_gradio_chatbot(self):
|
||||
"""Convert the conversation to gradio chatbot format."""
|
||||
ret = []
|
||||
for i, (role, msg) in enumerate(self.messages[self.offset :]):
|
||||
if i % 2 == 0:
|
||||
ret.append([msg, None])
|
||||
else:
|
||||
ret[-1][-1] = msg
|
||||
return ret
|
||||
|
||||
def to_openai_api_messages(self):
|
||||
"""Convert the conversation to OpenAI chat completion format."""
|
||||
ret = [{'role': 'system', 'content': self.system_message}]
|
||||
|
||||
for i, (_, msg) in enumerate(self.messages[self.offset :]):
|
||||
if i % 2 == 0:
|
||||
ret.append({'role': 'user', 'content': msg})
|
||||
else:
|
||||
if msg is not None:
|
||||
ret.append({'role': 'assistant', 'content': msg})
|
||||
return ret
|
||||
|
||||
def copy(self):
|
||||
return Conversation(
|
||||
name=self.name,
|
||||
system_template=self.system_template,
|
||||
system_message=self.system_message,
|
||||
roles=self.roles,
|
||||
messages=[[x, y] for x, y in self.messages],
|
||||
offset=self.offset,
|
||||
sep_style=self.sep_style,
|
||||
sep=self.sep,
|
||||
sep2=self.sep2,
|
||||
stop_str=self.stop_str,
|
||||
stop_token_ids=self.stop_token_ids,
|
||||
)
|
||||
|
||||
def dict(self):
|
||||
return {
|
||||
'template_name': self.name,
|
||||
'system_message': self.system_message,
|
||||
'roles': self.roles,
|
||||
'messages': self.messages,
|
||||
'offset': self.offset,
|
||||
}
|
||||
|
||||
|
||||
# A global registry for all conversation templates
|
||||
conv_templates: Dict[str, Conversation] = {}
|
||||
|
||||
|
||||
def register_conv_template(template: Conversation, override: bool = False):
|
||||
"""Register a new conversation template."""
|
||||
if not override:
|
||||
assert (
|
||||
template.name not in conv_templates
|
||||
), f'{template.name} has been registered.'
|
||||
|
||||
conv_templates[template.name] = template
|
||||
|
||||
|
||||
def get_conv_template(name: str) -> Conversation:
|
||||
"""Get a conversation template."""
|
||||
return conv_templates[name].copy()
|
||||
|
||||
|
||||
# Both Hermes-2 and internlm2-chat are chatml-format conversation templates. The difference
|
||||
# is that during training, the preprocessing function for the Hermes-2 template doesn't add
|
||||
# <s> at the beginning of the tokenized sequence, while the internlm2-chat template does.
|
||||
# Therefore, they are completely equivalent during inference.
|
||||
register_conv_template(
|
||||
Conversation(
|
||||
name='Hermes-2',
|
||||
system_template='<|im_start|>system\n{system_message}',
|
||||
# note: The new system prompt was not used here to avoid changes in benchmark performance.
|
||||
# system_message='我是书生·万象,英文名是InternVL,是由上海人工智能实验室、清华大学及多家合作单位联合开发的多模态大语言模型。',
|
||||
system_message='你是由上海人工智能实验室联合商汤科技开发的书生多模态大模型,英文名叫InternVL, 是一个有用无害的人工智能助手。',
|
||||
roles=('<|im_start|>user\n', '<|im_start|>assistant\n'),
|
||||
sep_style=SeparatorStyle.MPT,
|
||||
sep='<|im_end|>',
|
||||
stop_str='<|endoftext|>',
|
||||
)
|
||||
)
|
||||
|
||||
|
||||
register_conv_template(
|
||||
Conversation(
|
||||
name='internlm2-chat',
|
||||
system_template='<|im_start|>system\n{system_message}',
|
||||
# note: The new system prompt was not used here to avoid changes in benchmark performance.
|
||||
# system_message='我是书生·万象,英文名是InternVL,是由上海人工智能实验室、清华大学及多家合作单位联合开发的多模态大语言模型。',
|
||||
system_message='你是由上海人工智能实验室联合商汤科技开发的书生多模态大模型,英文名叫InternVL, 是一个有用无害的人工智能助手。',
|
||||
roles=('<|im_start|>user\n', '<|im_start|>assistant\n'),
|
||||
sep_style=SeparatorStyle.MPT,
|
||||
sep='<|im_end|>',
|
||||
)
|
||||
)
|
||||
|
||||
|
||||
register_conv_template(
|
||||
Conversation(
|
||||
name='phi3-chat',
|
||||
system_template='<|system|>\n{system_message}',
|
||||
# note: The new system prompt was not used here to avoid changes in benchmark performance.
|
||||
# system_message='我是书生·万象,英文名是InternVL,是由上海人工智能实验室、清华大学及多家合作单位联合开发的多模态大语言模型。',
|
||||
system_message='你是由上海人工智能实验室联合商汤科技开发的书生多模态大模型,英文名叫InternVL, 是一个有用无害的人工智能助手。',
|
||||
roles=('<|user|>\n', '<|assistant|>\n'),
|
||||
sep_style=SeparatorStyle.MPT,
|
||||
sep='<|end|>',
|
||||
)
|
||||
)
|
||||
|
||||
|
||||
register_conv_template(
|
||||
Conversation(
|
||||
name='internvl2_5',
|
||||
system_template='<|im_start|>system\n{system_message}',
|
||||
system_message='你是书生·万象,英文名是InternVL,是由上海人工智能实验室、清华大学及多家合作单位联合开发的多模态大语言模型。',
|
||||
roles=('<|im_start|>user\n', '<|im_start|>assistant\n'),
|
||||
sep_style=SeparatorStyle.MPT,
|
||||
sep='<|im_end|>\n',
|
||||
)
|
||||
)
|
File diff suppressed because one or more lines are too long
Binary file not shown.
After Width: | Height: | Size: 76 KiB |
Binary file not shown.
After Width: | Height: | Size: 123 KiB |
Binary file not shown.
|
@ -0,0 +1,8 @@
|
|||
{
|
||||
"_from_model_config": true,
|
||||
"transformers_version": "4.37.2",
|
||||
"eos_token_id": [
|
||||
92542,
|
||||
92543
|
||||
]
|
||||
}
|
Binary file not shown.
Binary file not shown.
Binary file not shown.
Binary file not shown.
|
@ -0,0 +1,580 @@
|
|||
{
|
||||
"metadata": {
|
||||
"total_size": 16150730752
|
||||
},
|
||||
"weight_map": {
|
||||
"language_model.model.layers.0.attention.wo.weight": "model-00001-of-00004.safetensors",
|
||||
"language_model.model.layers.0.attention.wqkv.weight": "model-00001-of-00004.safetensors",
|
||||
"language_model.model.layers.0.attention_norm.weight": "model-00001-of-00004.safetensors",
|
||||
"language_model.model.layers.0.feed_forward.w1.weight": "model-00001-of-00004.safetensors",
|
||||
"language_model.model.layers.0.feed_forward.w2.weight": "model-00001-of-00004.safetensors",
|
||||
"language_model.model.layers.0.feed_forward.w3.weight": "model-00001-of-00004.safetensors",
|
||||
"language_model.model.layers.0.ffn_norm.weight": "model-00001-of-00004.safetensors",
|
||||
"language_model.model.layers.1.attention.wo.weight": "model-00001-of-00004.safetensors",
|
||||
"language_model.model.layers.1.attention.wqkv.weight": "model-00001-of-00004.safetensors",
|
||||
"language_model.model.layers.1.attention_norm.weight": "model-00001-of-00004.safetensors",
|
||||
"language_model.model.layers.1.feed_forward.w1.weight": "model-00001-of-00004.safetensors",
|
||||
"language_model.model.layers.1.feed_forward.w2.weight": "model-00001-of-00004.safetensors",
|
||||
"language_model.model.layers.1.feed_forward.w3.weight": "model-00001-of-00004.safetensors",
|
||||
"language_model.model.layers.1.ffn_norm.weight": "model-00001-of-00004.safetensors",
|
||||
"language_model.model.layers.10.attention.wo.weight": "model-00002-of-00004.safetensors",
|
||||
"language_model.model.layers.10.attention.wqkv.weight": "model-00002-of-00004.safetensors",
|
||||
"language_model.model.layers.10.attention_norm.weight": "model-00002-of-00004.safetensors",
|
||||
"language_model.model.layers.10.feed_forward.w1.weight": "model-00002-of-00004.safetensors",
|
||||
"language_model.model.layers.10.feed_forward.w2.weight": "model-00002-of-00004.safetensors",
|
||||
"language_model.model.layers.10.feed_forward.w3.weight": "model-00002-of-00004.safetensors",
|
||||
"language_model.model.layers.10.ffn_norm.weight": "model-00002-of-00004.safetensors",
|
||||
"language_model.model.layers.11.attention.wo.weight": "model-00002-of-00004.safetensors",
|
||||
"language_model.model.layers.11.attention.wqkv.weight": "model-00002-of-00004.safetensors",
|
||||
"language_model.model.layers.11.attention_norm.weight": "model-00002-of-00004.safetensors",
|
||||
"language_model.model.layers.11.feed_forward.w1.weight": "model-00002-of-00004.safetensors",
|
||||
"language_model.model.layers.11.feed_forward.w2.weight": "model-00002-of-00004.safetensors",
|
||||
"language_model.model.layers.11.feed_forward.w3.weight": "model-00002-of-00004.safetensors",
|
||||
"language_model.model.layers.11.ffn_norm.weight": "model-00002-of-00004.safetensors",
|
||||
"language_model.model.layers.12.attention.wo.weight": "model-00002-of-00004.safetensors",
|
||||
"language_model.model.layers.12.attention.wqkv.weight": "model-00002-of-00004.safetensors",
|
||||
"language_model.model.layers.12.attention_norm.weight": "model-00002-of-00004.safetensors",
|
||||
"language_model.model.layers.12.feed_forward.w1.weight": "model-00002-of-00004.safetensors",
|
||||
"language_model.model.layers.12.feed_forward.w2.weight": "model-00002-of-00004.safetensors",
|
||||
"language_model.model.layers.12.feed_forward.w3.weight": "model-00002-of-00004.safetensors",
|
||||
"language_model.model.layers.12.ffn_norm.weight": "model-00002-of-00004.safetensors",
|
||||
"language_model.model.layers.13.attention.wo.weight": "model-00002-of-00004.safetensors",
|
||||
"language_model.model.layers.13.attention.wqkv.weight": "model-00002-of-00004.safetensors",
|
||||
"language_model.model.layers.13.attention_norm.weight": "model-00002-of-00004.safetensors",
|
||||
"language_model.model.layers.13.feed_forward.w1.weight": "model-00002-of-00004.safetensors",
|
||||
"language_model.model.layers.13.feed_forward.w2.weight": "model-00002-of-00004.safetensors",
|
||||
"language_model.model.layers.13.feed_forward.w3.weight": "model-00002-of-00004.safetensors",
|
||||
"language_model.model.layers.13.ffn_norm.weight": "model-00002-of-00004.safetensors",
|
||||
"language_model.model.layers.14.attention.wo.weight": "model-00002-of-00004.safetensors",
|
||||
"language_model.model.layers.14.attention.wqkv.weight": "model-00002-of-00004.safetensors",
|
||||
"language_model.model.layers.14.attention_norm.weight": "model-00002-of-00004.safetensors",
|
||||
"language_model.model.layers.14.feed_forward.w1.weight": "model-00002-of-00004.safetensors",
|
||||
"language_model.model.layers.14.feed_forward.w2.weight": "model-00002-of-00004.safetensors",
|
||||
"language_model.model.layers.14.feed_forward.w3.weight": "model-00002-of-00004.safetensors",
|
||||
"language_model.model.layers.14.ffn_norm.weight": "model-00002-of-00004.safetensors",
|
||||
"language_model.model.layers.15.attention.wo.weight": "model-00002-of-00004.safetensors",
|
||||
"language_model.model.layers.15.attention.wqkv.weight": "model-00002-of-00004.safetensors",
|
||||
"language_model.model.layers.15.attention_norm.weight": "model-00002-of-00004.safetensors",
|
||||
"language_model.model.layers.15.feed_forward.w1.weight": "model-00002-of-00004.safetensors",
|
||||
"language_model.model.layers.15.feed_forward.w2.weight": "model-00002-of-00004.safetensors",
|
||||
"language_model.model.layers.15.feed_forward.w3.weight": "model-00002-of-00004.safetensors",
|
||||
"language_model.model.layers.15.ffn_norm.weight": "model-00002-of-00004.safetensors",
|
||||
"language_model.model.layers.16.attention.wo.weight": "model-00002-of-00004.safetensors",
|
||||
"language_model.model.layers.16.attention.wqkv.weight": "model-00002-of-00004.safetensors",
|
||||
"language_model.model.layers.16.attention_norm.weight": "model-00002-of-00004.safetensors",
|
||||
"language_model.model.layers.16.feed_forward.w1.weight": "model-00002-of-00004.safetensors",
|
||||
"language_model.model.layers.16.feed_forward.w2.weight": "model-00002-of-00004.safetensors",
|
||||
"language_model.model.layers.16.feed_forward.w3.weight": "model-00002-of-00004.safetensors",
|
||||
"language_model.model.layers.16.ffn_norm.weight": "model-00002-of-00004.safetensors",
|
||||
"language_model.model.layers.17.attention.wo.weight": "model-00002-of-00004.safetensors",
|
||||
"language_model.model.layers.17.attention.wqkv.weight": "model-00002-of-00004.safetensors",
|
||||
"language_model.model.layers.17.attention_norm.weight": "model-00002-of-00004.safetensors",
|
||||
"language_model.model.layers.17.feed_forward.w1.weight": "model-00002-of-00004.safetensors",
|
||||
"language_model.model.layers.17.feed_forward.w2.weight": "model-00002-of-00004.safetensors",
|
||||
"language_model.model.layers.17.feed_forward.w3.weight": "model-00002-of-00004.safetensors",
|
||||
"language_model.model.layers.17.ffn_norm.weight": "model-00002-of-00004.safetensors",
|
||||
"language_model.model.layers.18.attention.wo.weight": "model-00002-of-00004.safetensors",
|
||||
"language_model.model.layers.18.attention.wqkv.weight": "model-00002-of-00004.safetensors",
|
||||
"language_model.model.layers.18.attention_norm.weight": "model-00002-of-00004.safetensors",
|
||||
"language_model.model.layers.18.feed_forward.w1.weight": "model-00002-of-00004.safetensors",
|
||||
"language_model.model.layers.18.feed_forward.w2.weight": "model-00002-of-00004.safetensors",
|
||||
"language_model.model.layers.18.feed_forward.w3.weight": "model-00002-of-00004.safetensors",
|
||||
"language_model.model.layers.18.ffn_norm.weight": "model-00002-of-00004.safetensors",
|
||||
"language_model.model.layers.19.attention.wo.weight": "model-00002-of-00004.safetensors",
|
||||
"language_model.model.layers.19.attention.wqkv.weight": "model-00002-of-00004.safetensors",
|
||||
"language_model.model.layers.19.attention_norm.weight": "model-00003-of-00004.safetensors",
|
||||
"language_model.model.layers.19.feed_forward.w1.weight": "model-00002-of-00004.safetensors",
|
||||
"language_model.model.layers.19.feed_forward.w2.weight": "model-00003-of-00004.safetensors",
|
||||
"language_model.model.layers.19.feed_forward.w3.weight": "model-00003-of-00004.safetensors",
|
||||
"language_model.model.layers.19.ffn_norm.weight": "model-00003-of-00004.safetensors",
|
||||
"language_model.model.layers.2.attention.wo.weight": "model-00001-of-00004.safetensors",
|
||||
"language_model.model.layers.2.attention.wqkv.weight": "model-00001-of-00004.safetensors",
|
||||
"language_model.model.layers.2.attention_norm.weight": "model-00001-of-00004.safetensors",
|
||||
"language_model.model.layers.2.feed_forward.w1.weight": "model-00001-of-00004.safetensors",
|
||||
"language_model.model.layers.2.feed_forward.w2.weight": "model-00001-of-00004.safetensors",
|
||||
"language_model.model.layers.2.feed_forward.w3.weight": "model-00001-of-00004.safetensors",
|
||||
"language_model.model.layers.2.ffn_norm.weight": "model-00001-of-00004.safetensors",
|
||||
"language_model.model.layers.20.attention.wo.weight": "model-00003-of-00004.safetensors",
|
||||
"language_model.model.layers.20.attention.wqkv.weight": "model-00003-of-00004.safetensors",
|
||||
"language_model.model.layers.20.attention_norm.weight": "model-00003-of-00004.safetensors",
|
||||
"language_model.model.layers.20.feed_forward.w1.weight": "model-00003-of-00004.safetensors",
|
||||
"language_model.model.layers.20.feed_forward.w2.weight": "model-00003-of-00004.safetensors",
|
||||
"language_model.model.layers.20.feed_forward.w3.weight": "model-00003-of-00004.safetensors",
|
||||
"language_model.model.layers.20.ffn_norm.weight": "model-00003-of-00004.safetensors",
|
||||
"language_model.model.layers.21.attention.wo.weight": "model-00003-of-00004.safetensors",
|
||||
"language_model.model.layers.21.attention.wqkv.weight": "model-00003-of-00004.safetensors",
|
||||
"language_model.model.layers.21.attention_norm.weight": "model-00003-of-00004.safetensors",
|
||||
"language_model.model.layers.21.feed_forward.w1.weight": "model-00003-of-00004.safetensors",
|
||||
"language_model.model.layers.21.feed_forward.w2.weight": "model-00003-of-00004.safetensors",
|
||||
"language_model.model.layers.21.feed_forward.w3.weight": "model-00003-of-00004.safetensors",
|
||||
"language_model.model.layers.21.ffn_norm.weight": "model-00003-of-00004.safetensors",
|
||||
"language_model.model.layers.22.attention.wo.weight": "model-00003-of-00004.safetensors",
|
||||
"language_model.model.layers.22.attention.wqkv.weight": "model-00003-of-00004.safetensors",
|
||||
"language_model.model.layers.22.attention_norm.weight": "model-00003-of-00004.safetensors",
|
||||
"language_model.model.layers.22.feed_forward.w1.weight": "model-00003-of-00004.safetensors",
|
||||
"language_model.model.layers.22.feed_forward.w2.weight": "model-00003-of-00004.safetensors",
|
||||
"language_model.model.layers.22.feed_forward.w3.weight": "model-00003-of-00004.safetensors",
|
||||
"language_model.model.layers.22.ffn_norm.weight": "model-00003-of-00004.safetensors",
|
||||
"language_model.model.layers.23.attention.wo.weight": "model-00003-of-00004.safetensors",
|
||||
"language_model.model.layers.23.attention.wqkv.weight": "model-00003-of-00004.safetensors",
|
||||
"language_model.model.layers.23.attention_norm.weight": "model-00003-of-00004.safetensors",
|
||||
"language_model.model.layers.23.feed_forward.w1.weight": "model-00003-of-00004.safetensors",
|
||||
"language_model.model.layers.23.feed_forward.w2.weight": "model-00003-of-00004.safetensors",
|
||||
"language_model.model.layers.23.feed_forward.w3.weight": "model-00003-of-00004.safetensors",
|
||||
"language_model.model.layers.23.ffn_norm.weight": "model-00003-of-00004.safetensors",
|
||||
"language_model.model.layers.24.attention.wo.weight": "model-00003-of-00004.safetensors",
|
||||
"language_model.model.layers.24.attention.wqkv.weight": "model-00003-of-00004.safetensors",
|
||||
"language_model.model.layers.24.attention_norm.weight": "model-00003-of-00004.safetensors",
|
||||
"language_model.model.layers.24.feed_forward.w1.weight": "model-00003-of-00004.safetensors",
|
||||
"language_model.model.layers.24.feed_forward.w2.weight": "model-00003-of-00004.safetensors",
|
||||
"language_model.model.layers.24.feed_forward.w3.weight": "model-00003-of-00004.safetensors",
|
||||
"language_model.model.layers.24.ffn_norm.weight": "model-00003-of-00004.safetensors",
|
||||
"language_model.model.layers.25.attention.wo.weight": "model-00003-of-00004.safetensors",
|
||||
"language_model.model.layers.25.attention.wqkv.weight": "model-00003-of-00004.safetensors",
|
||||
"language_model.model.layers.25.attention_norm.weight": "model-00003-of-00004.safetensors",
|
||||
"language_model.model.layers.25.feed_forward.w1.weight": "model-00003-of-00004.safetensors",
|
||||
"language_model.model.layers.25.feed_forward.w2.weight": "model-00003-of-00004.safetensors",
|
||||
"language_model.model.layers.25.feed_forward.w3.weight": "model-00003-of-00004.safetensors",
|
||||
"language_model.model.layers.25.ffn_norm.weight": "model-00003-of-00004.safetensors",
|
||||
"language_model.model.layers.26.attention.wo.weight": "model-00003-of-00004.safetensors",
|
||||
"language_model.model.layers.26.attention.wqkv.weight": "model-00003-of-00004.safetensors",
|
||||
"language_model.model.layers.26.attention_norm.weight": "model-00003-of-00004.safetensors",
|
||||
"language_model.model.layers.26.feed_forward.w1.weight": "model-00003-of-00004.safetensors",
|
||||
"language_model.model.layers.26.feed_forward.w2.weight": "model-00003-of-00004.safetensors",
|
||||
"language_model.model.layers.26.feed_forward.w3.weight": "model-00003-of-00004.safetensors",
|
||||
"language_model.model.layers.26.ffn_norm.weight": "model-00003-of-00004.safetensors",
|
||||
"language_model.model.layers.27.attention.wo.weight": "model-00003-of-00004.safetensors",
|
||||
"language_model.model.layers.27.attention.wqkv.weight": "model-00003-of-00004.safetensors",
|
||||
"language_model.model.layers.27.attention_norm.weight": "model-00003-of-00004.safetensors",
|
||||
"language_model.model.layers.27.feed_forward.w1.weight": "model-00003-of-00004.safetensors",
|
||||
"language_model.model.layers.27.feed_forward.w2.weight": "model-00003-of-00004.safetensors",
|
||||
"language_model.model.layers.27.feed_forward.w3.weight": "model-00003-of-00004.safetensors",
|
||||
"language_model.model.layers.27.ffn_norm.weight": "model-00003-of-00004.safetensors",
|
||||
"language_model.model.layers.28.attention.wo.weight": "model-00003-of-00004.safetensors",
|
||||
"language_model.model.layers.28.attention.wqkv.weight": "model-00003-of-00004.safetensors",
|
||||
"language_model.model.layers.28.attention_norm.weight": "model-00003-of-00004.safetensors",
|
||||
"language_model.model.layers.28.feed_forward.w1.weight": "model-00003-of-00004.safetensors",
|
||||
"language_model.model.layers.28.feed_forward.w2.weight": "model-00003-of-00004.safetensors",
|
||||
"language_model.model.layers.28.feed_forward.w3.weight": "model-00003-of-00004.safetensors",
|
||||
"language_model.model.layers.28.ffn_norm.weight": "model-00003-of-00004.safetensors",
|
||||
"language_model.model.layers.29.attention.wo.weight": "model-00003-of-00004.safetensors",
|
||||
"language_model.model.layers.29.attention.wqkv.weight": "model-00003-of-00004.safetensors",
|
||||
"language_model.model.layers.29.attention_norm.weight": "model-00003-of-00004.safetensors",
|
||||
"language_model.model.layers.29.feed_forward.w1.weight": "model-00003-of-00004.safetensors",
|
||||
"language_model.model.layers.29.feed_forward.w2.weight": "model-00003-of-00004.safetensors",
|
||||
"language_model.model.layers.29.feed_forward.w3.weight": "model-00003-of-00004.safetensors",
|
||||
"language_model.model.layers.29.ffn_norm.weight": "model-00003-of-00004.safetensors",
|
||||
"language_model.model.layers.3.attention.wo.weight": "model-00001-of-00004.safetensors",
|
||||
"language_model.model.layers.3.attention.wqkv.weight": "model-00001-of-00004.safetensors",
|
||||
"language_model.model.layers.3.attention_norm.weight": "model-00001-of-00004.safetensors",
|
||||
"language_model.model.layers.3.feed_forward.w1.weight": "model-00001-of-00004.safetensors",
|
||||
"language_model.model.layers.3.feed_forward.w2.weight": "model-00001-of-00004.safetensors",
|
||||
"language_model.model.layers.3.feed_forward.w3.weight": "model-00001-of-00004.safetensors",
|
||||
"language_model.model.layers.3.ffn_norm.weight": "model-00001-of-00004.safetensors",
|
||||
"language_model.model.layers.30.attention.wo.weight": "model-00003-of-00004.safetensors",
|
||||
"language_model.model.layers.30.attention.wqkv.weight": "model-00003-of-00004.safetensors",
|
||||
"language_model.model.layers.30.attention_norm.weight": "model-00004-of-00004.safetensors",
|
||||
"language_model.model.layers.30.feed_forward.w1.weight": "model-00003-of-00004.safetensors",
|
||||
"language_model.model.layers.30.feed_forward.w2.weight": "model-00004-of-00004.safetensors",
|
||||
"language_model.model.layers.30.feed_forward.w3.weight": "model-00003-of-00004.safetensors",
|
||||
"language_model.model.layers.30.ffn_norm.weight": "model-00004-of-00004.safetensors",
|
||||
"language_model.model.layers.31.attention.wo.weight": "model-00004-of-00004.safetensors",
|
||||
"language_model.model.layers.31.attention.wqkv.weight": "model-00004-of-00004.safetensors",
|
||||
"language_model.model.layers.31.attention_norm.weight": "model-00004-of-00004.safetensors",
|
||||
"language_model.model.layers.31.feed_forward.w1.weight": "model-00004-of-00004.safetensors",
|
||||
"language_model.model.layers.31.feed_forward.w2.weight": "model-00004-of-00004.safetensors",
|
||||
"language_model.model.layers.31.feed_forward.w3.weight": "model-00004-of-00004.safetensors",
|
||||
"language_model.model.layers.31.ffn_norm.weight": "model-00004-of-00004.safetensors",
|
||||
"language_model.model.layers.4.attention.wo.weight": "model-00001-of-00004.safetensors",
|
||||
"language_model.model.layers.4.attention.wqkv.weight": "model-00001-of-00004.safetensors",
|
||||
"language_model.model.layers.4.attention_norm.weight": "model-00001-of-00004.safetensors",
|
||||
"language_model.model.layers.4.feed_forward.w1.weight": "model-00001-of-00004.safetensors",
|
||||
"language_model.model.layers.4.feed_forward.w2.weight": "model-00001-of-00004.safetensors",
|
||||
"language_model.model.layers.4.feed_forward.w3.weight": "model-00001-of-00004.safetensors",
|
||||
"language_model.model.layers.4.ffn_norm.weight": "model-00001-of-00004.safetensors",
|
||||
"language_model.model.layers.5.attention.wo.weight": "model-00001-of-00004.safetensors",
|
||||
"language_model.model.layers.5.attention.wqkv.weight": "model-00001-of-00004.safetensors",
|
||||
"language_model.model.layers.5.attention_norm.weight": "model-00001-of-00004.safetensors",
|
||||
"language_model.model.layers.5.feed_forward.w1.weight": "model-00001-of-00004.safetensors",
|
||||
"language_model.model.layers.5.feed_forward.w2.weight": "model-00001-of-00004.safetensors",
|
||||
"language_model.model.layers.5.feed_forward.w3.weight": "model-00001-of-00004.safetensors",
|
||||
"language_model.model.layers.5.ffn_norm.weight": "model-00001-of-00004.safetensors",
|
||||
"language_model.model.layers.6.attention.wo.weight": "model-00001-of-00004.safetensors",
|
||||
"language_model.model.layers.6.attention.wqkv.weight": "model-00001-of-00004.safetensors",
|
||||
"language_model.model.layers.6.attention_norm.weight": "model-00001-of-00004.safetensors",
|
||||
"language_model.model.layers.6.feed_forward.w1.weight": "model-00001-of-00004.safetensors",
|
||||
"language_model.model.layers.6.feed_forward.w2.weight": "model-00001-of-00004.safetensors",
|
||||
"language_model.model.layers.6.feed_forward.w3.weight": "model-00001-of-00004.safetensors",
|
||||
"language_model.model.layers.6.ffn_norm.weight": "model-00001-of-00004.safetensors",
|
||||
"language_model.model.layers.7.attention.wo.weight": "model-00001-of-00004.safetensors",
|
||||
"language_model.model.layers.7.attention.wqkv.weight": "model-00001-of-00004.safetensors",
|
||||
"language_model.model.layers.7.attention_norm.weight": "model-00001-of-00004.safetensors",
|
||||
"language_model.model.layers.7.feed_forward.w1.weight": "model-00001-of-00004.safetensors",
|
||||
"language_model.model.layers.7.feed_forward.w2.weight": "model-00001-of-00004.safetensors",
|
||||
"language_model.model.layers.7.feed_forward.w3.weight": "model-00001-of-00004.safetensors",
|
||||
"language_model.model.layers.7.ffn_norm.weight": "model-00001-of-00004.safetensors",
|
||||
"language_model.model.layers.8.attention.wo.weight": "model-00001-of-00004.safetensors",
|
||||
"language_model.model.layers.8.attention.wqkv.weight": "model-00001-of-00004.safetensors",
|
||||
"language_model.model.layers.8.attention_norm.weight": "model-00002-of-00004.safetensors",
|
||||
"language_model.model.layers.8.feed_forward.w1.weight": "model-00002-of-00004.safetensors",
|
||||
"language_model.model.layers.8.feed_forward.w2.weight": "model-00002-of-00004.safetensors",
|
||||
"language_model.model.layers.8.feed_forward.w3.weight": "model-00002-of-00004.safetensors",
|
||||
"language_model.model.layers.8.ffn_norm.weight": "model-00002-of-00004.safetensors",
|
||||
"language_model.model.layers.9.attention.wo.weight": "model-00002-of-00004.safetensors",
|
||||
"language_model.model.layers.9.attention.wqkv.weight": "model-00002-of-00004.safetensors",
|
||||
"language_model.model.layers.9.attention_norm.weight": "model-00002-of-00004.safetensors",
|
||||
"language_model.model.layers.9.feed_forward.w1.weight": "model-00002-of-00004.safetensors",
|
||||
"language_model.model.layers.9.feed_forward.w2.weight": "model-00002-of-00004.safetensors",
|
||||
"language_model.model.layers.9.feed_forward.w3.weight": "model-00002-of-00004.safetensors",
|
||||
"language_model.model.layers.9.ffn_norm.weight": "model-00002-of-00004.safetensors",
|
||||
"language_model.model.norm.weight": "model-00004-of-00004.safetensors",
|
||||
"language_model.model.tok_embeddings.weight": "model-00001-of-00004.safetensors",
|
||||
"language_model.output.weight": "model-00004-of-00004.safetensors",
|
||||
"mlp1.0.bias": "model-00004-of-00004.safetensors",
|
||||
"mlp1.0.weight": "model-00004-of-00004.safetensors",
|
||||
"mlp1.1.bias": "model-00004-of-00004.safetensors",
|
||||
"mlp1.1.weight": "model-00004-of-00004.safetensors",
|
||||
"mlp1.3.bias": "model-00004-of-00004.safetensors",
|
||||
"mlp1.3.weight": "model-00004-of-00004.safetensors",
|
||||
"vision_model.embeddings.class_embedding": "model-00001-of-00004.safetensors",
|
||||
"vision_model.embeddings.patch_embedding.bias": "model-00001-of-00004.safetensors",
|
||||
"vision_model.embeddings.patch_embedding.weight": "model-00001-of-00004.safetensors",
|
||||
"vision_model.embeddings.position_embedding": "model-00001-of-00004.safetensors",
|
||||
"vision_model.encoder.layers.0.attn.proj.bias": "model-00001-of-00004.safetensors",
|
||||
"vision_model.encoder.layers.0.attn.proj.weight": "model-00001-of-00004.safetensors",
|
||||
"vision_model.encoder.layers.0.attn.qkv.bias": "model-00001-of-00004.safetensors",
|
||||
"vision_model.encoder.layers.0.attn.qkv.weight": "model-00001-of-00004.safetensors",
|
||||
"vision_model.encoder.layers.0.ls1": "model-00001-of-00004.safetensors",
|
||||
"vision_model.encoder.layers.0.ls2": "model-00001-of-00004.safetensors",
|
||||
"vision_model.encoder.layers.0.mlp.fc1.bias": "model-00001-of-00004.safetensors",
|
||||
"vision_model.encoder.layers.0.mlp.fc1.weight": "model-00001-of-00004.safetensors",
|
||||
"vision_model.encoder.layers.0.mlp.fc2.bias": "model-00001-of-00004.safetensors",
|
||||
"vision_model.encoder.layers.0.mlp.fc2.weight": "model-00001-of-00004.safetensors",
|
||||
"vision_model.encoder.layers.0.norm1.bias": "model-00001-of-00004.safetensors",
|
||||
"vision_model.encoder.layers.0.norm1.weight": "model-00001-of-00004.safetensors",
|
||||
"vision_model.encoder.layers.0.norm2.bias": "model-00001-of-00004.safetensors",
|
||||
"vision_model.encoder.layers.0.norm2.weight": "model-00001-of-00004.safetensors",
|
||||
"vision_model.encoder.layers.1.attn.proj.bias": "model-00001-of-00004.safetensors",
|
||||
"vision_model.encoder.layers.1.attn.proj.weight": "model-00001-of-00004.safetensors",
|
||||
"vision_model.encoder.layers.1.attn.qkv.bias": "model-00001-of-00004.safetensors",
|
||||
"vision_model.encoder.layers.1.attn.qkv.weight": "model-00001-of-00004.safetensors",
|
||||
"vision_model.encoder.layers.1.ls1": "model-00001-of-00004.safetensors",
|
||||
"vision_model.encoder.layers.1.ls2": "model-00001-of-00004.safetensors",
|
||||
"vision_model.encoder.layers.1.mlp.fc1.bias": "model-00001-of-00004.safetensors",
|
||||
"vision_model.encoder.layers.1.mlp.fc1.weight": "model-00001-of-00004.safetensors",
|
||||
"vision_model.encoder.layers.1.mlp.fc2.bias": "model-00001-of-00004.safetensors",
|
||||
"vision_model.encoder.layers.1.mlp.fc2.weight": "model-00001-of-00004.safetensors",
|
||||
"vision_model.encoder.layers.1.norm1.bias": "model-00001-of-00004.safetensors",
|
||||
"vision_model.encoder.layers.1.norm1.weight": "model-00001-of-00004.safetensors",
|
||||
"vision_model.encoder.layers.1.norm2.bias": "model-00001-of-00004.safetensors",
|
||||
"vision_model.encoder.layers.1.norm2.weight": "model-00001-of-00004.safetensors",
|
||||
"vision_model.encoder.layers.10.attn.proj.bias": "model-00001-of-00004.safetensors",
|
||||
"vision_model.encoder.layers.10.attn.proj.weight": "model-00001-of-00004.safetensors",
|
||||
"vision_model.encoder.layers.10.attn.qkv.bias": "model-00001-of-00004.safetensors",
|
||||
"vision_model.encoder.layers.10.attn.qkv.weight": "model-00001-of-00004.safetensors",
|
||||
"vision_model.encoder.layers.10.ls1": "model-00001-of-00004.safetensors",
|
||||
"vision_model.encoder.layers.10.ls2": "model-00001-of-00004.safetensors",
|
||||
"vision_model.encoder.layers.10.mlp.fc1.bias": "model-00001-of-00004.safetensors",
|
||||
"vision_model.encoder.layers.10.mlp.fc1.weight": "model-00001-of-00004.safetensors",
|
||||
"vision_model.encoder.layers.10.mlp.fc2.bias": "model-00001-of-00004.safetensors",
|
||||
"vision_model.encoder.layers.10.mlp.fc2.weight": "model-00001-of-00004.safetensors",
|
||||
"vision_model.encoder.layers.10.norm1.bias": "model-00001-of-00004.safetensors",
|
||||
"vision_model.encoder.layers.10.norm1.weight": "model-00001-of-00004.safetensors",
|
||||
"vision_model.encoder.layers.10.norm2.bias": "model-00001-of-00004.safetensors",
|
||||
"vision_model.encoder.layers.10.norm2.weight": "model-00001-of-00004.safetensors",
|
||||
"vision_model.encoder.layers.11.attn.proj.bias": "model-00001-of-00004.safetensors",
|
||||
"vision_model.encoder.layers.11.attn.proj.weight": "model-00001-of-00004.safetensors",
|
||||
"vision_model.encoder.layers.11.attn.qkv.bias": "model-00001-of-00004.safetensors",
|
||||
"vision_model.encoder.layers.11.attn.qkv.weight": "model-00001-of-00004.safetensors",
|
||||
"vision_model.encoder.layers.11.ls1": "model-00001-of-00004.safetensors",
|
||||
"vision_model.encoder.layers.11.ls2": "model-00001-of-00004.safetensors",
|
||||
"vision_model.encoder.layers.11.mlp.fc1.bias": "model-00001-of-00004.safetensors",
|
||||
"vision_model.encoder.layers.11.mlp.fc1.weight": "model-00001-of-00004.safetensors",
|
||||
"vision_model.encoder.layers.11.mlp.fc2.bias": "model-00001-of-00004.safetensors",
|
||||
"vision_model.encoder.layers.11.mlp.fc2.weight": "model-00001-of-00004.safetensors",
|
||||
"vision_model.encoder.layers.11.norm1.bias": "model-00001-of-00004.safetensors",
|
||||
"vision_model.encoder.layers.11.norm1.weight": "model-00001-of-00004.safetensors",
|
||||
"vision_model.encoder.layers.11.norm2.bias": "model-00001-of-00004.safetensors",
|
||||
"vision_model.encoder.layers.11.norm2.weight": "model-00001-of-00004.safetensors",
|
||||
"vision_model.encoder.layers.12.attn.proj.bias": "model-00001-of-00004.safetensors",
|
||||
"vision_model.encoder.layers.12.attn.proj.weight": "model-00001-of-00004.safetensors",
|
||||
"vision_model.encoder.layers.12.attn.qkv.bias": "model-00001-of-00004.safetensors",
|
||||
"vision_model.encoder.layers.12.attn.qkv.weight": "model-00001-of-00004.safetensors",
|
||||
"vision_model.encoder.layers.12.ls1": "model-00001-of-00004.safetensors",
|
||||
"vision_model.encoder.layers.12.ls2": "model-00001-of-00004.safetensors",
|
||||
"vision_model.encoder.layers.12.mlp.fc1.bias": "model-00001-of-00004.safetensors",
|
||||
"vision_model.encoder.layers.12.mlp.fc1.weight": "model-00001-of-00004.safetensors",
|
||||
"vision_model.encoder.layers.12.mlp.fc2.bias": "model-00001-of-00004.safetensors",
|
||||
"vision_model.encoder.layers.12.mlp.fc2.weight": "model-00001-of-00004.safetensors",
|
||||
"vision_model.encoder.layers.12.norm1.bias": "model-00001-of-00004.safetensors",
|
||||
"vision_model.encoder.layers.12.norm1.weight": "model-00001-of-00004.safetensors",
|
||||
"vision_model.encoder.layers.12.norm2.bias": "model-00001-of-00004.safetensors",
|
||||
"vision_model.encoder.layers.12.norm2.weight": "model-00001-of-00004.safetensors",
|
||||
"vision_model.encoder.layers.13.attn.proj.bias": "model-00001-of-00004.safetensors",
|
||||
"vision_model.encoder.layers.13.attn.proj.weight": "model-00001-of-00004.safetensors",
|
||||
"vision_model.encoder.layers.13.attn.qkv.bias": "model-00001-of-00004.safetensors",
|
||||
"vision_model.encoder.layers.13.attn.qkv.weight": "model-00001-of-00004.safetensors",
|
||||
"vision_model.encoder.layers.13.ls1": "model-00001-of-00004.safetensors",
|
||||
"vision_model.encoder.layers.13.ls2": "model-00001-of-00004.safetensors",
|
||||
"vision_model.encoder.layers.13.mlp.fc1.bias": "model-00001-of-00004.safetensors",
|
||||
"vision_model.encoder.layers.13.mlp.fc1.weight": "model-00001-of-00004.safetensors",
|
||||
"vision_model.encoder.layers.13.mlp.fc2.bias": "model-00001-of-00004.safetensors",
|
||||
"vision_model.encoder.layers.13.mlp.fc2.weight": "model-00001-of-00004.safetensors",
|
||||
"vision_model.encoder.layers.13.norm1.bias": "model-00001-of-00004.safetensors",
|
||||
"vision_model.encoder.layers.13.norm1.weight": "model-00001-of-00004.safetensors",
|
||||
"vision_model.encoder.layers.13.norm2.bias": "model-00001-of-00004.safetensors",
|
||||
"vision_model.encoder.layers.13.norm2.weight": "model-00001-of-00004.safetensors",
|
||||
"vision_model.encoder.layers.14.attn.proj.bias": "model-00001-of-00004.safetensors",
|
||||
"vision_model.encoder.layers.14.attn.proj.weight": "model-00001-of-00004.safetensors",
|
||||
"vision_model.encoder.layers.14.attn.qkv.bias": "model-00001-of-00004.safetensors",
|
||||
"vision_model.encoder.layers.14.attn.qkv.weight": "model-00001-of-00004.safetensors",
|
||||
"vision_model.encoder.layers.14.ls1": "model-00001-of-00004.safetensors",
|
||||
"vision_model.encoder.layers.14.ls2": "model-00001-of-00004.safetensors",
|
||||
"vision_model.encoder.layers.14.mlp.fc1.bias": "model-00001-of-00004.safetensors",
|
||||
"vision_model.encoder.layers.14.mlp.fc1.weight": "model-00001-of-00004.safetensors",
|
||||
"vision_model.encoder.layers.14.mlp.fc2.bias": "model-00001-of-00004.safetensors",
|
||||
"vision_model.encoder.layers.14.mlp.fc2.weight": "model-00001-of-00004.safetensors",
|
||||
"vision_model.encoder.layers.14.norm1.bias": "model-00001-of-00004.safetensors",
|
||||
"vision_model.encoder.layers.14.norm1.weight": "model-00001-of-00004.safetensors",
|
||||
"vision_model.encoder.layers.14.norm2.bias": "model-00001-of-00004.safetensors",
|
||||
"vision_model.encoder.layers.14.norm2.weight": "model-00001-of-00004.safetensors",
|
||||
"vision_model.encoder.layers.15.attn.proj.bias": "model-00001-of-00004.safetensors",
|
||||
"vision_model.encoder.layers.15.attn.proj.weight": "model-00001-of-00004.safetensors",
|
||||
"vision_model.encoder.layers.15.attn.qkv.bias": "model-00001-of-00004.safetensors",
|
||||
"vision_model.encoder.layers.15.attn.qkv.weight": "model-00001-of-00004.safetensors",
|
||||
"vision_model.encoder.layers.15.ls1": "model-00001-of-00004.safetensors",
|
||||
"vision_model.encoder.layers.15.ls2": "model-00001-of-00004.safetensors",
|
||||
"vision_model.encoder.layers.15.mlp.fc1.bias": "model-00001-of-00004.safetensors",
|
||||
"vision_model.encoder.layers.15.mlp.fc1.weight": "model-00001-of-00004.safetensors",
|
||||
"vision_model.encoder.layers.15.mlp.fc2.bias": "model-00001-of-00004.safetensors",
|
||||
"vision_model.encoder.layers.15.mlp.fc2.weight": "model-00001-of-00004.safetensors",
|
||||
"vision_model.encoder.layers.15.norm1.bias": "model-00001-of-00004.safetensors",
|
||||
"vision_model.encoder.layers.15.norm1.weight": "model-00001-of-00004.safetensors",
|
||||
"vision_model.encoder.layers.15.norm2.bias": "model-00001-of-00004.safetensors",
|
||||
"vision_model.encoder.layers.15.norm2.weight": "model-00001-of-00004.safetensors",
|
||||
"vision_model.encoder.layers.16.attn.proj.bias": "model-00001-of-00004.safetensors",
|
||||
"vision_model.encoder.layers.16.attn.proj.weight": "model-00001-of-00004.safetensors",
|
||||
"vision_model.encoder.layers.16.attn.qkv.bias": "model-00001-of-00004.safetensors",
|
||||
"vision_model.encoder.layers.16.attn.qkv.weight": "model-00001-of-00004.safetensors",
|
||||
"vision_model.encoder.layers.16.ls1": "model-00001-of-00004.safetensors",
|
||||
"vision_model.encoder.layers.16.ls2": "model-00001-of-00004.safetensors",
|
||||
"vision_model.encoder.layers.16.mlp.fc1.bias": "model-00001-of-00004.safetensors",
|
||||
"vision_model.encoder.layers.16.mlp.fc1.weight": "model-00001-of-00004.safetensors",
|
||||
"vision_model.encoder.layers.16.mlp.fc2.bias": "model-00001-of-00004.safetensors",
|
||||
"vision_model.encoder.layers.16.mlp.fc2.weight": "model-00001-of-00004.safetensors",
|
||||
"vision_model.encoder.layers.16.norm1.bias": "model-00001-of-00004.safetensors",
|
||||
"vision_model.encoder.layers.16.norm1.weight": "model-00001-of-00004.safetensors",
|
||||
"vision_model.encoder.layers.16.norm2.bias": "model-00001-of-00004.safetensors",
|
||||
"vision_model.encoder.layers.16.norm2.weight": "model-00001-of-00004.safetensors",
|
||||
"vision_model.encoder.layers.17.attn.proj.bias": "model-00001-of-00004.safetensors",
|
||||
"vision_model.encoder.layers.17.attn.proj.weight": "model-00001-of-00004.safetensors",
|
||||
"vision_model.encoder.layers.17.attn.qkv.bias": "model-00001-of-00004.safetensors",
|
||||
"vision_model.encoder.layers.17.attn.qkv.weight": "model-00001-of-00004.safetensors",
|
||||
"vision_model.encoder.layers.17.ls1": "model-00001-of-00004.safetensors",
|
||||
"vision_model.encoder.layers.17.ls2": "model-00001-of-00004.safetensors",
|
||||
"vision_model.encoder.layers.17.mlp.fc1.bias": "model-00001-of-00004.safetensors",
|
||||
"vision_model.encoder.layers.17.mlp.fc1.weight": "model-00001-of-00004.safetensors",
|
||||
"vision_model.encoder.layers.17.mlp.fc2.bias": "model-00001-of-00004.safetensors",
|
||||
"vision_model.encoder.layers.17.mlp.fc2.weight": "model-00001-of-00004.safetensors",
|
||||
"vision_model.encoder.layers.17.norm1.bias": "model-00001-of-00004.safetensors",
|
||||
"vision_model.encoder.layers.17.norm1.weight": "model-00001-of-00004.safetensors",
|
||||
"vision_model.encoder.layers.17.norm2.bias": "model-00001-of-00004.safetensors",
|
||||
"vision_model.encoder.layers.17.norm2.weight": "model-00001-of-00004.safetensors",
|
||||
"vision_model.encoder.layers.18.attn.proj.bias": "model-00001-of-00004.safetensors",
|
||||
"vision_model.encoder.layers.18.attn.proj.weight": "model-00001-of-00004.safetensors",
|
||||
"vision_model.encoder.layers.18.attn.qkv.bias": "model-00001-of-00004.safetensors",
|
||||
"vision_model.encoder.layers.18.attn.qkv.weight": "model-00001-of-00004.safetensors",
|
||||
"vision_model.encoder.layers.18.ls1": "model-00001-of-00004.safetensors",
|
||||
"vision_model.encoder.layers.18.ls2": "model-00001-of-00004.safetensors",
|
||||
"vision_model.encoder.layers.18.mlp.fc1.bias": "model-00001-of-00004.safetensors",
|
||||
"vision_model.encoder.layers.18.mlp.fc1.weight": "model-00001-of-00004.safetensors",
|
||||
"vision_model.encoder.layers.18.mlp.fc2.bias": "model-00001-of-00004.safetensors",
|
||||
"vision_model.encoder.layers.18.mlp.fc2.weight": "model-00001-of-00004.safetensors",
|
||||
"vision_model.encoder.layers.18.norm1.bias": "model-00001-of-00004.safetensors",
|
||||
"vision_model.encoder.layers.18.norm1.weight": "model-00001-of-00004.safetensors",
|
||||
"vision_model.encoder.layers.18.norm2.bias": "model-00001-of-00004.safetensors",
|
||||
"vision_model.encoder.layers.18.norm2.weight": "model-00001-of-00004.safetensors",
|
||||
"vision_model.encoder.layers.19.attn.proj.bias": "model-00001-of-00004.safetensors",
|
||||
"vision_model.encoder.layers.19.attn.proj.weight": "model-00001-of-00004.safetensors",
|
||||
"vision_model.encoder.layers.19.attn.qkv.bias": "model-00001-of-00004.safetensors",
|
||||
"vision_model.encoder.layers.19.attn.qkv.weight": "model-00001-of-00004.safetensors",
|
||||
"vision_model.encoder.layers.19.ls1": "model-00001-of-00004.safetensors",
|
||||
"vision_model.encoder.layers.19.ls2": "model-00001-of-00004.safetensors",
|
||||
"vision_model.encoder.layers.19.mlp.fc1.bias": "model-00001-of-00004.safetensors",
|
||||
"vision_model.encoder.layers.19.mlp.fc1.weight": "model-00001-of-00004.safetensors",
|
||||
"vision_model.encoder.layers.19.mlp.fc2.bias": "model-00001-of-00004.safetensors",
|
||||
"vision_model.encoder.layers.19.mlp.fc2.weight": "model-00001-of-00004.safetensors",
|
||||
"vision_model.encoder.layers.19.norm1.bias": "model-00001-of-00004.safetensors",
|
||||
"vision_model.encoder.layers.19.norm1.weight": "model-00001-of-00004.safetensors",
|
||||
"vision_model.encoder.layers.19.norm2.bias": "model-00001-of-00004.safetensors",
|
||||
"vision_model.encoder.layers.19.norm2.weight": "model-00001-of-00004.safetensors",
|
||||
"vision_model.encoder.layers.2.attn.proj.bias": "model-00001-of-00004.safetensors",
|
||||
"vision_model.encoder.layers.2.attn.proj.weight": "model-00001-of-00004.safetensors",
|
||||
"vision_model.encoder.layers.2.attn.qkv.bias": "model-00001-of-00004.safetensors",
|
||||
"vision_model.encoder.layers.2.attn.qkv.weight": "model-00001-of-00004.safetensors",
|
||||
"vision_model.encoder.layers.2.ls1": "model-00001-of-00004.safetensors",
|
||||
"vision_model.encoder.layers.2.ls2": "model-00001-of-00004.safetensors",
|
||||
"vision_model.encoder.layers.2.mlp.fc1.bias": "model-00001-of-00004.safetensors",
|
||||
"vision_model.encoder.layers.2.mlp.fc1.weight": "model-00001-of-00004.safetensors",
|
||||
"vision_model.encoder.layers.2.mlp.fc2.bias": "model-00001-of-00004.safetensors",
|
||||
"vision_model.encoder.layers.2.mlp.fc2.weight": "model-00001-of-00004.safetensors",
|
||||
"vision_model.encoder.layers.2.norm1.bias": "model-00001-of-00004.safetensors",
|
||||
"vision_model.encoder.layers.2.norm1.weight": "model-00001-of-00004.safetensors",
|
||||
"vision_model.encoder.layers.2.norm2.bias": "model-00001-of-00004.safetensors",
|
||||
"vision_model.encoder.layers.2.norm2.weight": "model-00001-of-00004.safetensors",
|
||||
"vision_model.encoder.layers.20.attn.proj.bias": "model-00001-of-00004.safetensors",
|
||||
"vision_model.encoder.layers.20.attn.proj.weight": "model-00001-of-00004.safetensors",
|
||||
"vision_model.encoder.layers.20.attn.qkv.bias": "model-00001-of-00004.safetensors",
|
||||
"vision_model.encoder.layers.20.attn.qkv.weight": "model-00001-of-00004.safetensors",
|
||||
"vision_model.encoder.layers.20.ls1": "model-00001-of-00004.safetensors",
|
||||
"vision_model.encoder.layers.20.ls2": "model-00001-of-00004.safetensors",
|
||||
"vision_model.encoder.layers.20.mlp.fc1.bias": "model-00001-of-00004.safetensors",
|
||||
"vision_model.encoder.layers.20.mlp.fc1.weight": "model-00001-of-00004.safetensors",
|
||||
"vision_model.encoder.layers.20.mlp.fc2.bias": "model-00001-of-00004.safetensors",
|
||||
"vision_model.encoder.layers.20.mlp.fc2.weight": "model-00001-of-00004.safetensors",
|
||||
"vision_model.encoder.layers.20.norm1.bias": "model-00001-of-00004.safetensors",
|
||||
"vision_model.encoder.layers.20.norm1.weight": "model-00001-of-00004.safetensors",
|
||||
"vision_model.encoder.layers.20.norm2.bias": "model-00001-of-00004.safetensors",
|
||||
"vision_model.encoder.layers.20.norm2.weight": "model-00001-of-00004.safetensors",
|
||||
"vision_model.encoder.layers.21.attn.proj.bias": "model-00001-of-00004.safetensors",
|
||||
"vision_model.encoder.layers.21.attn.proj.weight": "model-00001-of-00004.safetensors",
|
||||
"vision_model.encoder.layers.21.attn.qkv.bias": "model-00001-of-00004.safetensors",
|
||||
"vision_model.encoder.layers.21.attn.qkv.weight": "model-00001-of-00004.safetensors",
|
||||
"vision_model.encoder.layers.21.ls1": "model-00001-of-00004.safetensors",
|
||||
"vision_model.encoder.layers.21.ls2": "model-00001-of-00004.safetensors",
|
||||
"vision_model.encoder.layers.21.mlp.fc1.bias": "model-00001-of-00004.safetensors",
|
||||
"vision_model.encoder.layers.21.mlp.fc1.weight": "model-00001-of-00004.safetensors",
|
||||
"vision_model.encoder.layers.21.mlp.fc2.bias": "model-00001-of-00004.safetensors",
|
||||
"vision_model.encoder.layers.21.mlp.fc2.weight": "model-00001-of-00004.safetensors",
|
||||
"vision_model.encoder.layers.21.norm1.bias": "model-00001-of-00004.safetensors",
|
||||
"vision_model.encoder.layers.21.norm1.weight": "model-00001-of-00004.safetensors",
|
||||
"vision_model.encoder.layers.21.norm2.bias": "model-00001-of-00004.safetensors",
|
||||
"vision_model.encoder.layers.21.norm2.weight": "model-00001-of-00004.safetensors",
|
||||
"vision_model.encoder.layers.22.attn.proj.bias": "model-00001-of-00004.safetensors",
|
||||
"vision_model.encoder.layers.22.attn.proj.weight": "model-00001-of-00004.safetensors",
|
||||
"vision_model.encoder.layers.22.attn.qkv.bias": "model-00001-of-00004.safetensors",
|
||||
"vision_model.encoder.layers.22.attn.qkv.weight": "model-00001-of-00004.safetensors",
|
||||
"vision_model.encoder.layers.22.ls1": "model-00001-of-00004.safetensors",
|
||||
"vision_model.encoder.layers.22.ls2": "model-00001-of-00004.safetensors",
|
||||
"vision_model.encoder.layers.22.mlp.fc1.bias": "model-00001-of-00004.safetensors",
|
||||
"vision_model.encoder.layers.22.mlp.fc1.weight": "model-00001-of-00004.safetensors",
|
||||
"vision_model.encoder.layers.22.mlp.fc2.bias": "model-00001-of-00004.safetensors",
|
||||
"vision_model.encoder.layers.22.mlp.fc2.weight": "model-00001-of-00004.safetensors",
|
||||
"vision_model.encoder.layers.22.norm1.bias": "model-00001-of-00004.safetensors",
|
||||
"vision_model.encoder.layers.22.norm1.weight": "model-00001-of-00004.safetensors",
|
||||
"vision_model.encoder.layers.22.norm2.bias": "model-00001-of-00004.safetensors",
|
||||
"vision_model.encoder.layers.22.norm2.weight": "model-00001-of-00004.safetensors",
|
||||
"vision_model.encoder.layers.23.attn.proj.bias": "model-00001-of-00004.safetensors",
|
||||
"vision_model.encoder.layers.23.attn.proj.weight": "model-00001-of-00004.safetensors",
|
||||
"vision_model.encoder.layers.23.attn.qkv.bias": "model-00001-of-00004.safetensors",
|
||||
"vision_model.encoder.layers.23.attn.qkv.weight": "model-00001-of-00004.safetensors",
|
||||
"vision_model.encoder.layers.23.ls1": "model-00001-of-00004.safetensors",
|
||||
"vision_model.encoder.layers.23.ls2": "model-00001-of-00004.safetensors",
|
||||
"vision_model.encoder.layers.23.mlp.fc1.bias": "model-00001-of-00004.safetensors",
|
||||
"vision_model.encoder.layers.23.mlp.fc1.weight": "model-00001-of-00004.safetensors",
|
||||
"vision_model.encoder.layers.23.mlp.fc2.bias": "model-00001-of-00004.safetensors",
|
||||
"vision_model.encoder.layers.23.mlp.fc2.weight": "model-00001-of-00004.safetensors",
|
||||
"vision_model.encoder.layers.23.norm1.bias": "model-00001-of-00004.safetensors",
|
||||
"vision_model.encoder.layers.23.norm1.weight": "model-00001-of-00004.safetensors",
|
||||
"vision_model.encoder.layers.23.norm2.bias": "model-00001-of-00004.safetensors",
|
||||
"vision_model.encoder.layers.23.norm2.weight": "model-00001-of-00004.safetensors",
|
||||
"vision_model.encoder.layers.3.attn.proj.bias": "model-00001-of-00004.safetensors",
|
||||
"vision_model.encoder.layers.3.attn.proj.weight": "model-00001-of-00004.safetensors",
|
||||
"vision_model.encoder.layers.3.attn.qkv.bias": "model-00001-of-00004.safetensors",
|
||||
"vision_model.encoder.layers.3.attn.qkv.weight": "model-00001-of-00004.safetensors",
|
||||
"vision_model.encoder.layers.3.ls1": "model-00001-of-00004.safetensors",
|
||||
"vision_model.encoder.layers.3.ls2": "model-00001-of-00004.safetensors",
|
||||
"vision_model.encoder.layers.3.mlp.fc1.bias": "model-00001-of-00004.safetensors",
|
||||
"vision_model.encoder.layers.3.mlp.fc1.weight": "model-00001-of-00004.safetensors",
|
||||
"vision_model.encoder.layers.3.mlp.fc2.bias": "model-00001-of-00004.safetensors",
|
||||
"vision_model.encoder.layers.3.mlp.fc2.weight": "model-00001-of-00004.safetensors",
|
||||
"vision_model.encoder.layers.3.norm1.bias": "model-00001-of-00004.safetensors",
|
||||
"vision_model.encoder.layers.3.norm1.weight": "model-00001-of-00004.safetensors",
|
||||
"vision_model.encoder.layers.3.norm2.bias": "model-00001-of-00004.safetensors",
|
||||
"vision_model.encoder.layers.3.norm2.weight": "model-00001-of-00004.safetensors",
|
||||
"vision_model.encoder.layers.4.attn.proj.bias": "model-00001-of-00004.safetensors",
|
||||
"vision_model.encoder.layers.4.attn.proj.weight": "model-00001-of-00004.safetensors",
|
||||
"vision_model.encoder.layers.4.attn.qkv.bias": "model-00001-of-00004.safetensors",
|
||||
"vision_model.encoder.layers.4.attn.qkv.weight": "model-00001-of-00004.safetensors",
|
||||
"vision_model.encoder.layers.4.ls1": "model-00001-of-00004.safetensors",
|
||||
"vision_model.encoder.layers.4.ls2": "model-00001-of-00004.safetensors",
|
||||
"vision_model.encoder.layers.4.mlp.fc1.bias": "model-00001-of-00004.safetensors",
|
||||
"vision_model.encoder.layers.4.mlp.fc1.weight": "model-00001-of-00004.safetensors",
|
||||
"vision_model.encoder.layers.4.mlp.fc2.bias": "model-00001-of-00004.safetensors",
|
||||
"vision_model.encoder.layers.4.mlp.fc2.weight": "model-00001-of-00004.safetensors",
|
||||
"vision_model.encoder.layers.4.norm1.bias": "model-00001-of-00004.safetensors",
|
||||
"vision_model.encoder.layers.4.norm1.weight": "model-00001-of-00004.safetensors",
|
||||
"vision_model.encoder.layers.4.norm2.bias": "model-00001-of-00004.safetensors",
|
||||
"vision_model.encoder.layers.4.norm2.weight": "model-00001-of-00004.safetensors",
|
||||
"vision_model.encoder.layers.5.attn.proj.bias": "model-00001-of-00004.safetensors",
|
||||
"vision_model.encoder.layers.5.attn.proj.weight": "model-00001-of-00004.safetensors",
|
||||
"vision_model.encoder.layers.5.attn.qkv.bias": "model-00001-of-00004.safetensors",
|
||||
"vision_model.encoder.layers.5.attn.qkv.weight": "model-00001-of-00004.safetensors",
|
||||
"vision_model.encoder.layers.5.ls1": "model-00001-of-00004.safetensors",
|
||||
"vision_model.encoder.layers.5.ls2": "model-00001-of-00004.safetensors",
|
||||
"vision_model.encoder.layers.5.mlp.fc1.bias": "model-00001-of-00004.safetensors",
|
||||
"vision_model.encoder.layers.5.mlp.fc1.weight": "model-00001-of-00004.safetensors",
|
||||
"vision_model.encoder.layers.5.mlp.fc2.bias": "model-00001-of-00004.safetensors",
|
||||
"vision_model.encoder.layers.5.mlp.fc2.weight": "model-00001-of-00004.safetensors",
|
||||
"vision_model.encoder.layers.5.norm1.bias": "model-00001-of-00004.safetensors",
|
||||
"vision_model.encoder.layers.5.norm1.weight": "model-00001-of-00004.safetensors",
|
||||
"vision_model.encoder.layers.5.norm2.bias": "model-00001-of-00004.safetensors",
|
||||
"vision_model.encoder.layers.5.norm2.weight": "model-00001-of-00004.safetensors",
|
||||
"vision_model.encoder.layers.6.attn.proj.bias": "model-00001-of-00004.safetensors",
|
||||
"vision_model.encoder.layers.6.attn.proj.weight": "model-00001-of-00004.safetensors",
|
||||
"vision_model.encoder.layers.6.attn.qkv.bias": "model-00001-of-00004.safetensors",
|
||||
"vision_model.encoder.layers.6.attn.qkv.weight": "model-00001-of-00004.safetensors",
|
||||
"vision_model.encoder.layers.6.ls1": "model-00001-of-00004.safetensors",
|
||||
"vision_model.encoder.layers.6.ls2": "model-00001-of-00004.safetensors",
|
||||
"vision_model.encoder.layers.6.mlp.fc1.bias": "model-00001-of-00004.safetensors",
|
||||
"vision_model.encoder.layers.6.mlp.fc1.weight": "model-00001-of-00004.safetensors",
|
||||
"vision_model.encoder.layers.6.mlp.fc2.bias": "model-00001-of-00004.safetensors",
|
||||
"vision_model.encoder.layers.6.mlp.fc2.weight": "model-00001-of-00004.safetensors",
|
||||
"vision_model.encoder.layers.6.norm1.bias": "model-00001-of-00004.safetensors",
|
||||
"vision_model.encoder.layers.6.norm1.weight": "model-00001-of-00004.safetensors",
|
||||
"vision_model.encoder.layers.6.norm2.bias": "model-00001-of-00004.safetensors",
|
||||
"vision_model.encoder.layers.6.norm2.weight": "model-00001-of-00004.safetensors",
|
||||
"vision_model.encoder.layers.7.attn.proj.bias": "model-00001-of-00004.safetensors",
|
||||
"vision_model.encoder.layers.7.attn.proj.weight": "model-00001-of-00004.safetensors",
|
||||
"vision_model.encoder.layers.7.attn.qkv.bias": "model-00001-of-00004.safetensors",
|
||||
"vision_model.encoder.layers.7.attn.qkv.weight": "model-00001-of-00004.safetensors",
|
||||
"vision_model.encoder.layers.7.ls1": "model-00001-of-00004.safetensors",
|
||||
"vision_model.encoder.layers.7.ls2": "model-00001-of-00004.safetensors",
|
||||
"vision_model.encoder.layers.7.mlp.fc1.bias": "model-00001-of-00004.safetensors",
|
||||
"vision_model.encoder.layers.7.mlp.fc1.weight": "model-00001-of-00004.safetensors",
|
||||
"vision_model.encoder.layers.7.mlp.fc2.bias": "model-00001-of-00004.safetensors",
|
||||
"vision_model.encoder.layers.7.mlp.fc2.weight": "model-00001-of-00004.safetensors",
|
||||
"vision_model.encoder.layers.7.norm1.bias": "model-00001-of-00004.safetensors",
|
||||
"vision_model.encoder.layers.7.norm1.weight": "model-00001-of-00004.safetensors",
|
||||
"vision_model.encoder.layers.7.norm2.bias": "model-00001-of-00004.safetensors",
|
||||
"vision_model.encoder.layers.7.norm2.weight": "model-00001-of-00004.safetensors",
|
||||
"vision_model.encoder.layers.8.attn.proj.bias": "model-00001-of-00004.safetensors",
|
||||
"vision_model.encoder.layers.8.attn.proj.weight": "model-00001-of-00004.safetensors",
|
||||
"vision_model.encoder.layers.8.attn.qkv.bias": "model-00001-of-00004.safetensors",
|
||||
"vision_model.encoder.layers.8.attn.qkv.weight": "model-00001-of-00004.safetensors",
|
||||
"vision_model.encoder.layers.8.ls1": "model-00001-of-00004.safetensors",
|
||||
"vision_model.encoder.layers.8.ls2": "model-00001-of-00004.safetensors",
|
||||
"vision_model.encoder.layers.8.mlp.fc1.bias": "model-00001-of-00004.safetensors",
|
||||
"vision_model.encoder.layers.8.mlp.fc1.weight": "model-00001-of-00004.safetensors",
|
||||
"vision_model.encoder.layers.8.mlp.fc2.bias": "model-00001-of-00004.safetensors",
|
||||
"vision_model.encoder.layers.8.mlp.fc2.weight": "model-00001-of-00004.safetensors",
|
||||
"vision_model.encoder.layers.8.norm1.bias": "model-00001-of-00004.safetensors",
|
||||
"vision_model.encoder.layers.8.norm1.weight": "model-00001-of-00004.safetensors",
|
||||
"vision_model.encoder.layers.8.norm2.bias": "model-00001-of-00004.safetensors",
|
||||
"vision_model.encoder.layers.8.norm2.weight": "model-00001-of-00004.safetensors",
|
||||
"vision_model.encoder.layers.9.attn.proj.bias": "model-00001-of-00004.safetensors",
|
||||
"vision_model.encoder.layers.9.attn.proj.weight": "model-00001-of-00004.safetensors",
|
||||
"vision_model.encoder.layers.9.attn.qkv.bias": "model-00001-of-00004.safetensors",
|
||||
"vision_model.encoder.layers.9.attn.qkv.weight": "model-00001-of-00004.safetensors",
|
||||
"vision_model.encoder.layers.9.ls1": "model-00001-of-00004.safetensors",
|
||||
"vision_model.encoder.layers.9.ls2": "model-00001-of-00004.safetensors",
|
||||
"vision_model.encoder.layers.9.mlp.fc1.bias": "model-00001-of-00004.safetensors",
|
||||
"vision_model.encoder.layers.9.mlp.fc1.weight": "model-00001-of-00004.safetensors",
|
||||
"vision_model.encoder.layers.9.mlp.fc2.bias": "model-00001-of-00004.safetensors",
|
||||
"vision_model.encoder.layers.9.mlp.fc2.weight": "model-00001-of-00004.safetensors",
|
||||
"vision_model.encoder.layers.9.norm1.bias": "model-00001-of-00004.safetensors",
|
||||
"vision_model.encoder.layers.9.norm1.weight": "model-00001-of-00004.safetensors",
|
||||
"vision_model.encoder.layers.9.norm2.bias": "model-00001-of-00004.safetensors",
|
||||
"vision_model.encoder.layers.9.norm2.weight": "model-00001-of-00004.safetensors"
|
||||
}
|
||||
}
|
|
@ -0,0 +1,430 @@
|
|||
# --------------------------------------------------------
|
||||
# InternVL
|
||||
# Copyright (c) 2024 OpenGVLab
|
||||
# Licensed under The MIT License [see LICENSE for details]
|
||||
# --------------------------------------------------------
|
||||
|
||||
from typing import Optional, Tuple, Union
|
||||
|
||||
import torch
|
||||
import torch.nn.functional as F
|
||||
import torch.utils.checkpoint
|
||||
from einops import rearrange
|
||||
from timm.models.layers import DropPath
|
||||
from torch import nn
|
||||
from transformers.activations import ACT2FN
|
||||
from transformers.modeling_outputs import (BaseModelOutput,
|
||||
BaseModelOutputWithPooling)
|
||||
from transformers.modeling_utils import PreTrainedModel
|
||||
from transformers.utils import logging
|
||||
|
||||
from .configuration_intern_vit import InternVisionConfig
|
||||
|
||||
try:
|
||||
from flash_attn.bert_padding import pad_input, unpad_input
|
||||
from flash_attn.flash_attn_interface import \
|
||||
flash_attn_varlen_qkvpacked_func
|
||||
has_flash_attn = True
|
||||
except:
|
||||
print('FlashAttention2 is not installed.')
|
||||
has_flash_attn = False
|
||||
|
||||
logger = logging.get_logger(__name__)
|
||||
|
||||
|
||||
class FlashAttention(nn.Module):
|
||||
"""Implement the scaled dot product attention with softmax.
|
||||
Arguments
|
||||
---------
|
||||
softmax_scale: The temperature to use for the softmax attention.
|
||||
(default: 1/sqrt(d_keys) where d_keys is computed at
|
||||
runtime)
|
||||
attention_dropout: The dropout rate to apply to the attention
|
||||
(default: 0.0)
|
||||
"""
|
||||
|
||||
def __init__(self, softmax_scale=None, attention_dropout=0.0, device=None, dtype=None):
|
||||
super().__init__()
|
||||
self.softmax_scale = softmax_scale
|
||||
self.dropout_p = attention_dropout
|
||||
|
||||
def forward(self, qkv, key_padding_mask=None, causal=False, cu_seqlens=None,
|
||||
max_s=None, need_weights=False):
|
||||
"""Implements the multihead softmax attention.
|
||||
Arguments
|
||||
---------
|
||||
qkv: The tensor containing the query, key, and value. (B, S, 3, H, D) if key_padding_mask is None
|
||||
if unpadded: (nnz, 3, h, d)
|
||||
key_padding_mask: a bool tensor of shape (B, S)
|
||||
"""
|
||||
assert not need_weights
|
||||
assert qkv.dtype in [torch.float16, torch.bfloat16]
|
||||
assert qkv.is_cuda
|
||||
|
||||
if cu_seqlens is None:
|
||||
batch_size = qkv.shape[0]
|
||||
seqlen = qkv.shape[1]
|
||||
if key_padding_mask is None:
|
||||
qkv = rearrange(qkv, 'b s ... -> (b s) ...')
|
||||
max_s = seqlen
|
||||
cu_seqlens = torch.arange(0, (batch_size + 1) * seqlen, step=seqlen, dtype=torch.int32,
|
||||
device=qkv.device)
|
||||
output = flash_attn_varlen_qkvpacked_func(
|
||||
qkv, cu_seqlens, max_s, self.dropout_p if self.training else 0.0,
|
||||
softmax_scale=self.softmax_scale, causal=causal
|
||||
)
|
||||
output = rearrange(output, '(b s) ... -> b s ...', b=batch_size)
|
||||
else:
|
||||
nheads = qkv.shape[-2]
|
||||
x = rearrange(qkv, 'b s three h d -> b s (three h d)')
|
||||
x_unpad, indices, cu_seqlens, max_s = unpad_input(x, key_padding_mask)
|
||||
x_unpad = rearrange(x_unpad, 'nnz (three h d) -> nnz three h d', three=3, h=nheads)
|
||||
output_unpad = flash_attn_varlen_qkvpacked_func(
|
||||
x_unpad, cu_seqlens, max_s, self.dropout_p if self.training else 0.0,
|
||||
softmax_scale=self.softmax_scale, causal=causal
|
||||
)
|
||||
output = rearrange(pad_input(rearrange(output_unpad, 'nnz h d -> nnz (h d)'),
|
||||
indices, batch_size, seqlen),
|
||||
'b s (h d) -> b s h d', h=nheads)
|
||||
else:
|
||||
assert max_s is not None
|
||||
output = flash_attn_varlen_qkvpacked_func(
|
||||
qkv, cu_seqlens, max_s, self.dropout_p if self.training else 0.0,
|
||||
softmax_scale=self.softmax_scale, causal=causal
|
||||
)
|
||||
|
||||
return output, None
|
||||
|
||||
|
||||
class InternRMSNorm(nn.Module):
|
||||
def __init__(self, hidden_size, eps=1e-6):
|
||||
super().__init__()
|
||||
self.weight = nn.Parameter(torch.ones(hidden_size))
|
||||
self.variance_epsilon = eps
|
||||
|
||||
def forward(self, hidden_states):
|
||||
input_dtype = hidden_states.dtype
|
||||
hidden_states = hidden_states.to(torch.float32)
|
||||
variance = hidden_states.pow(2).mean(-1, keepdim=True)
|
||||
hidden_states = hidden_states * torch.rsqrt(variance + self.variance_epsilon)
|
||||
return self.weight * hidden_states.to(input_dtype)
|
||||
|
||||
|
||||
try:
|
||||
from apex.normalization import FusedRMSNorm
|
||||
|
||||
InternRMSNorm = FusedRMSNorm # noqa
|
||||
|
||||
logger.info('Discovered apex.normalization.FusedRMSNorm - will use it instead of InternRMSNorm')
|
||||
except ImportError:
|
||||
# using the normal InternRMSNorm
|
||||
pass
|
||||
except Exception:
|
||||
logger.warning('discovered apex but it failed to load, falling back to InternRMSNorm')
|
||||
pass
|
||||
|
||||
|
||||
NORM2FN = {
|
||||
'rms_norm': InternRMSNorm,
|
||||
'layer_norm': nn.LayerNorm,
|
||||
}
|
||||
|
||||
|
||||
class InternVisionEmbeddings(nn.Module):
|
||||
def __init__(self, config: InternVisionConfig):
|
||||
super().__init__()
|
||||
self.config = config
|
||||
self.embed_dim = config.hidden_size
|
||||
self.image_size = config.image_size
|
||||
self.patch_size = config.patch_size
|
||||
|
||||
self.class_embedding = nn.Parameter(
|
||||
torch.randn(1, 1, self.embed_dim),
|
||||
)
|
||||
|
||||
self.patch_embedding = nn.Conv2d(
|
||||
in_channels=3, out_channels=self.embed_dim, kernel_size=self.patch_size, stride=self.patch_size
|
||||
)
|
||||
|
||||
self.num_patches = (self.image_size // self.patch_size) ** 2
|
||||
self.num_positions = self.num_patches + 1
|
||||
|
||||
self.position_embedding = nn.Parameter(torch.randn(1, self.num_positions, self.embed_dim))
|
||||
|
||||
def _get_pos_embed(self, pos_embed, H, W):
|
||||
target_dtype = pos_embed.dtype
|
||||
pos_embed = pos_embed.float().reshape(
|
||||
1, self.image_size // self.patch_size, self.image_size // self.patch_size, -1).permute(0, 3, 1, 2)
|
||||
pos_embed = F.interpolate(pos_embed, size=(H, W), mode='bicubic', align_corners=False). \
|
||||
reshape(1, -1, H * W).permute(0, 2, 1).to(target_dtype)
|
||||
return pos_embed
|
||||
|
||||
def forward(self, pixel_values: torch.FloatTensor) -> torch.Tensor:
|
||||
target_dtype = self.patch_embedding.weight.dtype
|
||||
patch_embeds = self.patch_embedding(pixel_values) # shape = [*, channel, width, height]
|
||||
batch_size, _, height, width = patch_embeds.shape
|
||||
patch_embeds = patch_embeds.flatten(2).transpose(1, 2)
|
||||
class_embeds = self.class_embedding.expand(batch_size, 1, -1).to(target_dtype)
|
||||
embeddings = torch.cat([class_embeds, patch_embeds], dim=1)
|
||||
position_embedding = torch.cat([
|
||||
self.position_embedding[:, :1, :],
|
||||
self._get_pos_embed(self.position_embedding[:, 1:, :], height, width)
|
||||
], dim=1)
|
||||
embeddings = embeddings + position_embedding.to(target_dtype)
|
||||
return embeddings
|
||||
|
||||
|
||||
class InternAttention(nn.Module):
|
||||
"""Multi-headed attention from 'Attention Is All You Need' paper"""
|
||||
|
||||
def __init__(self, config: InternVisionConfig):
|
||||
super().__init__()
|
||||
self.config = config
|
||||
self.embed_dim = config.hidden_size
|
||||
self.num_heads = config.num_attention_heads
|
||||
self.use_flash_attn = config.use_flash_attn and has_flash_attn
|
||||
if config.use_flash_attn and not has_flash_attn:
|
||||
print('Warning: Flash Attention is not available, use_flash_attn is set to False.')
|
||||
self.head_dim = self.embed_dim // self.num_heads
|
||||
if self.head_dim * self.num_heads != self.embed_dim:
|
||||
raise ValueError(
|
||||
f'embed_dim must be divisible by num_heads (got `embed_dim`: {self.embed_dim} and `num_heads`:'
|
||||
f' {self.num_heads}).'
|
||||
)
|
||||
|
||||
self.scale = self.head_dim ** -0.5
|
||||
self.qkv = nn.Linear(self.embed_dim, 3 * self.embed_dim, bias=config.qkv_bias)
|
||||
self.attn_drop = nn.Dropout(config.attention_dropout)
|
||||
self.proj_drop = nn.Dropout(config.dropout)
|
||||
|
||||
self.qk_normalization = config.qk_normalization
|
||||
|
||||
if self.qk_normalization:
|
||||
self.q_norm = InternRMSNorm(self.embed_dim, eps=config.layer_norm_eps)
|
||||
self.k_norm = InternRMSNorm(self.embed_dim, eps=config.layer_norm_eps)
|
||||
|
||||
if self.use_flash_attn:
|
||||
self.inner_attn = FlashAttention(attention_dropout=config.attention_dropout)
|
||||
self.proj = nn.Linear(self.embed_dim, self.embed_dim)
|
||||
|
||||
def _naive_attn(self, x):
|
||||
B, N, C = x.shape
|
||||
qkv = self.qkv(x).reshape(B, N, 3, self.num_heads, C // self.num_heads).permute(2, 0, 3, 1, 4)
|
||||
q, k, v = qkv.unbind(0) # make torchscript happy (cannot use tensor as tuple)
|
||||
|
||||
if self.qk_normalization:
|
||||
B_, H_, N_, D_ = q.shape
|
||||
q = self.q_norm(q.transpose(1, 2).flatten(-2, -1)).view(B_, N_, H_, D_).transpose(1, 2)
|
||||
k = self.k_norm(k.transpose(1, 2).flatten(-2, -1)).view(B_, N_, H_, D_).transpose(1, 2)
|
||||
|
||||
attn = ((q * self.scale) @ k.transpose(-2, -1))
|
||||
attn = attn.softmax(dim=-1)
|
||||
attn = self.attn_drop(attn)
|
||||
|
||||
x = (attn @ v).transpose(1, 2).reshape(B, N, C)
|
||||
x = self.proj(x)
|
||||
x = self.proj_drop(x)
|
||||
return x
|
||||
|
||||
def _flash_attn(self, x, key_padding_mask=None, need_weights=False):
|
||||
qkv = self.qkv(x)
|
||||
qkv = rearrange(qkv, 'b s (three h d) -> b s three h d', three=3, h=self.num_heads)
|
||||
|
||||
if self.qk_normalization:
|
||||
q, k, v = qkv.unbind(2)
|
||||
q = self.q_norm(q.flatten(-2, -1)).view(q.shape)
|
||||
k = self.k_norm(k.flatten(-2, -1)).view(k.shape)
|
||||
qkv = torch.stack([q, k, v], dim=2)
|
||||
|
||||
context, _ = self.inner_attn(
|
||||
qkv, key_padding_mask=key_padding_mask, need_weights=need_weights, causal=False
|
||||
)
|
||||
outs = self.proj(rearrange(context, 'b s h d -> b s (h d)'))
|
||||
outs = self.proj_drop(outs)
|
||||
return outs
|
||||
|
||||
def forward(self, hidden_states: torch.Tensor) -> torch.Tensor:
|
||||
x = self._naive_attn(hidden_states) if not self.use_flash_attn else self._flash_attn(hidden_states)
|
||||
return x
|
||||
|
||||
|
||||
class InternMLP(nn.Module):
|
||||
def __init__(self, config: InternVisionConfig):
|
||||
super().__init__()
|
||||
self.config = config
|
||||
self.act = ACT2FN[config.hidden_act]
|
||||
self.fc1 = nn.Linear(config.hidden_size, config.intermediate_size)
|
||||
self.fc2 = nn.Linear(config.intermediate_size, config.hidden_size)
|
||||
|
||||
def forward(self, hidden_states: torch.Tensor) -> torch.Tensor:
|
||||
hidden_states = self.fc1(hidden_states)
|
||||
hidden_states = self.act(hidden_states)
|
||||
hidden_states = self.fc2(hidden_states)
|
||||
return hidden_states
|
||||
|
||||
|
||||
class InternVisionEncoderLayer(nn.Module):
|
||||
def __init__(self, config: InternVisionConfig, drop_path_rate: float):
|
||||
super().__init__()
|
||||
self.embed_dim = config.hidden_size
|
||||
self.intermediate_size = config.intermediate_size
|
||||
self.norm_type = config.norm_type
|
||||
|
||||
self.attn = InternAttention(config)
|
||||
self.mlp = InternMLP(config)
|
||||
self.norm1 = NORM2FN[self.norm_type](self.embed_dim, eps=config.layer_norm_eps)
|
||||
self.norm2 = NORM2FN[self.norm_type](self.embed_dim, eps=config.layer_norm_eps)
|
||||
|
||||
self.ls1 = nn.Parameter(config.initializer_factor * torch.ones(self.embed_dim))
|
||||
self.ls2 = nn.Parameter(config.initializer_factor * torch.ones(self.embed_dim))
|
||||
self.drop_path1 = DropPath(drop_path_rate) if drop_path_rate > 0. else nn.Identity()
|
||||
self.drop_path2 = DropPath(drop_path_rate) if drop_path_rate > 0. else nn.Identity()
|
||||
|
||||
def forward(
|
||||
self,
|
||||
hidden_states: torch.Tensor,
|
||||
) -> Tuple[torch.FloatTensor, Optional[torch.FloatTensor], Optional[Tuple[torch.FloatTensor]]]:
|
||||
"""
|
||||
Args:
|
||||
hidden_states (`Tuple[torch.FloatTensor, Optional[torch.FloatTensor]]`): input to the layer of shape `(batch, seq_len, embed_dim)`
|
||||
"""
|
||||
hidden_states = hidden_states + self.drop_path1(self.attn(self.norm1(hidden_states).to(hidden_states.dtype)) * self.ls1)
|
||||
|
||||
hidden_states = hidden_states + self.drop_path2(self.mlp(self.norm2(hidden_states).to(hidden_states.dtype)) * self.ls2)
|
||||
|
||||
return hidden_states
|
||||
|
||||
|
||||
class InternVisionEncoder(nn.Module):
|
||||
"""
|
||||
Transformer encoder consisting of `config.num_hidden_layers` self attention layers. Each layer is a
|
||||
[`InternEncoderLayer`].
|
||||
|
||||
Args:
|
||||
config (`InternConfig`):
|
||||
The corresponding vision configuration for the `InternEncoder`.
|
||||
"""
|
||||
|
||||
def __init__(self, config: InternVisionConfig):
|
||||
super().__init__()
|
||||
self.config = config
|
||||
# stochastic depth decay rule
|
||||
dpr = [x.item() for x in torch.linspace(0, config.drop_path_rate, config.num_hidden_layers)]
|
||||
self.layers = nn.ModuleList([
|
||||
InternVisionEncoderLayer(config, dpr[idx]) for idx in range(config.num_hidden_layers)])
|
||||
self.gradient_checkpointing = True
|
||||
|
||||
def forward(
|
||||
self,
|
||||
inputs_embeds,
|
||||
output_hidden_states: Optional[bool] = None,
|
||||
return_dict: Optional[bool] = None,
|
||||
) -> Union[Tuple, BaseModelOutput]:
|
||||
r"""
|
||||
Args:
|
||||
inputs_embeds (`torch.FloatTensor` of shape `(batch_size, sequence_length, hidden_size)`):
|
||||
Embedded representation of the inputs. Should be float, not int tokens.
|
||||
output_hidden_states (`bool`, *optional*):
|
||||
Whether or not to return the hidden states of all layers. See `hidden_states` under returned tensors
|
||||
for more detail.
|
||||
return_dict (`bool`, *optional*):
|
||||
Whether or not to return a [`~utils.ModelOutput`] instead of a plain tuple.
|
||||
"""
|
||||
output_hidden_states = (
|
||||
output_hidden_states if output_hidden_states is not None else self.config.output_hidden_states
|
||||
)
|
||||
return_dict = return_dict if return_dict is not None else self.config.use_return_dict
|
||||
|
||||
encoder_states = () if output_hidden_states else None
|
||||
hidden_states = inputs_embeds
|
||||
|
||||
for idx, encoder_layer in enumerate(self.layers):
|
||||
if output_hidden_states:
|
||||
encoder_states = encoder_states + (hidden_states,)
|
||||
if self.gradient_checkpointing and self.training:
|
||||
layer_outputs = torch.utils.checkpoint.checkpoint(
|
||||
encoder_layer,
|
||||
hidden_states)
|
||||
else:
|
||||
layer_outputs = encoder_layer(
|
||||
hidden_states,
|
||||
)
|
||||
hidden_states = layer_outputs
|
||||
|
||||
if output_hidden_states:
|
||||
encoder_states = encoder_states + (hidden_states,)
|
||||
|
||||
if not return_dict:
|
||||
return tuple(v for v in [hidden_states, encoder_states] if v is not None)
|
||||
return BaseModelOutput(
|
||||
last_hidden_state=hidden_states, hidden_states=encoder_states
|
||||
)
|
||||
|
||||
|
||||
class InternVisionModel(PreTrainedModel):
|
||||
main_input_name = 'pixel_values'
|
||||
_supports_flash_attn_2 = True
|
||||
config_class = InternVisionConfig
|
||||
_no_split_modules = ['InternVisionEncoderLayer']
|
||||
|
||||
def __init__(self, config: InternVisionConfig):
|
||||
super().__init__(config)
|
||||
self.config = config
|
||||
|
||||
self.embeddings = InternVisionEmbeddings(config)
|
||||
self.encoder = InternVisionEncoder(config)
|
||||
|
||||
def resize_pos_embeddings(self, old_size, new_size, patch_size):
|
||||
pos_emb = self.embeddings.position_embedding
|
||||
_, num_positions, embed_dim = pos_emb.shape
|
||||
cls_emb = pos_emb[:, :1, :]
|
||||
pos_emb = pos_emb[:, 1:, :].reshape(1, old_size // patch_size, old_size // patch_size, -1).permute(0, 3, 1, 2)
|
||||
pos_emb = F.interpolate(pos_emb.float(), size=new_size // patch_size, mode='bicubic', align_corners=False)
|
||||
pos_emb = pos_emb.to(cls_emb.dtype).reshape(1, embed_dim, -1).permute(0, 2, 1)
|
||||
pos_emb = torch.cat([cls_emb, pos_emb], dim=1)
|
||||
self.embeddings.position_embedding = nn.Parameter(pos_emb)
|
||||
self.embeddings.image_size = new_size
|
||||
logger.info('Resized position embeddings from {} to {}'.format(old_size, new_size))
|
||||
|
||||
def get_input_embeddings(self):
|
||||
return self.embeddings
|
||||
|
||||
def forward(
|
||||
self,
|
||||
pixel_values: Optional[torch.FloatTensor] = None,
|
||||
output_hidden_states: Optional[bool] = None,
|
||||
return_dict: Optional[bool] = None,
|
||||
pixel_embeds: Optional[torch.FloatTensor] = None,
|
||||
) -> Union[Tuple, BaseModelOutputWithPooling]:
|
||||
output_hidden_states = (
|
||||
output_hidden_states if output_hidden_states is not None else self.config.output_hidden_states
|
||||
)
|
||||
return_dict = return_dict if return_dict is not None else self.config.use_return_dict
|
||||
|
||||
if pixel_values is None and pixel_embeds is None:
|
||||
raise ValueError('You have to specify pixel_values or pixel_embeds')
|
||||
|
||||
if pixel_embeds is not None:
|
||||
hidden_states = pixel_embeds
|
||||
else:
|
||||
if len(pixel_values.shape) == 4:
|
||||
hidden_states = self.embeddings(pixel_values)
|
||||
else:
|
||||
raise ValueError(f'wrong pixel_values size: {pixel_values.shape}')
|
||||
encoder_outputs = self.encoder(
|
||||
inputs_embeds=hidden_states,
|
||||
output_hidden_states=output_hidden_states,
|
||||
return_dict=return_dict,
|
||||
)
|
||||
last_hidden_state = encoder_outputs.last_hidden_state
|
||||
pooled_output = last_hidden_state[:, 0, :]
|
||||
|
||||
if not return_dict:
|
||||
return (last_hidden_state, pooled_output) + encoder_outputs[1:]
|
||||
|
||||
return BaseModelOutputWithPooling(
|
||||
last_hidden_state=last_hidden_state,
|
||||
pooler_output=pooled_output,
|
||||
hidden_states=encoder_outputs.hidden_states,
|
||||
attentions=encoder_outputs.attentions,
|
||||
)
|
File diff suppressed because it is too large
Load Diff
|
@ -0,0 +1,349 @@
|
|||
# --------------------------------------------------------
|
||||
# InternVL
|
||||
# Copyright (c) 2024 OpenGVLab
|
||||
# Licensed under The MIT License [see LICENSE for details]
|
||||
# --------------------------------------------------------
|
||||
|
||||
import warnings
|
||||
from typing import List, Optional, Tuple, Union
|
||||
|
||||
import torch.utils.checkpoint
|
||||
import transformers
|
||||
from torch import nn
|
||||
from torch.nn import CrossEntropyLoss
|
||||
from transformers import (AutoModel, GenerationConfig, LlamaForCausalLM,
|
||||
LlamaTokenizer)
|
||||
from transformers.modeling_outputs import CausalLMOutputWithPast
|
||||
from transformers.modeling_utils import PreTrainedModel
|
||||
from transformers.utils import ModelOutput, logging
|
||||
|
||||
from .configuration_internvl_chat import InternVLChatConfig
|
||||
from .conversation import get_conv_template
|
||||
from .modeling_intern_vit import InternVisionModel, has_flash_attn
|
||||
from .modeling_internlm2 import InternLM2ForCausalLM
|
||||
|
||||
logger = logging.get_logger(__name__)
|
||||
|
||||
|
||||
def version_cmp(v1, v2, op='eq'):
|
||||
import operator
|
||||
|
||||
from packaging import version
|
||||
op_func = getattr(operator, op)
|
||||
return op_func(version.parse(v1), version.parse(v2))
|
||||
|
||||
|
||||
class InternVLChatModel(PreTrainedModel):
|
||||
config_class = InternVLChatConfig
|
||||
main_input_name = 'pixel_values'
|
||||
base_model_prefix = 'language_model'
|
||||
_supports_flash_attn_2 = True
|
||||
_no_split_modules = ['InternVisionModel', 'LlamaDecoderLayer', 'InternLM2DecoderLayer']
|
||||
|
||||
def __init__(self, config: InternVLChatConfig, vision_model=None, language_model=None, use_flash_attn=True):
|
||||
super().__init__(config)
|
||||
|
||||
assert version_cmp(transformers.__version__, '4.37.0', 'ge')
|
||||
image_size = config.force_image_size or config.vision_config.image_size
|
||||
patch_size = config.vision_config.patch_size
|
||||
self.patch_size = patch_size
|
||||
self.select_layer = config.select_layer
|
||||
self.template = config.template
|
||||
self.num_image_token = int((image_size // patch_size) ** 2 * (config.downsample_ratio ** 2))
|
||||
self.downsample_ratio = config.downsample_ratio
|
||||
self.ps_version = config.ps_version
|
||||
use_flash_attn = use_flash_attn if has_flash_attn else False
|
||||
config.vision_config.use_flash_attn = True if use_flash_attn else False
|
||||
config.llm_config.attn_implementation = 'flash_attention_2' if use_flash_attn else 'eager'
|
||||
|
||||
logger.info(f'num_image_token: {self.num_image_token}')
|
||||
logger.info(f'ps_version: {self.ps_version}')
|
||||
if vision_model is not None:
|
||||
self.vision_model = vision_model
|
||||
else:
|
||||
self.vision_model = InternVisionModel(config.vision_config)
|
||||
if language_model is not None:
|
||||
self.language_model = language_model
|
||||
else:
|
||||
if config.llm_config.architectures[0] == 'LlamaForCausalLM':
|
||||
self.language_model = LlamaForCausalLM(config.llm_config)
|
||||
elif config.llm_config.architectures[0] == 'InternLM2ForCausalLM':
|
||||
self.language_model = InternLM2ForCausalLM(config.llm_config)
|
||||
else:
|
||||
raise NotImplementedError(f'{config.llm_config.architectures[0]} is not implemented.')
|
||||
|
||||
vit_hidden_size = config.vision_config.hidden_size
|
||||
llm_hidden_size = config.llm_config.hidden_size
|
||||
|
||||
self.mlp1 = nn.Sequential(
|
||||
nn.LayerNorm(vit_hidden_size * int(1 / self.downsample_ratio) ** 2),
|
||||
nn.Linear(vit_hidden_size * int(1 / self.downsample_ratio) ** 2, llm_hidden_size),
|
||||
nn.GELU(),
|
||||
nn.Linear(llm_hidden_size, llm_hidden_size)
|
||||
)
|
||||
|
||||
self.img_context_token_id = None
|
||||
self.conv_template = get_conv_template(self.template)
|
||||
self.system_message = self.conv_template.system_message
|
||||
|
||||
def forward(
|
||||
self,
|
||||
pixel_values: torch.FloatTensor,
|
||||
input_ids: torch.LongTensor = None,
|
||||
attention_mask: Optional[torch.Tensor] = None,
|
||||
position_ids: Optional[torch.LongTensor] = None,
|
||||
image_flags: Optional[torch.LongTensor] = None,
|
||||
past_key_values: Optional[List[torch.FloatTensor]] = None,
|
||||
labels: Optional[torch.LongTensor] = None,
|
||||
use_cache: Optional[bool] = None,
|
||||
output_attentions: Optional[bool] = None,
|
||||
output_hidden_states: Optional[bool] = None,
|
||||
return_dict: Optional[bool] = None,
|
||||
) -> Union[Tuple, CausalLMOutputWithPast]:
|
||||
return_dict = return_dict if return_dict is not None else self.config.use_return_dict
|
||||
|
||||
image_flags = image_flags.squeeze(-1)
|
||||
input_embeds = self.language_model.get_input_embeddings()(input_ids).clone()
|
||||
|
||||
vit_embeds = self.extract_feature(pixel_values)
|
||||
vit_embeds = vit_embeds[image_flags == 1]
|
||||
vit_batch_size = pixel_values.shape[0]
|
||||
|
||||
B, N, C = input_embeds.shape
|
||||
input_embeds = input_embeds.reshape(B * N, C)
|
||||
|
||||
if torch.distributed.is_initialized() and torch.distributed.get_rank() == 0:
|
||||
print(f'dynamic ViT batch size: {vit_batch_size}, images per sample: {vit_batch_size / B}, dynamic token length: {N}')
|
||||
|
||||
input_ids = input_ids.reshape(B * N)
|
||||
selected = (input_ids == self.img_context_token_id)
|
||||
try:
|
||||
input_embeds[selected] = input_embeds[selected] * 0.0 + vit_embeds.reshape(-1, C)
|
||||
except Exception as e:
|
||||
vit_embeds = vit_embeds.reshape(-1, C)
|
||||
print(f'warning: {e}, input_embeds[selected].shape={input_embeds[selected].shape}, '
|
||||
f'vit_embeds.shape={vit_embeds.shape}')
|
||||
n_token = selected.sum()
|
||||
input_embeds[selected] = input_embeds[selected] * 0.0 + vit_embeds[:n_token]
|
||||
|
||||
input_embeds = input_embeds.reshape(B, N, C)
|
||||
|
||||
outputs = self.language_model(
|
||||
inputs_embeds=input_embeds,
|
||||
attention_mask=attention_mask,
|
||||
position_ids=position_ids,
|
||||
past_key_values=past_key_values,
|
||||
use_cache=use_cache,
|
||||
output_attentions=output_attentions,
|
||||
output_hidden_states=output_hidden_states,
|
||||
return_dict=return_dict,
|
||||
)
|
||||
logits = outputs.logits
|
||||
|
||||
loss = None
|
||||
if labels is not None:
|
||||
# Shift so that tokens < n predict n
|
||||
shift_logits = logits[..., :-1, :].contiguous()
|
||||
shift_labels = labels[..., 1:].contiguous()
|
||||
# Flatten the tokens
|
||||
loss_fct = CrossEntropyLoss()
|
||||
shift_logits = shift_logits.view(-1, self.language_model.config.vocab_size)
|
||||
shift_labels = shift_labels.view(-1)
|
||||
# Enable model parallelism
|
||||
shift_labels = shift_labels.to(shift_logits.device)
|
||||
loss = loss_fct(shift_logits, shift_labels)
|
||||
|
||||
if not return_dict:
|
||||
output = (logits,) + outputs[1:]
|
||||
return (loss,) + output if loss is not None else output
|
||||
|
||||
return CausalLMOutputWithPast(
|
||||
loss=loss,
|
||||
logits=logits,
|
||||
past_key_values=outputs.past_key_values,
|
||||
hidden_states=outputs.hidden_states,
|
||||
attentions=outputs.attentions,
|
||||
)
|
||||
|
||||
def pixel_shuffle(self, x, scale_factor=0.5):
|
||||
n, w, h, c = x.size()
|
||||
# N, W, H, C --> N, W, H * scale, C // scale
|
||||
x = x.view(n, w, int(h * scale_factor), int(c / scale_factor))
|
||||
# N, W, H * scale, C // scale --> N, H * scale, W, C // scale
|
||||
x = x.permute(0, 2, 1, 3).contiguous()
|
||||
# N, H * scale, W, C // scale --> N, H * scale, W * scale, C // (scale ** 2)
|
||||
x = x.view(n, int(h * scale_factor), int(w * scale_factor),
|
||||
int(c / (scale_factor * scale_factor)))
|
||||
if self.ps_version == 'v1':
|
||||
warnings.warn("In ps_version 'v1', the height and width have not been swapped back, "
|
||||
'which results in a transposed image.')
|
||||
else:
|
||||
x = x.permute(0, 2, 1, 3).contiguous()
|
||||
return x
|
||||
|
||||
def extract_feature(self, pixel_values):
|
||||
if self.select_layer == -1:
|
||||
vit_embeds = self.vision_model(
|
||||
pixel_values=pixel_values,
|
||||
output_hidden_states=False,
|
||||
return_dict=True).last_hidden_state
|
||||
else:
|
||||
vit_embeds = self.vision_model(
|
||||
pixel_values=pixel_values,
|
||||
output_hidden_states=True,
|
||||
return_dict=True).hidden_states[self.select_layer]
|
||||
vit_embeds = vit_embeds[:, 1:, :]
|
||||
|
||||
h = w = int(vit_embeds.shape[1] ** 0.5)
|
||||
vit_embeds = vit_embeds.reshape(vit_embeds.shape[0], h, w, -1)
|
||||
vit_embeds = self.pixel_shuffle(vit_embeds, scale_factor=self.downsample_ratio)
|
||||
vit_embeds = vit_embeds.reshape(vit_embeds.shape[0], -1, vit_embeds.shape[-1])
|
||||
vit_embeds = self.mlp1(vit_embeds)
|
||||
return vit_embeds
|
||||
|
||||
def batch_chat(self, tokenizer, pixel_values, questions, generation_config, num_patches_list=None,
|
||||
history=None, return_history=False, IMG_START_TOKEN='<img>', IMG_END_TOKEN='</img>',
|
||||
IMG_CONTEXT_TOKEN='<IMG_CONTEXT>', verbose=False, image_counts=None):
|
||||
if history is not None or return_history:
|
||||
print('Now multi-turn chat is not supported in batch_chat.')
|
||||
raise NotImplementedError
|
||||
|
||||
if image_counts is not None:
|
||||
num_patches_list = image_counts
|
||||
print('Warning: `image_counts` is deprecated. Please use `num_patches_list` instead.')
|
||||
|
||||
img_context_token_id = tokenizer.convert_tokens_to_ids(IMG_CONTEXT_TOKEN)
|
||||
self.img_context_token_id = img_context_token_id
|
||||
|
||||
if verbose and pixel_values is not None:
|
||||
image_bs = pixel_values.shape[0]
|
||||
print(f'dynamic ViT batch size: {image_bs}')
|
||||
|
||||
queries = []
|
||||
for idx, num_patches in enumerate(num_patches_list):
|
||||
question = questions[idx]
|
||||
if pixel_values is not None and '<image>' not in question:
|
||||
question = '<image>\n' + question
|
||||
template = get_conv_template(self.template)
|
||||
template.system_message = self.system_message
|
||||
template.append_message(template.roles[0], question)
|
||||
template.append_message(template.roles[1], None)
|
||||
query = template.get_prompt()
|
||||
|
||||
image_tokens = IMG_START_TOKEN + IMG_CONTEXT_TOKEN * self.num_image_token * num_patches + IMG_END_TOKEN
|
||||
query = query.replace('<image>', image_tokens, 1)
|
||||
queries.append(query)
|
||||
|
||||
tokenizer.padding_side = 'left'
|
||||
model_inputs = tokenizer(queries, return_tensors='pt', padding=True)
|
||||
input_ids = model_inputs['input_ids'].to(self.device)
|
||||
attention_mask = model_inputs['attention_mask'].to(self.device)
|
||||
eos_token_id = tokenizer.convert_tokens_to_ids(template.sep.strip())
|
||||
generation_config['eos_token_id'] = eos_token_id
|
||||
generation_output = self.generate(
|
||||
pixel_values=pixel_values,
|
||||
input_ids=input_ids,
|
||||
attention_mask=attention_mask,
|
||||
**generation_config
|
||||
)
|
||||
responses = tokenizer.batch_decode(generation_output, skip_special_tokens=True)
|
||||
responses = [response.split(template.sep.strip())[0].strip() for response in responses]
|
||||
return responses
|
||||
|
||||
def chat(self, tokenizer, pixel_values, question, generation_config, history=None, return_history=False,
|
||||
num_patches_list=None, IMG_START_TOKEN='<img>', IMG_END_TOKEN='</img>', IMG_CONTEXT_TOKEN='<IMG_CONTEXT>',
|
||||
verbose=False):
|
||||
|
||||
if history is None and pixel_values is not None and '<image>' not in question:
|
||||
question = '<image>\n' + question
|
||||
|
||||
if num_patches_list is None:
|
||||
num_patches_list = [pixel_values.shape[0]] if pixel_values is not None else []
|
||||
assert pixel_values is None or len(pixel_values) == sum(num_patches_list)
|
||||
|
||||
img_context_token_id = tokenizer.convert_tokens_to_ids(IMG_CONTEXT_TOKEN)
|
||||
self.img_context_token_id = img_context_token_id
|
||||
|
||||
template = get_conv_template(self.template)
|
||||
template.system_message = self.system_message
|
||||
eos_token_id = tokenizer.convert_tokens_to_ids(template.sep.strip())
|
||||
|
||||
history = [] if history is None else history
|
||||
for (old_question, old_answer) in history:
|
||||
template.append_message(template.roles[0], old_question)
|
||||
template.append_message(template.roles[1], old_answer)
|
||||
template.append_message(template.roles[0], question)
|
||||
template.append_message(template.roles[1], None)
|
||||
query = template.get_prompt()
|
||||
|
||||
if verbose and pixel_values is not None:
|
||||
image_bs = pixel_values.shape[0]
|
||||
print(f'dynamic ViT batch size: {image_bs}')
|
||||
|
||||
for num_patches in num_patches_list:
|
||||
image_tokens = IMG_START_TOKEN + IMG_CONTEXT_TOKEN * self.num_image_token * num_patches + IMG_END_TOKEN
|
||||
query = query.replace('<image>', image_tokens, 1)
|
||||
|
||||
model_inputs = tokenizer(query, return_tensors='pt')
|
||||
input_ids = model_inputs['input_ids'].to(self.device)
|
||||
attention_mask = model_inputs['attention_mask'].to(self.device)
|
||||
generation_config['eos_token_id'] = eos_token_id
|
||||
generation_output = self.generate(
|
||||
pixel_values=pixel_values,
|
||||
input_ids=input_ids,
|
||||
attention_mask=attention_mask,
|
||||
**generation_config
|
||||
)
|
||||
response = tokenizer.batch_decode(generation_output, skip_special_tokens=True)[0]
|
||||
response = response.split(template.sep.strip())[0].strip()
|
||||
history.append((question, response))
|
||||
if return_history:
|
||||
return response, history
|
||||
else:
|
||||
query_to_print = query.replace(IMG_CONTEXT_TOKEN, '')
|
||||
query_to_print = query_to_print.replace(f'{IMG_START_TOKEN}{IMG_END_TOKEN}', '<image>')
|
||||
if verbose:
|
||||
print(query_to_print, response)
|
||||
return response
|
||||
|
||||
@torch.no_grad()
|
||||
def generate(
|
||||
self,
|
||||
pixel_values: Optional[torch.FloatTensor] = None,
|
||||
input_ids: Optional[torch.FloatTensor] = None,
|
||||
attention_mask: Optional[torch.LongTensor] = None,
|
||||
visual_features: Optional[torch.FloatTensor] = None,
|
||||
generation_config: Optional[GenerationConfig] = None,
|
||||
output_hidden_states: Optional[bool] = None,
|
||||
**generate_kwargs,
|
||||
) -> torch.LongTensor:
|
||||
|
||||
assert self.img_context_token_id is not None
|
||||
if pixel_values is not None:
|
||||
if visual_features is not None:
|
||||
vit_embeds = visual_features
|
||||
else:
|
||||
vit_embeds = self.extract_feature(pixel_values)
|
||||
input_embeds = self.language_model.get_input_embeddings()(input_ids)
|
||||
B, N, C = input_embeds.shape
|
||||
input_embeds = input_embeds.reshape(B * N, C)
|
||||
|
||||
input_ids = input_ids.reshape(B * N)
|
||||
selected = (input_ids == self.img_context_token_id)
|
||||
assert selected.sum() != 0
|
||||
input_embeds[selected] = vit_embeds.reshape(-1, C).to(input_embeds.device)
|
||||
|
||||
input_embeds = input_embeds.reshape(B, N, C)
|
||||
else:
|
||||
input_embeds = self.language_model.get_input_embeddings()(input_ids)
|
||||
|
||||
outputs = self.language_model.generate(
|
||||
inputs_embeds=input_embeds,
|
||||
attention_mask=attention_mask,
|
||||
generation_config=generation_config,
|
||||
output_hidden_states=output_hidden_states,
|
||||
use_cache=True,
|
||||
**generate_kwargs,
|
||||
)
|
||||
|
||||
return outputs
|
|
@ -0,0 +1,19 @@
|
|||
{
|
||||
"crop_size": 448,
|
||||
"do_center_crop": true,
|
||||
"do_normalize": true,
|
||||
"do_resize": true,
|
||||
"feature_extractor_type": "CLIPFeatureExtractor",
|
||||
"image_mean": [
|
||||
0.485,
|
||||
0.456,
|
||||
0.406
|
||||
],
|
||||
"image_std": [
|
||||
0.229,
|
||||
0.224,
|
||||
0.225
|
||||
],
|
||||
"resample": 3,
|
||||
"size": 448
|
||||
}
|
BIN
runs/Nov18_19-03-50_HOST-10-140-60-23/events.out.tfevents.1731928182.HOST-10-140-60-23.129254.0 (Stored with Git LFS)
Normal file
BIN
runs/Nov18_19-03-50_HOST-10-140-60-23/events.out.tfevents.1731928182.HOST-10-140-60-23.129254.0 (Stored with Git LFS)
Normal file
Binary file not shown.
|
@ -0,0 +1,47 @@
|
|||
{
|
||||
"additional_special_tokens": [
|
||||
"<|im_start|>",
|
||||
"<|im_end|>",
|
||||
"<|action_start|>",
|
||||
"<|action_end|>",
|
||||
"<|interpreter|>",
|
||||
"<|plugin|>",
|
||||
"<img>",
|
||||
"</img>",
|
||||
"<IMG_CONTEXT>",
|
||||
"<quad>",
|
||||
"</quad>",
|
||||
"<ref>",
|
||||
"</ref>",
|
||||
"<box>",
|
||||
"</box>"
|
||||
],
|
||||
"bos_token": {
|
||||
"content": "<s>",
|
||||
"lstrip": false,
|
||||
"normalized": false,
|
||||
"rstrip": false,
|
||||
"single_word": false
|
||||
},
|
||||
"eos_token": {
|
||||
"content": "</s>",
|
||||
"lstrip": false,
|
||||
"normalized": false,
|
||||
"rstrip": false,
|
||||
"single_word": false
|
||||
},
|
||||
"pad_token": {
|
||||
"content": "</s>",
|
||||
"lstrip": false,
|
||||
"normalized": false,
|
||||
"rstrip": false,
|
||||
"single_word": false
|
||||
},
|
||||
"unk_token": {
|
||||
"content": "<unk>",
|
||||
"lstrip": false,
|
||||
"normalized": false,
|
||||
"rstrip": false,
|
||||
"single_word": false
|
||||
}
|
||||
}
|
|
@ -0,0 +1,235 @@
|
|||
# Copyright (c) The InternLM team and The HuggingFace Inc. team. All rights reserved.
|
||||
#
|
||||
# This code is based on transformers/src/transformers/models/llama/tokenization_llama.py
|
||||
#
|
||||
# Licensed under the Apache License, Version 2.0 (the "License");
|
||||
# you may not use this file except in compliance with the License.
|
||||
# You may obtain a copy of the License at
|
||||
#
|
||||
# http://www.apache.org/licenses/LICENSE-2.0
|
||||
#
|
||||
# Unless required by applicable law or agreed to in writing, software
|
||||
# distributed under the License is distributed on an "AS IS" BASIS,
|
||||
# WITHOUT WARRANTIES OR CONDITIONS OF ANY KIND, either express or implied.
|
||||
# See the License for the specific language governing permissions and
|
||||
# limitations under the License.
|
||||
|
||||
"""Tokenization classes for InternLM."""
|
||||
import os
|
||||
from shutil import copyfile
|
||||
from typing import Any, Dict, List, Optional, Tuple
|
||||
|
||||
import sentencepiece as spm
|
||||
from transformers.tokenization_utils import PreTrainedTokenizer
|
||||
from transformers.utils import logging
|
||||
|
||||
logger = logging.get_logger(__name__)
|
||||
|
||||
VOCAB_FILES_NAMES = {'vocab_file': './tokenizer.model'}
|
||||
|
||||
PRETRAINED_VOCAB_FILES_MAP = {}
|
||||
|
||||
|
||||
# Modified from transformers.model.llama.tokenization_llama.LlamaTokenizer
|
||||
class InternLM2Tokenizer(PreTrainedTokenizer):
|
||||
"""
|
||||
Construct a InternLM2 tokenizer. Based on byte-level Byte-Pair-Encoding.
|
||||
|
||||
Args:
|
||||
vocab_file (`str`):
|
||||
Path to the vocabulary file.
|
||||
"""
|
||||
|
||||
vocab_files_names = VOCAB_FILES_NAMES
|
||||
pretrained_vocab_files_map = PRETRAINED_VOCAB_FILES_MAP
|
||||
model_input_names = ['input_ids', 'attention_mask']
|
||||
_auto_class = 'AutoTokenizer'
|
||||
|
||||
def __init__(
|
||||
self,
|
||||
vocab_file,
|
||||
unk_token='<unk>',
|
||||
bos_token='<s>',
|
||||
eos_token='</s>',
|
||||
pad_token='</s>',
|
||||
sp_model_kwargs: Optional[Dict[str, Any]] = None,
|
||||
add_bos_token=True,
|
||||
add_eos_token=False,
|
||||
decode_with_prefix_space=False,
|
||||
clean_up_tokenization_spaces=False,
|
||||
**kwargs,
|
||||
):
|
||||
self.sp_model_kwargs = {} if sp_model_kwargs is None else sp_model_kwargs
|
||||
self.vocab_file = vocab_file
|
||||
self.add_bos_token = add_bos_token
|
||||
self.add_eos_token = add_eos_token
|
||||
self.decode_with_prefix_space = decode_with_prefix_space
|
||||
self.sp_model = spm.SentencePieceProcessor(**self.sp_model_kwargs)
|
||||
self.sp_model.Load(vocab_file)
|
||||
self._no_prefix_space_tokens = None
|
||||
super().__init__(
|
||||
bos_token=bos_token,
|
||||
eos_token=eos_token,
|
||||
unk_token=unk_token,
|
||||
pad_token=pad_token,
|
||||
clean_up_tokenization_spaces=clean_up_tokenization_spaces,
|
||||
**kwargs,
|
||||
)
|
||||
|
||||
@property
|
||||
def no_prefix_space_tokens(self):
|
||||
if self._no_prefix_space_tokens is None:
|
||||
vocab = self.convert_ids_to_tokens(list(range(self.vocab_size)))
|
||||
self._no_prefix_space_tokens = {i for i, tok in enumerate(vocab) if not tok.startswith('▁')}
|
||||
return self._no_prefix_space_tokens
|
||||
|
||||
@property
|
||||
def vocab_size(self):
|
||||
"""Returns vocab size"""
|
||||
return self.sp_model.get_piece_size()
|
||||
|
||||
@property
|
||||
def bos_token_id(self) -> Optional[int]:
|
||||
return self.sp_model.bos_id()
|
||||
|
||||
@property
|
||||
def eos_token_id(self) -> Optional[int]:
|
||||
return self.sp_model.eos_id()
|
||||
|
||||
def get_vocab(self):
|
||||
"""Returns vocab as a dict"""
|
||||
vocab = {self.convert_ids_to_tokens(i): i for i in range(self.vocab_size)}
|
||||
vocab.update(self.added_tokens_encoder)
|
||||
return vocab
|
||||
|
||||
def _tokenize(self, text):
|
||||
"""Returns a tokenized string."""
|
||||
return self.sp_model.encode(text, out_type=str)
|
||||
|
||||
def _convert_token_to_id(self, token):
|
||||
"""Converts a token (str) in an id using the vocab."""
|
||||
return self.sp_model.piece_to_id(token)
|
||||
|
||||
def _convert_id_to_token(self, index):
|
||||
"""Converts an index (integer) in a token (str) using the vocab."""
|
||||
token = self.sp_model.IdToPiece(index)
|
||||
return token
|
||||
|
||||
def _maybe_add_prefix_space(self, tokens, decoded):
|
||||
if tokens and tokens[0] not in self.no_prefix_space_tokens:
|
||||
return ' ' + decoded
|
||||
else:
|
||||
return decoded
|
||||
|
||||
def convert_tokens_to_string(self, tokens):
|
||||
"""Converts a sequence of tokens (string) in a single string."""
|
||||
current_sub_tokens = []
|
||||
out_string = ''
|
||||
prev_is_special = False
|
||||
for token in tokens:
|
||||
# make sure that special tokens are not decoded using sentencepiece model
|
||||
if token in self.all_special_tokens:
|
||||
if not prev_is_special:
|
||||
out_string += ' '
|
||||
out_string += self.sp_model.decode(current_sub_tokens) + token
|
||||
prev_is_special = True
|
||||
current_sub_tokens = []
|
||||
else:
|
||||
current_sub_tokens.append(token)
|
||||
prev_is_special = False
|
||||
out_string += self.sp_model.decode(current_sub_tokens)
|
||||
out_string = self.clean_up_tokenization(out_string)
|
||||
out_string = self._maybe_add_prefix_space(tokens=tokens, decoded=out_string)
|
||||
return out_string[1:]
|
||||
|
||||
def save_vocabulary(self, save_directory, filename_prefix: Optional[str] = None) -> Tuple[str]:
|
||||
"""
|
||||
Save the vocabulary and special tokens file to a directory.
|
||||
|
||||
Args:
|
||||
save_directory (`str`):
|
||||
The directory in which to save the vocabulary.
|
||||
|
||||
Returns:
|
||||
`Tuple(str)`: Paths to the files saved.
|
||||
"""
|
||||
if not os.path.isdir(save_directory):
|
||||
logger.error(f'Vocabulary path ({save_directory}) should be a directory')
|
||||
return
|
||||
out_vocab_file = os.path.join(
|
||||
save_directory, (filename_prefix + '-' if filename_prefix else '') + VOCAB_FILES_NAMES['vocab_file']
|
||||
)
|
||||
|
||||
if os.path.abspath(self.vocab_file) != os.path.abspath(out_vocab_file) and os.path.isfile(self.vocab_file):
|
||||
copyfile(self.vocab_file, out_vocab_file)
|
||||
elif not os.path.isfile(self.vocab_file):
|
||||
with open(out_vocab_file, 'wb') as fi:
|
||||
content_spiece_model = self.sp_model.serialized_model_proto()
|
||||
fi.write(content_spiece_model)
|
||||
|
||||
return (out_vocab_file,)
|
||||
|
||||
def build_inputs_with_special_tokens(self, token_ids_0, token_ids_1=None):
|
||||
if self.add_bos_token:
|
||||
bos_token_ids = [self.bos_token_id]
|
||||
else:
|
||||
bos_token_ids = []
|
||||
|
||||
output = bos_token_ids + token_ids_0
|
||||
|
||||
if token_ids_1 is not None:
|
||||
output = output + token_ids_1
|
||||
|
||||
if self.add_eos_token:
|
||||
output = output + [self.eos_token_id]
|
||||
|
||||
return output
|
||||
|
||||
def get_special_tokens_mask(
|
||||
self, token_ids_0: List[int], token_ids_1: Optional[List[int]] = None, already_has_special_tokens: bool = False
|
||||
) -> List[int]:
|
||||
"""
|
||||
Retrieve sequence ids from a token list that has no special tokens added. This method is called when adding
|
||||
special tokens using the tokenizer `prepare_for_model` method.
|
||||
|
||||
Args:
|
||||
token_ids_0 (`List[int]`):
|
||||
List of IDs.
|
||||
token_ids_1 (`List[int]`, *optional*):
|
||||
Optional second list of IDs for sequence pairs.
|
||||
already_has_special_tokens (`bool`, *optional*, defaults to `False`):
|
||||
Whether or not the token list is already formatted with special tokens for the model.
|
||||
|
||||
Returns:
|
||||
`List[int]`: A list of integers in the range [0, 1]: 1 for a special token, 0 for a sequence token.
|
||||
"""
|
||||
if already_has_special_tokens:
|
||||
return super().get_special_tokens_mask(
|
||||
token_ids_0=token_ids_0, token_ids_1=token_ids_1, already_has_special_tokens=True
|
||||
)
|
||||
|
||||
if token_ids_1 is None:
|
||||
return [1] + ([0] * len(token_ids_0)) + [1]
|
||||
return [1] + ([0] * len(token_ids_0)) + [1, 1] + ([0] * len(token_ids_1)) + [1]
|
||||
|
||||
def create_token_type_ids_from_sequences(
|
||||
self, token_ids_0: List[int], token_ids_1: Optional[List[int]] = None
|
||||
) -> List[int]:
|
||||
"""
|
||||
Create a mask from the two sequences passed to be used in a sequence-pair classification task. T5 does not make
|
||||
use of token type ids, therefore a list of zeros is returned.
|
||||
|
||||
Args:
|
||||
token_ids_0 (`List[int]`):
|
||||
List of IDs.
|
||||
token_ids_1 (`List[int]`, *optional*):
|
||||
Optional second list of IDs for sequence pairs.
|
||||
|
||||
Returns:
|
||||
`List[int]`: List of zeros.
|
||||
"""
|
||||
eos = [self.eos_token_id]
|
||||
|
||||
if token_ids_1 is None:
|
||||
return len(token_ids_0 + eos) * [0]
|
||||
return len(token_ids_0 + eos + token_ids_1 + eos) * [0]
|
|
@ -0,0 +1,211 @@
|
|||
# Copyright (c) The InternLM team and The HuggingFace Inc. team. All rights reserved.
|
||||
#
|
||||
# This code is based on transformers/src/transformers/models/llama/tokenization_llama_fast.py
|
||||
#
|
||||
# Licensed under the Apache License, Version 2.0 (the "License");
|
||||
# you may not use this file except in compliance with the License.
|
||||
# You may obtain a copy of the License at
|
||||
#
|
||||
# http://www.apache.org/licenses/LICENSE-2.0
|
||||
#
|
||||
# Unless required by applicable law or agreed to in writing, software
|
||||
# distributed under the License is distributed on an "AS IS" BASIS,
|
||||
# WITHOUT WARRANTIES OR CONDITIONS OF ANY KIND, either express or implied.
|
||||
# See the License for the specific language governing permissions and
|
||||
# limitations under the License.
|
||||
|
||||
"""Tokenization Fast class for InternLM."""
|
||||
import os
|
||||
from shutil import copyfile
|
||||
from typing import Any, Dict, Optional, Tuple
|
||||
|
||||
from tokenizers import Tokenizer, decoders, normalizers, processors
|
||||
from tokenizers.models import BPE
|
||||
from transformers.convert_slow_tokenizer import (SLOW_TO_FAST_CONVERTERS,
|
||||
SentencePieceExtractor,
|
||||
SpmConverter)
|
||||
from transformers.tokenization_utils_fast import PreTrainedTokenizerFast
|
||||
from transformers.utils import logging
|
||||
|
||||
from .tokenization_internlm2 import InternLM2Tokenizer
|
||||
|
||||
logger = logging.get_logger(__name__)
|
||||
|
||||
VOCAB_FILES_NAMES = {'vocab_file': './tokenizer.model'}
|
||||
|
||||
|
||||
# Modified from transformers.convert_slow_tokenizer.LlamaConverter
|
||||
class InternLM2Converter(SpmConverter):
|
||||
handle_byte_fallback = True
|
||||
|
||||
def vocab(self, proto):
|
||||
vocab = [
|
||||
('<unk>', 0.0),
|
||||
('<s>', 0.0),
|
||||
('</s>', 0.0),
|
||||
]
|
||||
vocab += [(piece.piece, piece.score) for piece in proto.pieces[3:]]
|
||||
return vocab
|
||||
|
||||
def unk_id(self, proto):
|
||||
unk_id = 0
|
||||
return unk_id
|
||||
|
||||
def decoder(self, replacement, add_prefix_space):
|
||||
return decoders.Sequence(
|
||||
[
|
||||
decoders.Replace('▁', ' '),
|
||||
decoders.ByteFallback(),
|
||||
decoders.Fuse(),
|
||||
decoders.Strip(content=' ', left=1),
|
||||
]
|
||||
)
|
||||
|
||||
def tokenizer(self, proto):
|
||||
model_type = proto.trainer_spec.model_type
|
||||
vocab_scores = self.vocab(proto)
|
||||
# special tokens
|
||||
added_tokens = self.original_tokenizer.added_tokens_decoder
|
||||
for i in range(len(vocab_scores)):
|
||||
piece, score = vocab_scores[i]
|
||||
if i in added_tokens:
|
||||
vocab_scores[i] = (added_tokens[i].content, score)
|
||||
if model_type == 1:
|
||||
raise RuntimeError('InternLM2 is supposed to be a BPE model!')
|
||||
|
||||
elif model_type == 2:
|
||||
_, merges = SentencePieceExtractor(self.original_tokenizer.vocab_file).extract(vocab_scores)
|
||||
bpe_vocab = {word: i for i, (word, _score) in enumerate(vocab_scores)}
|
||||
tokenizer = Tokenizer(
|
||||
BPE(bpe_vocab, merges, unk_token=proto.trainer_spec.unk_piece, fuse_unk=True, byte_fallback=True)
|
||||
)
|
||||
tokenizer.add_special_tokens(
|
||||
[ added_token for index, added_token in added_tokens.items()]
|
||||
)
|
||||
else:
|
||||
raise Exception(
|
||||
"You're trying to run a `Unigram` model but you're file was trained with a different algorithm"
|
||||
)
|
||||
|
||||
return tokenizer
|
||||
|
||||
def normalizer(self, proto):
|
||||
normalizers_list = []
|
||||
if proto.normalizer_spec.add_dummy_prefix:
|
||||
normalizers_list.append(normalizers.Prepend(prepend='▁'))
|
||||
normalizers_list.append(normalizers.Replace(pattern=' ', content='▁'))
|
||||
return normalizers.Sequence(normalizers_list)
|
||||
|
||||
def pre_tokenizer(self, replacement, add_prefix_space):
|
||||
return None
|
||||
|
||||
|
||||
SLOW_TO_FAST_CONVERTERS['InternLM2Tokenizer'] = InternLM2Converter
|
||||
|
||||
|
||||
# Modified from transformers.model.llama.tokenization_llama_fast.LlamaTokenizerFast -> InternLM2TokenizerFast
|
||||
class InternLM2TokenizerFast(PreTrainedTokenizerFast):
|
||||
vocab_files_names = VOCAB_FILES_NAMES
|
||||
slow_tokenizer_class = InternLM2Tokenizer
|
||||
padding_side = 'left'
|
||||
model_input_names = ['input_ids', 'attention_mask']
|
||||
_auto_class = 'AutoTokenizer'
|
||||
|
||||
def __init__(
|
||||
self,
|
||||
vocab_file,
|
||||
unk_token='<unk>',
|
||||
bos_token='<s>',
|
||||
eos_token='</s>',
|
||||
pad_token='</s>',
|
||||
sp_model_kwargs: Optional[Dict[str, Any]] = None,
|
||||
add_bos_token=True,
|
||||
add_eos_token=False,
|
||||
decode_with_prefix_space=False,
|
||||
clean_up_tokenization_spaces=False,
|
||||
**kwargs,
|
||||
):
|
||||
super().__init__(
|
||||
vocab_file=vocab_file,
|
||||
unk_token=unk_token,
|
||||
bos_token=bos_token,
|
||||
eos_token=eos_token,
|
||||
pad_token=pad_token,
|
||||
sp_model_kwargs=sp_model_kwargs,
|
||||
add_bos_token=add_bos_token,
|
||||
add_eos_token=add_eos_token,
|
||||
decode_with_prefix_space=decode_with_prefix_space,
|
||||
clean_up_tokenization_spaces=clean_up_tokenization_spaces,
|
||||
**kwargs,
|
||||
)
|
||||
self._add_bos_token = add_bos_token
|
||||
self._add_eos_token = add_eos_token
|
||||
self.update_post_processor()
|
||||
self.vocab_file = vocab_file
|
||||
|
||||
@property
|
||||
def can_save_slow_tokenizer(self) -> bool:
|
||||
return os.path.isfile(self.vocab_file) if self.vocab_file else False
|
||||
|
||||
def update_post_processor(self):
|
||||
"""
|
||||
Updates the underlying post processor with the current `bos_token` and `eos_token`.
|
||||
"""
|
||||
bos = self.bos_token
|
||||
bos_token_id = self.bos_token_id
|
||||
if bos is None and self.add_bos_token:
|
||||
raise ValueError('add_bos_token = True but bos_token = None')
|
||||
|
||||
eos = self.eos_token
|
||||
eos_token_id = self.eos_token_id
|
||||
if eos is None and self.add_eos_token:
|
||||
raise ValueError('add_eos_token = True but eos_token = None')
|
||||
|
||||
single = f"{(bos+':0 ') if self.add_bos_token else ''}$A:0{(' '+eos+':0') if self.add_eos_token else ''}"
|
||||
pair = f"{single}{(' '+bos+':1') if self.add_bos_token else ''} $B:1{(' '+eos+':1') if self.add_eos_token else ''}"
|
||||
|
||||
special_tokens = []
|
||||
if self.add_bos_token:
|
||||
special_tokens.append((bos, bos_token_id))
|
||||
if self.add_eos_token:
|
||||
special_tokens.append((eos, eos_token_id))
|
||||
self._tokenizer.post_processor = processors.TemplateProcessing(
|
||||
single=single, pair=pair, special_tokens=special_tokens
|
||||
)
|
||||
|
||||
@property
|
||||
def add_eos_token(self):
|
||||
return self._add_eos_token
|
||||
|
||||
@property
|
||||
def add_bos_token(self):
|
||||
return self._add_bos_token
|
||||
|
||||
@add_eos_token.setter
|
||||
def add_eos_token(self, value):
|
||||
self._add_eos_token = value
|
||||
self.update_post_processor()
|
||||
|
||||
@add_bos_token.setter
|
||||
def add_bos_token(self, value):
|
||||
self._add_bos_token = value
|
||||
self.update_post_processor()
|
||||
|
||||
def save_vocabulary(self, save_directory: str, filename_prefix: Optional[str] = None) -> Tuple[str]:
|
||||
if not self.can_save_slow_tokenizer:
|
||||
raise ValueError(
|
||||
'Your fast tokenizer does not have the necessary information to save the vocabulary for a slow '
|
||||
'tokenizer.'
|
||||
)
|
||||
|
||||
if not os.path.isdir(save_directory):
|
||||
logger.error(f'Vocabulary path ({save_directory}) should be a directory')
|
||||
return
|
||||
out_vocab_file = os.path.join(
|
||||
save_directory, (filename_prefix + '-' if filename_prefix else '') + VOCAB_FILES_NAMES['vocab_file']
|
||||
)
|
||||
|
||||
if os.path.abspath(self.vocab_file) != os.path.abspath(out_vocab_file):
|
||||
copyfile(self.vocab_file, out_vocab_file)
|
||||
|
||||
return (out_vocab_file,)
|
Binary file not shown.
|
@ -0,0 +1,179 @@
|
|||
{
|
||||
"added_tokens_decoder": {
|
||||
"0": {
|
||||
"content": "<unk>",
|
||||
"lstrip": false,
|
||||
"normalized": false,
|
||||
"rstrip": false,
|
||||
"single_word": false,
|
||||
"special": true
|
||||
},
|
||||
"1": {
|
||||
"content": "<s>",
|
||||
"lstrip": false,
|
||||
"normalized": false,
|
||||
"rstrip": false,
|
||||
"single_word": false,
|
||||
"special": true
|
||||
},
|
||||
"2": {
|
||||
"content": "</s>",
|
||||
"lstrip": false,
|
||||
"normalized": false,
|
||||
"rstrip": false,
|
||||
"single_word": false,
|
||||
"special": true
|
||||
},
|
||||
"92538": {
|
||||
"content": "<|plugin|>",
|
||||
"lstrip": false,
|
||||
"normalized": false,
|
||||
"rstrip": false,
|
||||
"single_word": false,
|
||||
"special": true
|
||||
},
|
||||
"92539": {
|
||||
"content": "<|interpreter|>",
|
||||
"lstrip": false,
|
||||
"normalized": false,
|
||||
"rstrip": false,
|
||||
"single_word": false,
|
||||
"special": true
|
||||
},
|
||||
"92540": {
|
||||
"content": "<|action_end|>",
|
||||
"lstrip": false,
|
||||
"normalized": false,
|
||||
"rstrip": false,
|
||||
"single_word": false,
|
||||
"special": true
|
||||
},
|
||||
"92541": {
|
||||
"content": "<|action_start|>",
|
||||
"lstrip": false,
|
||||
"normalized": false,
|
||||
"rstrip": false,
|
||||
"single_word": false,
|
||||
"special": true
|
||||
},
|
||||
"92542": {
|
||||
"content": "<|im_end|>",
|
||||
"lstrip": false,
|
||||
"normalized": false,
|
||||
"rstrip": false,
|
||||
"single_word": false,
|
||||
"special": true
|
||||
},
|
||||
"92543": {
|
||||
"content": "<|im_start|>",
|
||||
"lstrip": false,
|
||||
"normalized": false,
|
||||
"rstrip": false,
|
||||
"single_word": false,
|
||||
"special": true
|
||||
},
|
||||
"92544": {
|
||||
"content": "<img>",
|
||||
"lstrip": false,
|
||||
"normalized": false,
|
||||
"rstrip": false,
|
||||
"single_word": false,
|
||||
"special": true
|
||||
},
|
||||
"92545": {
|
||||
"content": "</img>",
|
||||
"lstrip": false,
|
||||
"normalized": false,
|
||||
"rstrip": false,
|
||||
"single_word": false,
|
||||
"special": true
|
||||
},
|
||||
"92546": {
|
||||
"content": "<IMG_CONTEXT>",
|
||||
"lstrip": false,
|
||||
"normalized": false,
|
||||
"rstrip": false,
|
||||
"single_word": false,
|
||||
"special": true
|
||||
},
|
||||
"92547": {
|
||||
"content": "<quad>",
|
||||
"lstrip": false,
|
||||
"normalized": false,
|
||||
"rstrip": false,
|
||||
"single_word": false,
|
||||
"special": true
|
||||
},
|
||||
"92548": {
|
||||
"content": "</quad>",
|
||||
"lstrip": false,
|
||||
"normalized": false,
|
||||
"rstrip": false,
|
||||
"single_word": false,
|
||||
"special": true
|
||||
},
|
||||
"92549": {
|
||||
"content": "<ref>",
|
||||
"lstrip": false,
|
||||
"normalized": false,
|
||||
"rstrip": false,
|
||||
"single_word": false,
|
||||
"special": true
|
||||
},
|
||||
"92550": {
|
||||
"content": "</ref>",
|
||||
"lstrip": false,
|
||||
"normalized": false,
|
||||
"rstrip": false,
|
||||
"single_word": false,
|
||||
"special": true
|
||||
},
|
||||
"92551": {
|
||||
"content": "<box>",
|
||||
"lstrip": false,
|
||||
"normalized": false,
|
||||
"rstrip": false,
|
||||
"single_word": false,
|
||||
"special": true
|
||||
},
|
||||
"92552": {
|
||||
"content": "</box>",
|
||||
"lstrip": false,
|
||||
"normalized": false,
|
||||
"rstrip": false,
|
||||
"single_word": false,
|
||||
"special": true
|
||||
}
|
||||
},
|
||||
"additional_special_tokens": [
|
||||
"<|im_start|>",
|
||||
"<|im_end|>",
|
||||
"<|action_start|>",
|
||||
"<|action_end|>",
|
||||
"<|interpreter|>",
|
||||
"<|plugin|>",
|
||||
"<img>",
|
||||
"</img>",
|
||||
"<IMG_CONTEXT>",
|
||||
"<quad>",
|
||||
"</quad>",
|
||||
"<ref>",
|
||||
"</ref>",
|
||||
"<box>",
|
||||
"</box>"
|
||||
],
|
||||
"auto_map": {
|
||||
"AutoTokenizer": [
|
||||
"tokenization_internlm2.InternLM2Tokenizer",
|
||||
null
|
||||
]
|
||||
},
|
||||
"bos_token": "<s>",
|
||||
"chat_template": "{{ bos_token }}{% for message in messages %}{{'<|im_start|>' + message['role'] + '\n' + message['content'] + '<|im_end|>' + '\n'}}{% endfor %}{% if add_generation_prompt %}{{ '<|im_start|>assistant\n' }}{% endif %}",
|
||||
"clean_up_tokenization_spaces": false,
|
||||
"eos_token": "</s>",
|
||||
"model_max_length": 16384,
|
||||
"pad_token": "</s>",
|
||||
"tokenizer_class": "InternLM2Tokenizer",
|
||||
"unk_token": "<unk>"
|
||||
}
|
Loading…
Reference in New Issue