first commit
This commit is contained in:
commit
6d6c4736b0
|
@ -0,0 +1,14 @@
|
|||
Copyright (C) 2025 AIDC-AI
|
||||
Licensed under the Apache License, Version 2.0 (the "License");
|
||||
you may not use this file except in compliance with the License.
|
||||
You may obtain a copy of the License at
|
||||
http://www.apache.org/licenses/LICENSE-2.0
|
||||
Unless required by applicable law or agreed to in writing, software
|
||||
distributed under the License is distributed on an "AS IS" BASIS,
|
||||
WITHOUT WARRANTIES OR CONDITIONS OF ANY KIND, either express or implied.
|
||||
See the License for the specific language governing permissions and
|
||||
limitations under the License.
|
||||
|
||||
This model was trained based on the following models:
|
||||
1. Qwen2.5 (https://huggingface.co/Qwen/Qwen2.5-3B-Instruct), license:(https://huggingface.co/Qwen/Qwen2.5-3B-Instruct/blob/main/LICENSE, SPDX-License-identifier: Apache-2.0).
|
||||
2. AimV2 (https://huggingface.co/apple/aimv2-huge-patch14-448), license: Apple-Sample-Code-License (https://developer.apple.com/support/downloads/terms/apple-sample-code/Apple-Sample-Code-License.pdf)
|
|
@ -0,0 +1,258 @@
|
|||
---
|
||||
license: apache-2.0
|
||||
datasets:
|
||||
- AIDC-AI/Ovis-dataset
|
||||
library_name: transformers
|
||||
tags:
|
||||
- MLLM
|
||||
pipeline_tag: image-text-to-text
|
||||
language:
|
||||
- en
|
||||
- zh
|
||||
---
|
||||
|
||||
# Ovis2-4B
|
||||
<div align="center">
|
||||
<img src=https://cdn-uploads.huggingface.co/production/uploads/637aebed7ce76c3b834cea37/3IK823BZ8w-mz_QfeYkDn.png width="30%"/>
|
||||
</div>
|
||||
|
||||
## Introduction
|
||||
[GitHub](https://github.com/AIDC-AI/Ovis) | [Paper](https://arxiv.org/abs/2405.20797)
|
||||
|
||||
We are pleased to announce the release of **Ovis2**, our latest advancement in multi-modal large language models (MLLMs). Ovis2 inherits the innovative architectural design of the Ovis series, aimed at structurally aligning visual and textual embeddings. As the successor to Ovis1.6, Ovis2 incorporates significant improvements in both dataset curation and training methodologies.
|
||||
|
||||
**Key Features**:
|
||||
|
||||
- **Small Model Performance**: Optimized training strategies enable small-scale models to achieve higher capability density, demonstrating cross-tier leading advantages.
|
||||
|
||||
- **Enhanced Reasoning Capabilities**: Significantly strengthens Chain-of-Thought (CoT) reasoning abilities through the combination of instruction tuning and preference learning.
|
||||
|
||||
- **Video and Multi-Image Processing**: Video and multi-image data are incorporated into training to enhance the ability to handle complex visual information across frames and images.
|
||||
|
||||
- **Multilingual Support and OCR**: Enhances multilingual OCR beyond English and Chinese and improves structured data extraction from complex visual elements like tables and charts.
|
||||
|
||||
<div align="center">
|
||||
<img src="https://cdn-uploads.huggingface.co/production/uploads/637aebed7ce76c3b834cea37/XB-vgzDL6FshrSNGyZvzc.png" width="100%" />
|
||||
</div>
|
||||
|
||||
## Model Zoo
|
||||
|
||||
| Ovis MLLMs | ViT | LLM | Model Weights | Demo |
|
||||
|:-----------|:-----------------------:|:---------------------:|:-------------------------------------------------------:|:--------------------------------------------------------:|
|
||||
| Ovis2-1B | aimv2-large-patch14-448 | Qwen2.5-0.5B-Instruct | [Huggingface](https://huggingface.co/AIDC-AI/Ovis2-1B) | [Space](https://huggingface.co/spaces/AIDC-AI/Ovis2-1B) |
|
||||
| Ovis2-2B | aimv2-large-patch14-448 | Qwen2.5-1.5B-Instruct | [Huggingface](https://huggingface.co/AIDC-AI/Ovis2-2B) | [Space](https://huggingface.co/spaces/AIDC-AI/Ovis2-2B) |
|
||||
| Ovis2-4B | aimv2-huge-patch14-448 | Qwen2.5-3B-Instruct | [Huggingface](https://huggingface.co/AIDC-AI/Ovis2-4B) | [Space](https://huggingface.co/spaces/AIDC-AI/Ovis2-4B) |
|
||||
| Ovis2-8B | aimv2-huge-patch14-448 | Qwen2.5-7B-Instruct | [Huggingface](https://huggingface.co/AIDC-AI/Ovis2-8B) | [Space](https://huggingface.co/spaces/AIDC-AI/Ovis2-8B) |
|
||||
| Ovis2-16B | aimv2-huge-patch14-448 | Qwen2.5-14B-Instruct | [Huggingface](https://huggingface.co/AIDC-AI/Ovis2-16B) | [Space](https://huggingface.co/spaces/AIDC-AI/Ovis2-16B) |
|
||||
| Ovis2-34B | aimv2-1B-patch14-448 | Qwen2.5-32B-Instruct | [Huggingface](https://huggingface.co/AIDC-AI/Ovis2-34B) | - |
|
||||
|
||||
## Performance
|
||||
We use [VLMEvalKit](https://github.com/open-compass/VLMEvalKit), as employed in the OpenCompass [multimodal](https://rank.opencompass.org.cn/leaderboard-multimodal) and [reasoning](https://rank.opencompass.org.cn/leaderboard-multimodal-reasoning) leaderboard, to evaluate Ovis2.
|
||||
|
||||
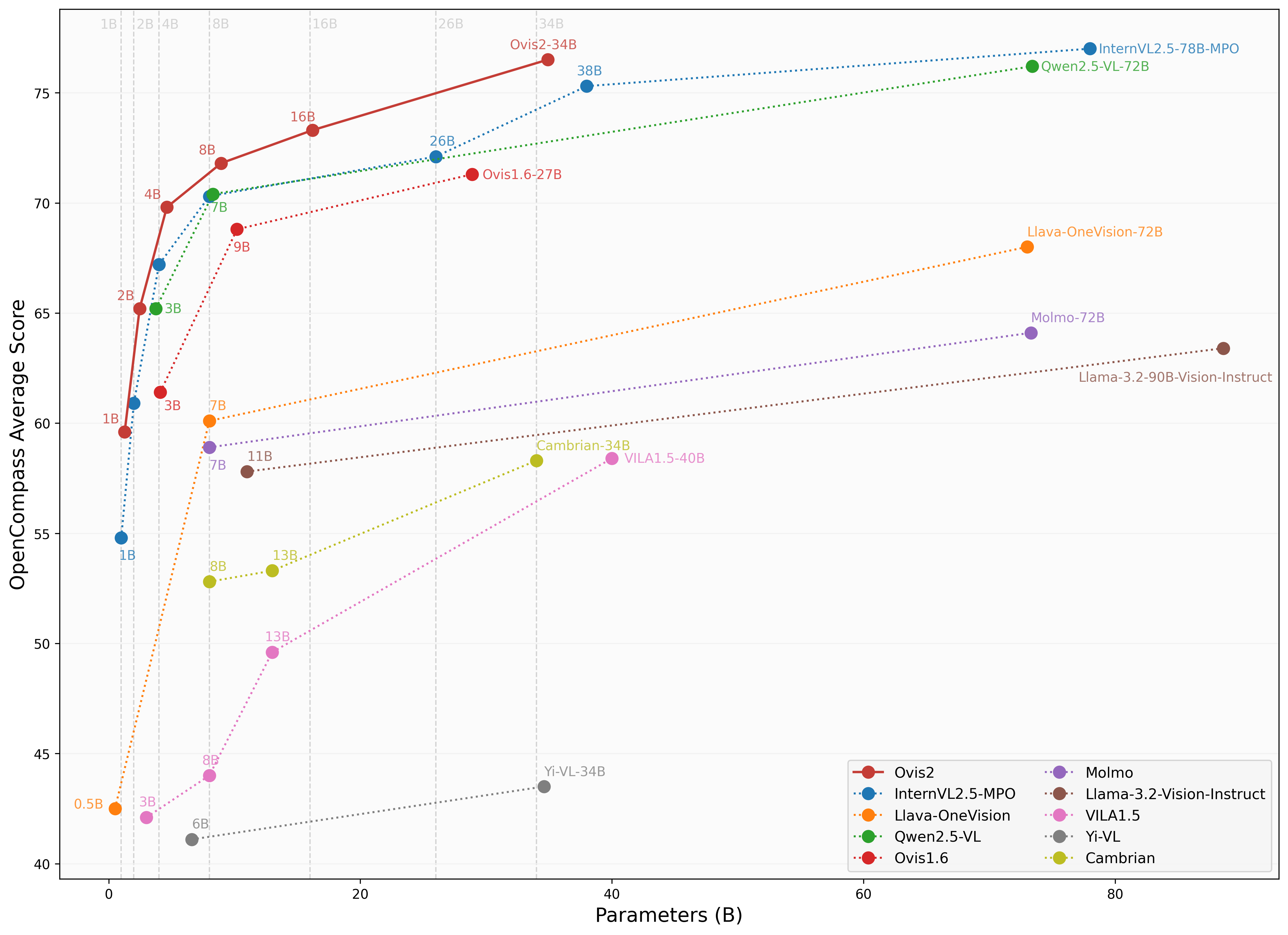
|
||||
|
||||
### Image Benchmark
|
||||
| Benchmark | Qwen2.5-VL-7B | InternVL2.5-8B-MPO | MiniCPM-o-2.6 | Ovis1.6-9B | InternVL2.5-4B-MPO | Ovis2-4B | Ovis2-8B |
|
||||
|:-----------------------------|:---------------:|:--------------------:|:---------------:|:------------:|:--------------------:|:----------:|:----------:|
|
||||
| MMBench-V1.1<sub>test</sub> | 82.6 | 82.0 | 80.6 | 80.5 | 77.8 | 81.4 | **83.6** |
|
||||
| MMStar | 64.1 | **65.2** | 63.3 | 62.9 | 61 | 61.9 | 64.6 |
|
||||
| MMMU<sub>val</sub> | 56.2 | 54.8 | 50.9 | 55 | 51.8 | 49.0 | **57.4** |
|
||||
| MathVista<sub>testmini</sub> | 65.8 | 67.9 | **73.3** | 67.3 | 64.1 | 69.6 | 71.8 |
|
||||
| HallusionBench | **56.3** | 51.7 | 51.1 | 52.2 | 47.5 | 53.8 | **56.3** |
|
||||
| AI2D | 84.1 | 84.5 | 86.1 | 84.4 | 81.5 | 85.7 | **86.6** |
|
||||
| OCRBench | 87.7 | 88.2 | 88.9 | 83 | 87.9 | **91.1** | 89.1 |
|
||||
| MMVet | 66.6 | **68.1** | 67.2 | 65 | 66 | 65.5 | 65.1 |
|
||||
| MMBench<sub>test</sub> | 83.4 | 83.2 | 83.2 | 82.7 | 79.6 | 83.2 | **84.9** |
|
||||
| MMT-Bench<sub>val</sub> | 62.7 | 62.5 | 62.3 | 64.9 | 61.6 | 65.2 | **66.6** |
|
||||
| RealWorldQA | 68.8 | 71.1 | 68.0 | 70.7 | 64.4 | 71.1 | **72.5** |
|
||||
| BLINK | 56.1 | **56.6** | 53.9 | 48.5 | 50.6 | 53.0 | 54.3 |
|
||||
| QBench | 77.9 | 73.8 | 78.7 | 76.7 | 71.5 | 78.1 | **78.9** |
|
||||
| ABench | 75.6 | 77.0 | **77.5** | 74.4 | 75.9 | **77.5** | 76.4 |
|
||||
| MTVQA | 28.5 | 27.2 | 23.1 | 19.2 | 28 | 29.4 | **29.7** |
|
||||
|
||||
### Video Benchmark
|
||||
| Benchmark | Qwen2.5-VL-7B | InternVL2.5-8B | LLaVA-OV-7B | InternVL2.5-4B | Ovis2-4B | Ovis2-8B |
|
||||
|:--------------------|:-------------:|:--------------:|:------------------:|:--------------:|:---------:|:-------------:|
|
||||
| VideoMME(wo/w-subs) | 65.1/71.6 | 64.2 / 66.9 | 58.2/61.5 | 62.3 / 63.6 | 64.0/66.3 | **68.0/71.6** |
|
||||
| MVBench | 69.6 | **72.0** | 56.7 | 71.6 | 68.45 | 68.15 |
|
||||
| MLVU(M-Avg/G-Avg) | 70.2/- | 68.9/- | 64.7/- | 68.3/- | 70.8/4.23 | **76.4**/4.25 |
|
||||
| MMBench-Video | 1.79 | 1.68 | - | 1.73 | 1.69 | **1.85** |
|
||||
| TempCompass | **71.7** | - | - | - | 67.02 | 69.28 |
|
||||
|
||||
## Usage
|
||||
Below is a code snippet demonstrating how to run Ovis with various input types. For additional usage instructions, including inference wrapper and Gradio UI, please refer to [Ovis GitHub](https://github.com/AIDC-AI/Ovis?tab=readme-ov-file#inference).
|
||||
```bash
|
||||
pip install torch==2.4.0 transformers==4.46.2 numpy==1.25.0 pillow==10.3.0
|
||||
pip install flash-attn==2.7.0.post2 --no-build-isolation
|
||||
```
|
||||
```python
|
||||
import torch
|
||||
from PIL import Image
|
||||
from transformers import AutoModelForCausalLM
|
||||
|
||||
# load model
|
||||
model = AutoModelForCausalLM.from_pretrained("AIDC-AI/Ovis2-4B",
|
||||
torch_dtype=torch.bfloat16,
|
||||
multimodal_max_length=32768,
|
||||
trust_remote_code=True).cuda()
|
||||
text_tokenizer = model.get_text_tokenizer()
|
||||
visual_tokenizer = model.get_visual_tokenizer()
|
||||
|
||||
# single-image input
|
||||
image_path = '/data/images/example_1.jpg'
|
||||
images = [Image.open(image_path)]
|
||||
max_partition = 9
|
||||
text = 'Describe the image.'
|
||||
query = f'<image>\n{text}'
|
||||
|
||||
## cot-style input
|
||||
# cot_suffix = "Provide a step-by-step solution to the problem, and conclude with 'the answer is' followed by the final solution."
|
||||
# image_path = '/data/images/example_1.jpg'
|
||||
# images = [Image.open(image_path)]
|
||||
# max_partition = 9
|
||||
# text = "What's the area of the shape?"
|
||||
# query = f'<image>\n{text}\n{cot_suffix}'
|
||||
|
||||
## multiple-images input
|
||||
# image_paths = [
|
||||
# '/data/images/example_1.jpg',
|
||||
# '/data/images/example_2.jpg',
|
||||
# '/data/images/example_3.jpg'
|
||||
# ]
|
||||
# images = [Image.open(image_path) for image_path in image_paths]
|
||||
# max_partition = 4
|
||||
# text = 'Describe each image.'
|
||||
# query = '\n'.join([f'Image {i+1}: <image>' for i in range(len(images))]) + '\n' + text
|
||||
|
||||
## video input (require `pip install moviepy==1.0.3`)
|
||||
# from moviepy.editor import VideoFileClip
|
||||
# video_path = '/data/videos/example_1.mp4'
|
||||
# num_frames = 12
|
||||
# max_partition = 1
|
||||
# text = 'Describe the video.'
|
||||
# with VideoFileClip(video_path) as clip:
|
||||
# total_frames = int(clip.fps * clip.duration)
|
||||
# if total_frames <= num_frames:
|
||||
# sampled_indices = range(total_frames)
|
||||
# else:
|
||||
# stride = total_frames / num_frames
|
||||
# sampled_indices = [min(total_frames - 1, int((stride * i + stride * (i + 1)) / 2)) for i in range(num_frames)]
|
||||
# frames = [clip.get_frame(index / clip.fps) for index in sampled_indices]
|
||||
# frames = [Image.fromarray(frame, mode='RGB') for frame in frames]
|
||||
# images = frames
|
||||
# query = '\n'.join(['<image>'] * len(images)) + '\n' + text
|
||||
|
||||
## text-only input
|
||||
# images = []
|
||||
# max_partition = None
|
||||
# text = 'Hello'
|
||||
# query = text
|
||||
|
||||
# format conversation
|
||||
prompt, input_ids, pixel_values = model.preprocess_inputs(query, images, max_partition=max_partition)
|
||||
attention_mask = torch.ne(input_ids, text_tokenizer.pad_token_id)
|
||||
input_ids = input_ids.unsqueeze(0).to(device=model.device)
|
||||
attention_mask = attention_mask.unsqueeze(0).to(device=model.device)
|
||||
if pixel_values is not None:
|
||||
pixel_values = pixel_values.to(dtype=visual_tokenizer.dtype, device=visual_tokenizer.device)
|
||||
pixel_values = [pixel_values]
|
||||
|
||||
# generate output
|
||||
with torch.inference_mode():
|
||||
gen_kwargs = dict(
|
||||
max_new_tokens=1024,
|
||||
do_sample=False,
|
||||
top_p=None,
|
||||
top_k=None,
|
||||
temperature=None,
|
||||
repetition_penalty=None,
|
||||
eos_token_id=model.generation_config.eos_token_id,
|
||||
pad_token_id=text_tokenizer.pad_token_id,
|
||||
use_cache=True
|
||||
)
|
||||
output_ids = model.generate(input_ids, pixel_values=pixel_values, attention_mask=attention_mask, **gen_kwargs)[0]
|
||||
output = text_tokenizer.decode(output_ids, skip_special_tokens=True)
|
||||
print(f'Output:\n{output}')
|
||||
```
|
||||
|
||||
<details>
|
||||
<summary>Batch Inference</summary>
|
||||
|
||||
```python
|
||||
import torch
|
||||
from PIL import Image
|
||||
from transformers import AutoModelForCausalLM
|
||||
|
||||
# load model
|
||||
model = AutoModelForCausalLM.from_pretrained("AIDC-AI/Ovis2-4B",
|
||||
torch_dtype=torch.bfloat16,
|
||||
multimodal_max_length=32768,
|
||||
trust_remote_code=True).cuda()
|
||||
text_tokenizer = model.get_text_tokenizer()
|
||||
visual_tokenizer = model.get_visual_tokenizer()
|
||||
|
||||
# preprocess inputs
|
||||
batch_inputs = [
|
||||
('/data/images/example_1.jpg', 'What colors dominate the image?'),
|
||||
('/data/images/example_2.jpg', 'What objects are depicted in this image?'),
|
||||
('/data/images/example_3.jpg', 'Is there any text in the image?')
|
||||
]
|
||||
|
||||
batch_input_ids = []
|
||||
batch_attention_mask = []
|
||||
batch_pixel_values = []
|
||||
|
||||
for image_path, text in batch_inputs:
|
||||
image = Image.open(image_path)
|
||||
query = f'<image>\n{text}'
|
||||
prompt, input_ids, pixel_values = model.preprocess_inputs(query, [image], max_partition=9)
|
||||
attention_mask = torch.ne(input_ids, text_tokenizer.pad_token_id)
|
||||
batch_input_ids.append(input_ids.to(device=model.device))
|
||||
batch_attention_mask.append(attention_mask.to(device=model.device))
|
||||
batch_pixel_values.append(pixel_values.to(dtype=visual_tokenizer.dtype, device=visual_tokenizer.device))
|
||||
|
||||
batch_input_ids = torch.nn.utils.rnn.pad_sequence([i.flip(dims=[0]) for i in batch_input_ids], batch_first=True,
|
||||
padding_value=0.0).flip(dims=[1])
|
||||
batch_input_ids = batch_input_ids[:, -model.config.multimodal_max_length:]
|
||||
batch_attention_mask = torch.nn.utils.rnn.pad_sequence([i.flip(dims=[0]) for i in batch_attention_mask],
|
||||
batch_first=True, padding_value=False).flip(dims=[1])
|
||||
batch_attention_mask = batch_attention_mask[:, -model.config.multimodal_max_length:]
|
||||
|
||||
# generate outputs
|
||||
with torch.inference_mode():
|
||||
gen_kwargs = dict(
|
||||
max_new_tokens=1024,
|
||||
do_sample=False,
|
||||
top_p=None,
|
||||
top_k=None,
|
||||
temperature=None,
|
||||
repetition_penalty=None,
|
||||
eos_token_id=model.generation_config.eos_token_id,
|
||||
pad_token_id=text_tokenizer.pad_token_id,
|
||||
use_cache=True
|
||||
)
|
||||
output_ids = model.generate(batch_input_ids, pixel_values=batch_pixel_values, attention_mask=batch_attention_mask,
|
||||
**gen_kwargs)
|
||||
|
||||
for i in range(len(batch_inputs)):
|
||||
output = text_tokenizer.decode(output_ids[i], skip_special_tokens=True)
|
||||
print(f'Output {i + 1}:\n{output}\n')
|
||||
```
|
||||
</details>
|
||||
|
||||
## Citation
|
||||
If you find Ovis useful, please consider citing the paper
|
||||
```
|
||||
@article{lu2024ovis,
|
||||
title={Ovis: Structural Embedding Alignment for Multimodal Large Language Model},
|
||||
author={Shiyin Lu and Yang Li and Qing-Guo Chen and Zhao Xu and Weihua Luo and Kaifu Zhang and Han-Jia Ye},
|
||||
year={2024},
|
||||
journal={arXiv:2405.20797}
|
||||
}
|
||||
```
|
||||
|
||||
## License
|
||||
This project is licensed under the [Apache License, Version 2.0](https://www.apache.org/licenses/LICENSE-2.0.txt) (SPDX-License-Identifier: Apache-2.0).
|
||||
|
||||
## Disclaimer
|
||||
We used compliance-checking algorithms during the training process, to ensure the compliance of the trained model to the best of our ability. Due to the complexity of the data and the diversity of language model usage scenarios, we cannot guarantee that the model is completely free of copyright issues or improper content. If you believe anything infringes on your rights or generates improper content, please contact us, and we will promptly address the matter.
|
|
@ -0,0 +1,24 @@
|
|||
{
|
||||
"</tool_call>": 151658,
|
||||
"<tool_call>": 151657,
|
||||
"<|box_end|>": 151649,
|
||||
"<|box_start|>": 151648,
|
||||
"<|endoftext|>": 151643,
|
||||
"<|file_sep|>": 151664,
|
||||
"<|fim_middle|>": 151660,
|
||||
"<|fim_pad|>": 151662,
|
||||
"<|fim_prefix|>": 151659,
|
||||
"<|fim_suffix|>": 151661,
|
||||
"<|im_end|>": 151645,
|
||||
"<|im_start|>": 151644,
|
||||
"<|image_pad|>": 151655,
|
||||
"<|object_ref_end|>": 151647,
|
||||
"<|object_ref_start|>": 151646,
|
||||
"<|quad_end|>": 151651,
|
||||
"<|quad_start|>": 151650,
|
||||
"<|repo_name|>": 151663,
|
||||
"<|video_pad|>": 151656,
|
||||
"<|vision_end|>": 151653,
|
||||
"<|vision_pad|>": 151654,
|
||||
"<|vision_start|>": 151652
|
||||
}
|
|
@ -0,0 +1,256 @@
|
|||
{
|
||||
"architectures": [
|
||||
"Ovis"
|
||||
],
|
||||
"auto_map": {
|
||||
"AutoConfig": "configuration_ovis.OvisConfig",
|
||||
"AutoModelForCausalLM": "modeling_ovis.Ovis"
|
||||
},
|
||||
"conversation_formatter_class": "QwenConversationFormatter",
|
||||
"disable_tie_weight": false,
|
||||
"hidden_size": 2048,
|
||||
"llm_attn_implementation": "flash_attention_2",
|
||||
"llm_config": {
|
||||
"_attn_implementation_autoset": true,
|
||||
"_name_or_path": "Qwen/Qwen2.5-3B-Instruct",
|
||||
"add_cross_attention": false,
|
||||
"architectures": [
|
||||
"Qwen2ForCausalLM"
|
||||
],
|
||||
"attention_dropout": 0.0,
|
||||
"bad_words_ids": null,
|
||||
"begin_suppress_tokens": null,
|
||||
"bos_token_id": 151643,
|
||||
"chunk_size_feed_forward": 0,
|
||||
"cross_attention_hidden_size": null,
|
||||
"decoder_start_token_id": null,
|
||||
"diversity_penalty": 0.0,
|
||||
"do_sample": false,
|
||||
"early_stopping": false,
|
||||
"encoder_no_repeat_ngram_size": 0,
|
||||
"eos_token_id": 151645,
|
||||
"exponential_decay_length_penalty": null,
|
||||
"finetuning_task": null,
|
||||
"forced_bos_token_id": null,
|
||||
"forced_eos_token_id": null,
|
||||
"hidden_act": "silu",
|
||||
"hidden_size": 2048,
|
||||
"id2label": {
|
||||
"0": "LABEL_0",
|
||||
"1": "LABEL_1"
|
||||
},
|
||||
"initializer_range": 0.02,
|
||||
"intermediate_size": 11008,
|
||||
"is_decoder": false,
|
||||
"is_encoder_decoder": false,
|
||||
"label2id": {
|
||||
"LABEL_0": 0,
|
||||
"LABEL_1": 1
|
||||
},
|
||||
"length_penalty": 1.0,
|
||||
"max_length": 20,
|
||||
"max_position_embeddings": 32768,
|
||||
"max_window_layers": 70,
|
||||
"min_length": 0,
|
||||
"model_type": "qwen2",
|
||||
"no_repeat_ngram_size": 0,
|
||||
"num_attention_heads": 16,
|
||||
"num_beam_groups": 1,
|
||||
"num_beams": 1,
|
||||
"num_hidden_layers": 36,
|
||||
"num_key_value_heads": 2,
|
||||
"num_return_sequences": 1,
|
||||
"output_attentions": false,
|
||||
"output_hidden_states": false,
|
||||
"output_scores": false,
|
||||
"pad_token_id": null,
|
||||
"prefix": null,
|
||||
"problem_type": null,
|
||||
"pruned_heads": {},
|
||||
"remove_invalid_values": false,
|
||||
"repetition_penalty": 1.0,
|
||||
"return_dict": true,
|
||||
"return_dict_in_generate": false,
|
||||
"rms_norm_eps": 1e-06,
|
||||
"rope_scaling": null,
|
||||
"rope_theta": 1000000.0,
|
||||
"sep_token_id": null,
|
||||
"sliding_window": null,
|
||||
"suppress_tokens": null,
|
||||
"task_specific_params": null,
|
||||
"temperature": 1.0,
|
||||
"tf_legacy_loss": false,
|
||||
"tie_encoder_decoder": false,
|
||||
"tie_word_embeddings": true,
|
||||
"tokenizer_class": null,
|
||||
"top_k": 50,
|
||||
"top_p": 1.0,
|
||||
"torch_dtype": "bfloat16",
|
||||
"torchscript": false,
|
||||
"typical_p": 1.0,
|
||||
"use_bfloat16": false,
|
||||
"use_cache": true,
|
||||
"use_sliding_window": false,
|
||||
"vocab_size": 151936
|
||||
},
|
||||
"model_type": "ovis",
|
||||
"multimodal_max_length": 32768,
|
||||
"torch_dtype": "bfloat16",
|
||||
"transformers_version": "4.46.2",
|
||||
"use_cache": true,
|
||||
"visual_tokenizer_config": {
|
||||
"_attn_implementation_autoset": true,
|
||||
"_name_or_path": "",
|
||||
"add_cross_attention": false,
|
||||
"architectures": null,
|
||||
"backbone_config": {
|
||||
"_attn_implementation_autoset": true,
|
||||
"_name_or_path": "apple/aimv2-huge-patch14-448",
|
||||
"add_cross_attention": false,
|
||||
"architectures": [
|
||||
"AIMv2Model"
|
||||
],
|
||||
"attention_dropout": 0.0,
|
||||
"auto_map": {
|
||||
"AutoConfig": "configuration_aimv2.AIMv2Config",
|
||||
"AutoModel": "modeling_aimv2.AIMv2Model",
|
||||
"FlaxAutoModel": "modeling_flax_aimv2.FlaxAIMv2Model"
|
||||
},
|
||||
"bad_words_ids": null,
|
||||
"begin_suppress_tokens": null,
|
||||
"bos_token_id": null,
|
||||
"chunk_size_feed_forward": 0,
|
||||
"cross_attention_hidden_size": null,
|
||||
"decoder_start_token_id": null,
|
||||
"diversity_penalty": 0.0,
|
||||
"do_sample": false,
|
||||
"early_stopping": false,
|
||||
"encoder_no_repeat_ngram_size": 0,
|
||||
"eos_token_id": null,
|
||||
"exponential_decay_length_penalty": null,
|
||||
"finetuning_task": null,
|
||||
"forced_bos_token_id": null,
|
||||
"forced_eos_token_id": null,
|
||||
"hidden_size": 1536,
|
||||
"id2label": {
|
||||
"0": "LABEL_0",
|
||||
"1": "LABEL_1"
|
||||
},
|
||||
"image_size": 448,
|
||||
"intermediate_size": 4096,
|
||||
"is_decoder": false,
|
||||
"is_encoder_decoder": false,
|
||||
"label2id": {
|
||||
"LABEL_0": 0,
|
||||
"LABEL_1": 1
|
||||
},
|
||||
"length_penalty": 1.0,
|
||||
"max_length": 20,
|
||||
"min_length": 0,
|
||||
"model_type": "aimv2",
|
||||
"no_repeat_ngram_size": 0,
|
||||
"num_attention_heads": 12,
|
||||
"num_beam_groups": 1,
|
||||
"num_beams": 1,
|
||||
"num_channels": 3,
|
||||
"num_hidden_layers": 24,
|
||||
"num_return_sequences": 1,
|
||||
"output_attentions": false,
|
||||
"output_hidden_states": false,
|
||||
"output_scores": false,
|
||||
"pad_token_id": null,
|
||||
"patch_size": 14,
|
||||
"prefix": null,
|
||||
"problem_type": null,
|
||||
"projection_dropout": 0.0,
|
||||
"pruned_heads": {},
|
||||
"qkv_bias": false,
|
||||
"remove_invalid_values": false,
|
||||
"repetition_penalty": 1.0,
|
||||
"return_dict": true,
|
||||
"return_dict_in_generate": false,
|
||||
"rms_norm_eps": 1e-05,
|
||||
"sep_token_id": null,
|
||||
"suppress_tokens": null,
|
||||
"task_specific_params": null,
|
||||
"temperature": 1.0,
|
||||
"tf_legacy_loss": false,
|
||||
"tie_encoder_decoder": false,
|
||||
"tie_word_embeddings": true,
|
||||
"tokenizer_class": null,
|
||||
"top_k": 50,
|
||||
"top_p": 1.0,
|
||||
"torch_dtype": "float32",
|
||||
"torchscript": false,
|
||||
"typical_p": 1.0,
|
||||
"use_bfloat16": false,
|
||||
"use_bias": false
|
||||
},
|
||||
"backbone_kwargs": {},
|
||||
"bad_words_ids": null,
|
||||
"begin_suppress_tokens": null,
|
||||
"bos_token_id": null,
|
||||
"chunk_size_feed_forward": 0,
|
||||
"cross_attention_hidden_size": null,
|
||||
"decoder_start_token_id": null,
|
||||
"depths": null,
|
||||
"diversity_penalty": 0.0,
|
||||
"do_sample": false,
|
||||
"drop_cls_token": false,
|
||||
"early_stopping": false,
|
||||
"encoder_no_repeat_ngram_size": 0,
|
||||
"eos_token_id": null,
|
||||
"exponential_decay_length_penalty": null,
|
||||
"finetuning_task": null,
|
||||
"forced_bos_token_id": null,
|
||||
"forced_eos_token_id": null,
|
||||
"hidden_stride": 2,
|
||||
"id2label": {
|
||||
"0": "LABEL_0",
|
||||
"1": "LABEL_1"
|
||||
},
|
||||
"is_decoder": false,
|
||||
"is_encoder_decoder": false,
|
||||
"label2id": {
|
||||
"LABEL_0": 0,
|
||||
"LABEL_1": 1
|
||||
},
|
||||
"length_penalty": 1.0,
|
||||
"max_length": 20,
|
||||
"min_length": 0,
|
||||
"model_type": "aimv2_visual_tokenizer",
|
||||
"no_repeat_ngram_size": 0,
|
||||
"num_beam_groups": 1,
|
||||
"num_beams": 1,
|
||||
"num_return_sequences": 1,
|
||||
"output_attentions": false,
|
||||
"output_hidden_states": false,
|
||||
"output_scores": false,
|
||||
"pad_token_id": null,
|
||||
"prefix": null,
|
||||
"problem_type": null,
|
||||
"pruned_heads": {},
|
||||
"remove_invalid_values": false,
|
||||
"repetition_penalty": 1.0,
|
||||
"return_dict": true,
|
||||
"return_dict_in_generate": false,
|
||||
"sep_token_id": null,
|
||||
"suppress_tokens": null,
|
||||
"task_specific_params": null,
|
||||
"tau": 1.0,
|
||||
"temperature": 1.0,
|
||||
"tf_legacy_loss": false,
|
||||
"tie_encoder_decoder": false,
|
||||
"tie_word_embeddings": true,
|
||||
"tokenize_function": "softmax",
|
||||
"tokenizer_class": null,
|
||||
"top_k": 50,
|
||||
"top_p": 1.0,
|
||||
"torch_dtype": null,
|
||||
"torchscript": false,
|
||||
"typical_p": 1.0,
|
||||
"use_bfloat16": false,
|
||||
"use_indicators": false,
|
||||
"vocab_size": 65536
|
||||
}
|
||||
}
|
|
@ -0,0 +1,63 @@
|
|||
# copied from https://huggingface.co/apple/aimv2-huge-patch14-448
|
||||
from typing import Any
|
||||
|
||||
from transformers.configuration_utils import PretrainedConfig
|
||||
|
||||
__all__ = ["AIMv2Config"]
|
||||
|
||||
|
||||
class AIMv2Config(PretrainedConfig):
|
||||
"""This is the configuration class to store the configuration of an [`AIMv2Model`].
|
||||
|
||||
Instantiating a configuration with the defaults will yield a similar configuration
|
||||
to that of the [apple/aimv2-large-patch14-224](https://huggingface.co/apple/aimv2-large-patch14-224).
|
||||
|
||||
Args:
|
||||
hidden_size: Dimension of the hidden representations.
|
||||
intermediate_size: Dimension of the SwiGLU representations.
|
||||
num_hidden_layers: Number of hidden layers in the Transformer.
|
||||
num_attention_heads: Number of attention heads for each attention layer
|
||||
in the Transformer.
|
||||
num_channels: Number of input channels.
|
||||
image_size: Image size.
|
||||
patch_size: Patch size.
|
||||
rms_norm_eps: Epsilon value used for the RMS normalization layer.
|
||||
attention_dropout: Dropout ratio for attention probabilities.
|
||||
projection_dropout: Dropout ratio for the projection layer after the attention.
|
||||
qkv_bias: Whether to add a bias to the queries, keys and values.
|
||||
use_bias: Whether to add a bias in the feed-forward and projection layers.
|
||||
kwargs: Keyword arguments for the [`PretrainedConfig`].
|
||||
"""
|
||||
|
||||
model_type: str = "aimv2"
|
||||
|
||||
def __init__(
|
||||
self,
|
||||
hidden_size: int = 1024,
|
||||
intermediate_size: int = 2816,
|
||||
num_hidden_layers: int = 24,
|
||||
num_attention_heads: int = 8,
|
||||
num_channels: int = 3,
|
||||
image_size: int = 224,
|
||||
patch_size: int = 14,
|
||||
rms_norm_eps: float = 1e-5,
|
||||
attention_dropout: float = 0.0,
|
||||
projection_dropout: float = 0.0,
|
||||
qkv_bias: bool = False,
|
||||
use_bias: bool = False,
|
||||
**kwargs: Any,
|
||||
):
|
||||
super().__init__(**kwargs)
|
||||
self.hidden_size = hidden_size
|
||||
self.intermediate_size = intermediate_size
|
||||
self.num_hidden_layers = num_hidden_layers
|
||||
self.num_attention_heads = num_attention_heads
|
||||
self.num_channels = num_channels
|
||||
self.patch_size = patch_size
|
||||
self.image_size = image_size
|
||||
self.attention_dropout = attention_dropout
|
||||
self.rms_norm_eps = rms_norm_eps
|
||||
|
||||
self.projection_dropout = projection_dropout
|
||||
self.qkv_bias = qkv_bias
|
||||
self.use_bias = use_bias
|
|
@ -0,0 +1,204 @@
|
|||
from abc import ABC, abstractmethod
|
||||
from typing import List, Dict, Union, Optional
|
||||
|
||||
from transformers import PretrainedConfig, AutoConfig, AutoModel
|
||||
from .configuration_aimv2 import AIMv2Config
|
||||
from .modeling_aimv2 import AIMv2Model
|
||||
|
||||
IGNORE_ID = -100
|
||||
IMAGE_TOKEN_ID = -200
|
||||
IMAGE_TOKEN = "<image>"
|
||||
IMAGE_ATOM_ID = -300
|
||||
IMAGE_INDICATOR_IDS = [-301, -302, -303, -304, -305]
|
||||
|
||||
AutoConfig.register("aimv2", AIMv2Config)
|
||||
AutoModel.register(AIMv2Config, AIMv2Model)
|
||||
|
||||
# ----------------------------------------------------------------------
|
||||
# Visual Tokenizer Configuration
|
||||
# ----------------------------------------------------------------------
|
||||
class BaseVisualTokenizerConfig(PretrainedConfig):
|
||||
def __init__(
|
||||
self,
|
||||
vocab_size=16384,
|
||||
tokenize_function="softmax",
|
||||
tau=1.0,
|
||||
depths=None,
|
||||
drop_cls_token=False,
|
||||
backbone_config: Optional[Union[PretrainedConfig, dict]] = None,
|
||||
hidden_stride: int = 1,
|
||||
**kwargs
|
||||
):
|
||||
super().__init__(**kwargs)
|
||||
self.vocab_size = vocab_size
|
||||
self.tokenize_function = tokenize_function
|
||||
self.tau = tau
|
||||
if isinstance(depths, str):
|
||||
depths = [int(x) for x in depths.split('|')]
|
||||
self.depths = depths
|
||||
self.backbone_kwargs = {}
|
||||
self.drop_cls_token = drop_cls_token
|
||||
if backbone_config is not None:
|
||||
assert isinstance(backbone_config, (PretrainedConfig, dict)), \
|
||||
f"expect `backbone_config` to be instance of PretrainedConfig or dict, but got {type(backbone_config)} type"
|
||||
if not isinstance(backbone_config, PretrainedConfig):
|
||||
model_type = backbone_config['model_type']
|
||||
backbone_config.pop('model_type')
|
||||
backbone_config = AutoConfig.for_model(model_type, **backbone_config)
|
||||
self.backbone_config = backbone_config
|
||||
self.hidden_stride = hidden_stride
|
||||
|
||||
|
||||
class Aimv2VisualTokenizerConfig(BaseVisualTokenizerConfig):
|
||||
model_type = "aimv2_visual_tokenizer"
|
||||
|
||||
def __init__(self, **kwargs):
|
||||
super().__init__(**kwargs)
|
||||
if self.drop_cls_token:
|
||||
self.drop_cls_token = False
|
||||
if self.depths:
|
||||
assert len(self.depths) == 1
|
||||
self.backbone_kwargs['num_hidden_layers'] = self.depths[0]
|
||||
|
||||
|
||||
AutoConfig.register("aimv2_visual_tokenizer", Aimv2VisualTokenizerConfig)
|
||||
|
||||
|
||||
# ----------------------------------------------------------------------
|
||||
# Ovis Configuration
|
||||
# ----------------------------------------------------------------------
|
||||
class OvisConfig(PretrainedConfig):
|
||||
model_type = "ovis"
|
||||
|
||||
def __init__(
|
||||
self,
|
||||
llm_config: Optional[Union[PretrainedConfig, dict]] = None,
|
||||
visual_tokenizer_config: Optional[Union[PretrainedConfig, dict]] = None,
|
||||
multimodal_max_length=8192,
|
||||
hidden_size=None,
|
||||
conversation_formatter_class=None,
|
||||
llm_attn_implementation=None,
|
||||
disable_tie_weight=False,
|
||||
**kwargs
|
||||
):
|
||||
super().__init__(**kwargs)
|
||||
if llm_config is not None:
|
||||
assert isinstance(llm_config, (PretrainedConfig, dict)), \
|
||||
f"expect `llm_config` to be instance of PretrainedConfig or dict, but got {type(llm_config)} type"
|
||||
if not isinstance(llm_config, PretrainedConfig):
|
||||
model_type = llm_config['model_type']
|
||||
llm_config.pop('model_type')
|
||||
llm_config = AutoConfig.for_model(model_type, **llm_config)
|
||||
self.llm_config = llm_config
|
||||
if visual_tokenizer_config is not None:
|
||||
assert isinstance(visual_tokenizer_config, (PretrainedConfig, dict)), \
|
||||
f"expect `visual_tokenizer_config` to be instance of PretrainedConfig or dict, but got {type(visual_tokenizer_config)} type"
|
||||
if not isinstance(visual_tokenizer_config, PretrainedConfig):
|
||||
model_type = visual_tokenizer_config['model_type']
|
||||
visual_tokenizer_config.pop('model_type')
|
||||
visual_tokenizer_config = AutoConfig.for_model(model_type, **visual_tokenizer_config)
|
||||
self.visual_tokenizer_config = visual_tokenizer_config
|
||||
self.multimodal_max_length = multimodal_max_length
|
||||
self.hidden_size = hidden_size
|
||||
self.conversation_formatter_class = conversation_formatter_class
|
||||
self.llm_attn_implementation = llm_attn_implementation
|
||||
self.disable_tie_weight = disable_tie_weight
|
||||
|
||||
|
||||
# ----------------------------------------------------------------------
|
||||
# Conversation Formatter
|
||||
# ----------------------------------------------------------------------
|
||||
class ConversationFormatter(ABC):
|
||||
support_tokenizer_types = None
|
||||
|
||||
def __init__(self, tokenizer):
|
||||
tokenizer_type = type(tokenizer).__name__
|
||||
assert tokenizer_type in self.support_tokenizer_types, \
|
||||
f'Invalid tokenizer type, expected one from `{self.support_tokenizer_types}`, but got `{tokenizer_type}`'
|
||||
self.tokenizer = tokenizer
|
||||
self.image_token = IMAGE_TOKEN
|
||||
self.image_token_id = IMAGE_TOKEN_ID
|
||||
self.ignore_id = IGNORE_ID
|
||||
|
||||
def _tokenize_with_image_symbol(self, text):
|
||||
text_chunks = [self.tokenizer(chunk, add_special_tokens=False).input_ids for chunk in
|
||||
text.split(self.image_token)]
|
||||
token_ids = []
|
||||
num_chuck = len(text_chunks)
|
||||
for i, chunk in enumerate(text_chunks):
|
||||
token_ids.extend(chunk)
|
||||
if i < num_chuck - 1:
|
||||
token_ids.append(self.image_token_id)
|
||||
return token_ids
|
||||
|
||||
@abstractmethod
|
||||
def format(self, conversations: List[Dict], generation_preface=None):
|
||||
pass
|
||||
|
||||
@abstractmethod
|
||||
def format_query(self, query, generation_preface=""):
|
||||
pass
|
||||
|
||||
|
||||
class QwenConversationFormatter(ConversationFormatter):
|
||||
support_tokenizer_types = ['QWenTokenizer', 'Qwen2TokenizerFast']
|
||||
|
||||
def __init__(self, tokenizer):
|
||||
super().__init__(tokenizer)
|
||||
self.from2role = {
|
||||
"system": "<|im_start|>system\n",
|
||||
"human": "<|im_start|>user\n",
|
||||
"gpt": "<|im_start|>assistant\n",
|
||||
}
|
||||
self.gpt_token_num = None
|
||||
self.im_end = "<|im_end|>\n"
|
||||
self.default_system_prompt = "You are a helpful assistant."
|
||||
|
||||
def format(self, conversations: List[Dict], generation_preface=None):
|
||||
if self.gpt_token_num is None:
|
||||
self.gpt_token_num = len(self.tokenizer(self.from2role["gpt"], add_special_tokens=False).input_ids)
|
||||
|
||||
if conversations[0]["from"] != "system":
|
||||
conversations.insert(0, {
|
||||
"from": "system",
|
||||
"value": self.default_system_prompt
|
||||
})
|
||||
|
||||
if generation_preface is not None:
|
||||
conversations.append({
|
||||
"from": "gpt",
|
||||
"value": generation_preface
|
||||
})
|
||||
|
||||
prompt = ""
|
||||
input_ids = []
|
||||
labels = []
|
||||
num_conversation = len(conversations)
|
||||
for i, conversation in enumerate(conversations):
|
||||
frm = conversation["from"]
|
||||
role = self.from2role[frm]
|
||||
message = conversation["value"]
|
||||
text = role + message
|
||||
if i < num_conversation - 1 or generation_preface is None:
|
||||
text += self.im_end
|
||||
prompt += text
|
||||
token_ids = self._tokenize_with_image_symbol(text)
|
||||
input_ids.extend(token_ids)
|
||||
label_ids = [self.ignore_id] * len(token_ids)
|
||||
if frm == "gpt" and generation_preface is None:
|
||||
# learning `\n` following `im_end` is meaningless, so the last `\n` token is ignored in label
|
||||
label_ids[self.gpt_token_num:-1] = token_ids[self.gpt_token_num:-1]
|
||||
labels.extend(label_ids)
|
||||
|
||||
assert self._tokenize_with_image_symbol(prompt) == input_ids
|
||||
assert len(input_ids) == len(labels)
|
||||
|
||||
return prompt, input_ids, labels
|
||||
|
||||
def format_query(self, query, generation_preface=""):
|
||||
prompt, input_ids, _ = self.format([{
|
||||
"from": "human",
|
||||
"value": query
|
||||
}], generation_preface=generation_preface)
|
||||
|
||||
return prompt, input_ids
|
|
@ -0,0 +1,15 @@
|
|||
{
|
||||
"bos_token_id": 151643,
|
||||
"do_sample": true,
|
||||
"eos_token_id": [
|
||||
151645,
|
||||
151643
|
||||
],
|
||||
"multimodal_max_length": 32768,
|
||||
"pad_token_id": 151643,
|
||||
"repetition_penalty": 1.05,
|
||||
"temperature": 0.7,
|
||||
"top_k": 20,
|
||||
"top_p": 0.8,
|
||||
"transformers_version": "4.46.2"
|
||||
}
|
File diff suppressed because it is too large
Load Diff
|
@ -0,0 +1,619 @@
|
|||
{
|
||||
"metadata": {
|
||||
"total_size": 9232212972
|
||||
},
|
||||
"weight_map": {
|
||||
"llm.lm_head.weight": "model-00002-of-00002.safetensors",
|
||||
"llm.model.embed_tokens.weight": "model-00001-of-00002.safetensors",
|
||||
"llm.model.layers.0.input_layernorm.weight": "model-00001-of-00002.safetensors",
|
||||
"llm.model.layers.0.mlp.down_proj.weight": "model-00001-of-00002.safetensors",
|
||||
"llm.model.layers.0.mlp.gate_proj.weight": "model-00001-of-00002.safetensors",
|
||||
"llm.model.layers.0.mlp.up_proj.weight": "model-00001-of-00002.safetensors",
|
||||
"llm.model.layers.0.post_attention_layernorm.weight": "model-00001-of-00002.safetensors",
|
||||
"llm.model.layers.0.self_attn.k_proj.bias": "model-00001-of-00002.safetensors",
|
||||
"llm.model.layers.0.self_attn.k_proj.weight": "model-00001-of-00002.safetensors",
|
||||
"llm.model.layers.0.self_attn.o_proj.weight": "model-00001-of-00002.safetensors",
|
||||
"llm.model.layers.0.self_attn.q_proj.bias": "model-00001-of-00002.safetensors",
|
||||
"llm.model.layers.0.self_attn.q_proj.weight": "model-00001-of-00002.safetensors",
|
||||
"llm.model.layers.0.self_attn.v_proj.bias": "model-00001-of-00002.safetensors",
|
||||
"llm.model.layers.0.self_attn.v_proj.weight": "model-00001-of-00002.safetensors",
|
||||
"llm.model.layers.1.input_layernorm.weight": "model-00001-of-00002.safetensors",
|
||||
"llm.model.layers.1.mlp.down_proj.weight": "model-00001-of-00002.safetensors",
|
||||
"llm.model.layers.1.mlp.gate_proj.weight": "model-00001-of-00002.safetensors",
|
||||
"llm.model.layers.1.mlp.up_proj.weight": "model-00001-of-00002.safetensors",
|
||||
"llm.model.layers.1.post_attention_layernorm.weight": "model-00001-of-00002.safetensors",
|
||||
"llm.model.layers.1.self_attn.k_proj.bias": "model-00001-of-00002.safetensors",
|
||||
"llm.model.layers.1.self_attn.k_proj.weight": "model-00001-of-00002.safetensors",
|
||||
"llm.model.layers.1.self_attn.o_proj.weight": "model-00001-of-00002.safetensors",
|
||||
"llm.model.layers.1.self_attn.q_proj.bias": "model-00001-of-00002.safetensors",
|
||||
"llm.model.layers.1.self_attn.q_proj.weight": "model-00001-of-00002.safetensors",
|
||||
"llm.model.layers.1.self_attn.v_proj.bias": "model-00001-of-00002.safetensors",
|
||||
"llm.model.layers.1.self_attn.v_proj.weight": "model-00001-of-00002.safetensors",
|
||||
"llm.model.layers.10.input_layernorm.weight": "model-00001-of-00002.safetensors",
|
||||
"llm.model.layers.10.mlp.down_proj.weight": "model-00001-of-00002.safetensors",
|
||||
"llm.model.layers.10.mlp.gate_proj.weight": "model-00001-of-00002.safetensors",
|
||||
"llm.model.layers.10.mlp.up_proj.weight": "model-00001-of-00002.safetensors",
|
||||
"llm.model.layers.10.post_attention_layernorm.weight": "model-00001-of-00002.safetensors",
|
||||
"llm.model.layers.10.self_attn.k_proj.bias": "model-00001-of-00002.safetensors",
|
||||
"llm.model.layers.10.self_attn.k_proj.weight": "model-00001-of-00002.safetensors",
|
||||
"llm.model.layers.10.self_attn.o_proj.weight": "model-00001-of-00002.safetensors",
|
||||
"llm.model.layers.10.self_attn.q_proj.bias": "model-00001-of-00002.safetensors",
|
||||
"llm.model.layers.10.self_attn.q_proj.weight": "model-00001-of-00002.safetensors",
|
||||
"llm.model.layers.10.self_attn.v_proj.bias": "model-00001-of-00002.safetensors",
|
||||
"llm.model.layers.10.self_attn.v_proj.weight": "model-00001-of-00002.safetensors",
|
||||
"llm.model.layers.11.input_layernorm.weight": "model-00001-of-00002.safetensors",
|
||||
"llm.model.layers.11.mlp.down_proj.weight": "model-00001-of-00002.safetensors",
|
||||
"llm.model.layers.11.mlp.gate_proj.weight": "model-00001-of-00002.safetensors",
|
||||
"llm.model.layers.11.mlp.up_proj.weight": "model-00001-of-00002.safetensors",
|
||||
"llm.model.layers.11.post_attention_layernorm.weight": "model-00001-of-00002.safetensors",
|
||||
"llm.model.layers.11.self_attn.k_proj.bias": "model-00001-of-00002.safetensors",
|
||||
"llm.model.layers.11.self_attn.k_proj.weight": "model-00001-of-00002.safetensors",
|
||||
"llm.model.layers.11.self_attn.o_proj.weight": "model-00001-of-00002.safetensors",
|
||||
"llm.model.layers.11.self_attn.q_proj.bias": "model-00001-of-00002.safetensors",
|
||||
"llm.model.layers.11.self_attn.q_proj.weight": "model-00001-of-00002.safetensors",
|
||||
"llm.model.layers.11.self_attn.v_proj.bias": "model-00001-of-00002.safetensors",
|
||||
"llm.model.layers.11.self_attn.v_proj.weight": "model-00001-of-00002.safetensors",
|
||||
"llm.model.layers.12.input_layernorm.weight": "model-00001-of-00002.safetensors",
|
||||
"llm.model.layers.12.mlp.down_proj.weight": "model-00001-of-00002.safetensors",
|
||||
"llm.model.layers.12.mlp.gate_proj.weight": "model-00001-of-00002.safetensors",
|
||||
"llm.model.layers.12.mlp.up_proj.weight": "model-00001-of-00002.safetensors",
|
||||
"llm.model.layers.12.post_attention_layernorm.weight": "model-00001-of-00002.safetensors",
|
||||
"llm.model.layers.12.self_attn.k_proj.bias": "model-00001-of-00002.safetensors",
|
||||
"llm.model.layers.12.self_attn.k_proj.weight": "model-00001-of-00002.safetensors",
|
||||
"llm.model.layers.12.self_attn.o_proj.weight": "model-00001-of-00002.safetensors",
|
||||
"llm.model.layers.12.self_attn.q_proj.bias": "model-00001-of-00002.safetensors",
|
||||
"llm.model.layers.12.self_attn.q_proj.weight": "model-00001-of-00002.safetensors",
|
||||
"llm.model.layers.12.self_attn.v_proj.bias": "model-00001-of-00002.safetensors",
|
||||
"llm.model.layers.12.self_attn.v_proj.weight": "model-00001-of-00002.safetensors",
|
||||
"llm.model.layers.13.input_layernorm.weight": "model-00001-of-00002.safetensors",
|
||||
"llm.model.layers.13.mlp.down_proj.weight": "model-00001-of-00002.safetensors",
|
||||
"llm.model.layers.13.mlp.gate_proj.weight": "model-00001-of-00002.safetensors",
|
||||
"llm.model.layers.13.mlp.up_proj.weight": "model-00001-of-00002.safetensors",
|
||||
"llm.model.layers.13.post_attention_layernorm.weight": "model-00001-of-00002.safetensors",
|
||||
"llm.model.layers.13.self_attn.k_proj.bias": "model-00001-of-00002.safetensors",
|
||||
"llm.model.layers.13.self_attn.k_proj.weight": "model-00001-of-00002.safetensors",
|
||||
"llm.model.layers.13.self_attn.o_proj.weight": "model-00001-of-00002.safetensors",
|
||||
"llm.model.layers.13.self_attn.q_proj.bias": "model-00001-of-00002.safetensors",
|
||||
"llm.model.layers.13.self_attn.q_proj.weight": "model-00001-of-00002.safetensors",
|
||||
"llm.model.layers.13.self_attn.v_proj.bias": "model-00001-of-00002.safetensors",
|
||||
"llm.model.layers.13.self_attn.v_proj.weight": "model-00001-of-00002.safetensors",
|
||||
"llm.model.layers.14.input_layernorm.weight": "model-00001-of-00002.safetensors",
|
||||
"llm.model.layers.14.mlp.down_proj.weight": "model-00001-of-00002.safetensors",
|
||||
"llm.model.layers.14.mlp.gate_proj.weight": "model-00001-of-00002.safetensors",
|
||||
"llm.model.layers.14.mlp.up_proj.weight": "model-00001-of-00002.safetensors",
|
||||
"llm.model.layers.14.post_attention_layernorm.weight": "model-00001-of-00002.safetensors",
|
||||
"llm.model.layers.14.self_attn.k_proj.bias": "model-00001-of-00002.safetensors",
|
||||
"llm.model.layers.14.self_attn.k_proj.weight": "model-00001-of-00002.safetensors",
|
||||
"llm.model.layers.14.self_attn.o_proj.weight": "model-00001-of-00002.safetensors",
|
||||
"llm.model.layers.14.self_attn.q_proj.bias": "model-00001-of-00002.safetensors",
|
||||
"llm.model.layers.14.self_attn.q_proj.weight": "model-00001-of-00002.safetensors",
|
||||
"llm.model.layers.14.self_attn.v_proj.bias": "model-00001-of-00002.safetensors",
|
||||
"llm.model.layers.14.self_attn.v_proj.weight": "model-00001-of-00002.safetensors",
|
||||
"llm.model.layers.15.input_layernorm.weight": "model-00001-of-00002.safetensors",
|
||||
"llm.model.layers.15.mlp.down_proj.weight": "model-00001-of-00002.safetensors",
|
||||
"llm.model.layers.15.mlp.gate_proj.weight": "model-00001-of-00002.safetensors",
|
||||
"llm.model.layers.15.mlp.up_proj.weight": "model-00001-of-00002.safetensors",
|
||||
"llm.model.layers.15.post_attention_layernorm.weight": "model-00001-of-00002.safetensors",
|
||||
"llm.model.layers.15.self_attn.k_proj.bias": "model-00001-of-00002.safetensors",
|
||||
"llm.model.layers.15.self_attn.k_proj.weight": "model-00001-of-00002.safetensors",
|
||||
"llm.model.layers.15.self_attn.o_proj.weight": "model-00001-of-00002.safetensors",
|
||||
"llm.model.layers.15.self_attn.q_proj.bias": "model-00001-of-00002.safetensors",
|
||||
"llm.model.layers.15.self_attn.q_proj.weight": "model-00001-of-00002.safetensors",
|
||||
"llm.model.layers.15.self_attn.v_proj.bias": "model-00001-of-00002.safetensors",
|
||||
"llm.model.layers.15.self_attn.v_proj.weight": "model-00001-of-00002.safetensors",
|
||||
"llm.model.layers.16.input_layernorm.weight": "model-00001-of-00002.safetensors",
|
||||
"llm.model.layers.16.mlp.down_proj.weight": "model-00001-of-00002.safetensors",
|
||||
"llm.model.layers.16.mlp.gate_proj.weight": "model-00001-of-00002.safetensors",
|
||||
"llm.model.layers.16.mlp.up_proj.weight": "model-00001-of-00002.safetensors",
|
||||
"llm.model.layers.16.post_attention_layernorm.weight": "model-00001-of-00002.safetensors",
|
||||
"llm.model.layers.16.self_attn.k_proj.bias": "model-00001-of-00002.safetensors",
|
||||
"llm.model.layers.16.self_attn.k_proj.weight": "model-00001-of-00002.safetensors",
|
||||
"llm.model.layers.16.self_attn.o_proj.weight": "model-00001-of-00002.safetensors",
|
||||
"llm.model.layers.16.self_attn.q_proj.bias": "model-00001-of-00002.safetensors",
|
||||
"llm.model.layers.16.self_attn.q_proj.weight": "model-00001-of-00002.safetensors",
|
||||
"llm.model.layers.16.self_attn.v_proj.bias": "model-00001-of-00002.safetensors",
|
||||
"llm.model.layers.16.self_attn.v_proj.weight": "model-00001-of-00002.safetensors",
|
||||
"llm.model.layers.17.input_layernorm.weight": "model-00001-of-00002.safetensors",
|
||||
"llm.model.layers.17.mlp.down_proj.weight": "model-00001-of-00002.safetensors",
|
||||
"llm.model.layers.17.mlp.gate_proj.weight": "model-00001-of-00002.safetensors",
|
||||
"llm.model.layers.17.mlp.up_proj.weight": "model-00001-of-00002.safetensors",
|
||||
"llm.model.layers.17.post_attention_layernorm.weight": "model-00001-of-00002.safetensors",
|
||||
"llm.model.layers.17.self_attn.k_proj.bias": "model-00001-of-00002.safetensors",
|
||||
"llm.model.layers.17.self_attn.k_proj.weight": "model-00001-of-00002.safetensors",
|
||||
"llm.model.layers.17.self_attn.o_proj.weight": "model-00001-of-00002.safetensors",
|
||||
"llm.model.layers.17.self_attn.q_proj.bias": "model-00001-of-00002.safetensors",
|
||||
"llm.model.layers.17.self_attn.q_proj.weight": "model-00001-of-00002.safetensors",
|
||||
"llm.model.layers.17.self_attn.v_proj.bias": "model-00001-of-00002.safetensors",
|
||||
"llm.model.layers.17.self_attn.v_proj.weight": "model-00001-of-00002.safetensors",
|
||||
"llm.model.layers.18.input_layernorm.weight": "model-00001-of-00002.safetensors",
|
||||
"llm.model.layers.18.mlp.down_proj.weight": "model-00001-of-00002.safetensors",
|
||||
"llm.model.layers.18.mlp.gate_proj.weight": "model-00001-of-00002.safetensors",
|
||||
"llm.model.layers.18.mlp.up_proj.weight": "model-00001-of-00002.safetensors",
|
||||
"llm.model.layers.18.post_attention_layernorm.weight": "model-00001-of-00002.safetensors",
|
||||
"llm.model.layers.18.self_attn.k_proj.bias": "model-00001-of-00002.safetensors",
|
||||
"llm.model.layers.18.self_attn.k_proj.weight": "model-00001-of-00002.safetensors",
|
||||
"llm.model.layers.18.self_attn.o_proj.weight": "model-00001-of-00002.safetensors",
|
||||
"llm.model.layers.18.self_attn.q_proj.bias": "model-00001-of-00002.safetensors",
|
||||
"llm.model.layers.18.self_attn.q_proj.weight": "model-00001-of-00002.safetensors",
|
||||
"llm.model.layers.18.self_attn.v_proj.bias": "model-00001-of-00002.safetensors",
|
||||
"llm.model.layers.18.self_attn.v_proj.weight": "model-00001-of-00002.safetensors",
|
||||
"llm.model.layers.19.input_layernorm.weight": "model-00001-of-00002.safetensors",
|
||||
"llm.model.layers.19.mlp.down_proj.weight": "model-00001-of-00002.safetensors",
|
||||
"llm.model.layers.19.mlp.gate_proj.weight": "model-00001-of-00002.safetensors",
|
||||
"llm.model.layers.19.mlp.up_proj.weight": "model-00001-of-00002.safetensors",
|
||||
"llm.model.layers.19.post_attention_layernorm.weight": "model-00001-of-00002.safetensors",
|
||||
"llm.model.layers.19.self_attn.k_proj.bias": "model-00001-of-00002.safetensors",
|
||||
"llm.model.layers.19.self_attn.k_proj.weight": "model-00001-of-00002.safetensors",
|
||||
"llm.model.layers.19.self_attn.o_proj.weight": "model-00001-of-00002.safetensors",
|
||||
"llm.model.layers.19.self_attn.q_proj.bias": "model-00001-of-00002.safetensors",
|
||||
"llm.model.layers.19.self_attn.q_proj.weight": "model-00001-of-00002.safetensors",
|
||||
"llm.model.layers.19.self_attn.v_proj.bias": "model-00001-of-00002.safetensors",
|
||||
"llm.model.layers.19.self_attn.v_proj.weight": "model-00001-of-00002.safetensors",
|
||||
"llm.model.layers.2.input_layernorm.weight": "model-00001-of-00002.safetensors",
|
||||
"llm.model.layers.2.mlp.down_proj.weight": "model-00001-of-00002.safetensors",
|
||||
"llm.model.layers.2.mlp.gate_proj.weight": "model-00001-of-00002.safetensors",
|
||||
"llm.model.layers.2.mlp.up_proj.weight": "model-00001-of-00002.safetensors",
|
||||
"llm.model.layers.2.post_attention_layernorm.weight": "model-00001-of-00002.safetensors",
|
||||
"llm.model.layers.2.self_attn.k_proj.bias": "model-00001-of-00002.safetensors",
|
||||
"llm.model.layers.2.self_attn.k_proj.weight": "model-00001-of-00002.safetensors",
|
||||
"llm.model.layers.2.self_attn.o_proj.weight": "model-00001-of-00002.safetensors",
|
||||
"llm.model.layers.2.self_attn.q_proj.bias": "model-00001-of-00002.safetensors",
|
||||
"llm.model.layers.2.self_attn.q_proj.weight": "model-00001-of-00002.safetensors",
|
||||
"llm.model.layers.2.self_attn.v_proj.bias": "model-00001-of-00002.safetensors",
|
||||
"llm.model.layers.2.self_attn.v_proj.weight": "model-00001-of-00002.safetensors",
|
||||
"llm.model.layers.20.input_layernorm.weight": "model-00001-of-00002.safetensors",
|
||||
"llm.model.layers.20.mlp.down_proj.weight": "model-00001-of-00002.safetensors",
|
||||
"llm.model.layers.20.mlp.gate_proj.weight": "model-00001-of-00002.safetensors",
|
||||
"llm.model.layers.20.mlp.up_proj.weight": "model-00001-of-00002.safetensors",
|
||||
"llm.model.layers.20.post_attention_layernorm.weight": "model-00001-of-00002.safetensors",
|
||||
"llm.model.layers.20.self_attn.k_proj.bias": "model-00001-of-00002.safetensors",
|
||||
"llm.model.layers.20.self_attn.k_proj.weight": "model-00001-of-00002.safetensors",
|
||||
"llm.model.layers.20.self_attn.o_proj.weight": "model-00001-of-00002.safetensors",
|
||||
"llm.model.layers.20.self_attn.q_proj.bias": "model-00001-of-00002.safetensors",
|
||||
"llm.model.layers.20.self_attn.q_proj.weight": "model-00001-of-00002.safetensors",
|
||||
"llm.model.layers.20.self_attn.v_proj.bias": "model-00001-of-00002.safetensors",
|
||||
"llm.model.layers.20.self_attn.v_proj.weight": "model-00001-of-00002.safetensors",
|
||||
"llm.model.layers.21.input_layernorm.weight": "model-00001-of-00002.safetensors",
|
||||
"llm.model.layers.21.mlp.down_proj.weight": "model-00001-of-00002.safetensors",
|
||||
"llm.model.layers.21.mlp.gate_proj.weight": "model-00001-of-00002.safetensors",
|
||||
"llm.model.layers.21.mlp.up_proj.weight": "model-00001-of-00002.safetensors",
|
||||
"llm.model.layers.21.post_attention_layernorm.weight": "model-00001-of-00002.safetensors",
|
||||
"llm.model.layers.21.self_attn.k_proj.bias": "model-00001-of-00002.safetensors",
|
||||
"llm.model.layers.21.self_attn.k_proj.weight": "model-00001-of-00002.safetensors",
|
||||
"llm.model.layers.21.self_attn.o_proj.weight": "model-00001-of-00002.safetensors",
|
||||
"llm.model.layers.21.self_attn.q_proj.bias": "model-00001-of-00002.safetensors",
|
||||
"llm.model.layers.21.self_attn.q_proj.weight": "model-00001-of-00002.safetensors",
|
||||
"llm.model.layers.21.self_attn.v_proj.bias": "model-00001-of-00002.safetensors",
|
||||
"llm.model.layers.21.self_attn.v_proj.weight": "model-00001-of-00002.safetensors",
|
||||
"llm.model.layers.22.input_layernorm.weight": "model-00001-of-00002.safetensors",
|
||||
"llm.model.layers.22.mlp.down_proj.weight": "model-00001-of-00002.safetensors",
|
||||
"llm.model.layers.22.mlp.gate_proj.weight": "model-00001-of-00002.safetensors",
|
||||
"llm.model.layers.22.mlp.up_proj.weight": "model-00001-of-00002.safetensors",
|
||||
"llm.model.layers.22.post_attention_layernorm.weight": "model-00001-of-00002.safetensors",
|
||||
"llm.model.layers.22.self_attn.k_proj.bias": "model-00001-of-00002.safetensors",
|
||||
"llm.model.layers.22.self_attn.k_proj.weight": "model-00001-of-00002.safetensors",
|
||||
"llm.model.layers.22.self_attn.o_proj.weight": "model-00001-of-00002.safetensors",
|
||||
"llm.model.layers.22.self_attn.q_proj.bias": "model-00001-of-00002.safetensors",
|
||||
"llm.model.layers.22.self_attn.q_proj.weight": "model-00001-of-00002.safetensors",
|
||||
"llm.model.layers.22.self_attn.v_proj.bias": "model-00001-of-00002.safetensors",
|
||||
"llm.model.layers.22.self_attn.v_proj.weight": "model-00001-of-00002.safetensors",
|
||||
"llm.model.layers.23.input_layernorm.weight": "model-00001-of-00002.safetensors",
|
||||
"llm.model.layers.23.mlp.down_proj.weight": "model-00001-of-00002.safetensors",
|
||||
"llm.model.layers.23.mlp.gate_proj.weight": "model-00001-of-00002.safetensors",
|
||||
"llm.model.layers.23.mlp.up_proj.weight": "model-00001-of-00002.safetensors",
|
||||
"llm.model.layers.23.post_attention_layernorm.weight": "model-00001-of-00002.safetensors",
|
||||
"llm.model.layers.23.self_attn.k_proj.bias": "model-00001-of-00002.safetensors",
|
||||
"llm.model.layers.23.self_attn.k_proj.weight": "model-00001-of-00002.safetensors",
|
||||
"llm.model.layers.23.self_attn.o_proj.weight": "model-00001-of-00002.safetensors",
|
||||
"llm.model.layers.23.self_attn.q_proj.bias": "model-00001-of-00002.safetensors",
|
||||
"llm.model.layers.23.self_attn.q_proj.weight": "model-00001-of-00002.safetensors",
|
||||
"llm.model.layers.23.self_attn.v_proj.bias": "model-00001-of-00002.safetensors",
|
||||
"llm.model.layers.23.self_attn.v_proj.weight": "model-00001-of-00002.safetensors",
|
||||
"llm.model.layers.24.input_layernorm.weight": "model-00001-of-00002.safetensors",
|
||||
"llm.model.layers.24.mlp.down_proj.weight": "model-00001-of-00002.safetensors",
|
||||
"llm.model.layers.24.mlp.gate_proj.weight": "model-00001-of-00002.safetensors",
|
||||
"llm.model.layers.24.mlp.up_proj.weight": "model-00001-of-00002.safetensors",
|
||||
"llm.model.layers.24.post_attention_layernorm.weight": "model-00001-of-00002.safetensors",
|
||||
"llm.model.layers.24.self_attn.k_proj.bias": "model-00001-of-00002.safetensors",
|
||||
"llm.model.layers.24.self_attn.k_proj.weight": "model-00001-of-00002.safetensors",
|
||||
"llm.model.layers.24.self_attn.o_proj.weight": "model-00001-of-00002.safetensors",
|
||||
"llm.model.layers.24.self_attn.q_proj.bias": "model-00001-of-00002.safetensors",
|
||||
"llm.model.layers.24.self_attn.q_proj.weight": "model-00001-of-00002.safetensors",
|
||||
"llm.model.layers.24.self_attn.v_proj.bias": "model-00001-of-00002.safetensors",
|
||||
"llm.model.layers.24.self_attn.v_proj.weight": "model-00001-of-00002.safetensors",
|
||||
"llm.model.layers.25.input_layernorm.weight": "model-00001-of-00002.safetensors",
|
||||
"llm.model.layers.25.mlp.down_proj.weight": "model-00001-of-00002.safetensors",
|
||||
"llm.model.layers.25.mlp.gate_proj.weight": "model-00001-of-00002.safetensors",
|
||||
"llm.model.layers.25.mlp.up_proj.weight": "model-00001-of-00002.safetensors",
|
||||
"llm.model.layers.25.post_attention_layernorm.weight": "model-00001-of-00002.safetensors",
|
||||
"llm.model.layers.25.self_attn.k_proj.bias": "model-00001-of-00002.safetensors",
|
||||
"llm.model.layers.25.self_attn.k_proj.weight": "model-00001-of-00002.safetensors",
|
||||
"llm.model.layers.25.self_attn.o_proj.weight": "model-00001-of-00002.safetensors",
|
||||
"llm.model.layers.25.self_attn.q_proj.bias": "model-00001-of-00002.safetensors",
|
||||
"llm.model.layers.25.self_attn.q_proj.weight": "model-00001-of-00002.safetensors",
|
||||
"llm.model.layers.25.self_attn.v_proj.bias": "model-00001-of-00002.safetensors",
|
||||
"llm.model.layers.25.self_attn.v_proj.weight": "model-00001-of-00002.safetensors",
|
||||
"llm.model.layers.26.input_layernorm.weight": "model-00001-of-00002.safetensors",
|
||||
"llm.model.layers.26.mlp.down_proj.weight": "model-00001-of-00002.safetensors",
|
||||
"llm.model.layers.26.mlp.gate_proj.weight": "model-00001-of-00002.safetensors",
|
||||
"llm.model.layers.26.mlp.up_proj.weight": "model-00001-of-00002.safetensors",
|
||||
"llm.model.layers.26.post_attention_layernorm.weight": "model-00001-of-00002.safetensors",
|
||||
"llm.model.layers.26.self_attn.k_proj.bias": "model-00001-of-00002.safetensors",
|
||||
"llm.model.layers.26.self_attn.k_proj.weight": "model-00001-of-00002.safetensors",
|
||||
"llm.model.layers.26.self_attn.o_proj.weight": "model-00001-of-00002.safetensors",
|
||||
"llm.model.layers.26.self_attn.q_proj.bias": "model-00001-of-00002.safetensors",
|
||||
"llm.model.layers.26.self_attn.q_proj.weight": "model-00001-of-00002.safetensors",
|
||||
"llm.model.layers.26.self_attn.v_proj.bias": "model-00001-of-00002.safetensors",
|
||||
"llm.model.layers.26.self_attn.v_proj.weight": "model-00001-of-00002.safetensors",
|
||||
"llm.model.layers.27.input_layernorm.weight": "model-00001-of-00002.safetensors",
|
||||
"llm.model.layers.27.mlp.down_proj.weight": "model-00001-of-00002.safetensors",
|
||||
"llm.model.layers.27.mlp.gate_proj.weight": "model-00001-of-00002.safetensors",
|
||||
"llm.model.layers.27.mlp.up_proj.weight": "model-00001-of-00002.safetensors",
|
||||
"llm.model.layers.27.post_attention_layernorm.weight": "model-00001-of-00002.safetensors",
|
||||
"llm.model.layers.27.self_attn.k_proj.bias": "model-00001-of-00002.safetensors",
|
||||
"llm.model.layers.27.self_attn.k_proj.weight": "model-00001-of-00002.safetensors",
|
||||
"llm.model.layers.27.self_attn.o_proj.weight": "model-00001-of-00002.safetensors",
|
||||
"llm.model.layers.27.self_attn.q_proj.bias": "model-00001-of-00002.safetensors",
|
||||
"llm.model.layers.27.self_attn.q_proj.weight": "model-00001-of-00002.safetensors",
|
||||
"llm.model.layers.27.self_attn.v_proj.bias": "model-00001-of-00002.safetensors",
|
||||
"llm.model.layers.27.self_attn.v_proj.weight": "model-00001-of-00002.safetensors",
|
||||
"llm.model.layers.28.input_layernorm.weight": "model-00002-of-00002.safetensors",
|
||||
"llm.model.layers.28.mlp.down_proj.weight": "model-00002-of-00002.safetensors",
|
||||
"llm.model.layers.28.mlp.gate_proj.weight": "model-00002-of-00002.safetensors",
|
||||
"llm.model.layers.28.mlp.up_proj.weight": "model-00002-of-00002.safetensors",
|
||||
"llm.model.layers.28.post_attention_layernorm.weight": "model-00002-of-00002.safetensors",
|
||||
"llm.model.layers.28.self_attn.k_proj.bias": "model-00001-of-00002.safetensors",
|
||||
"llm.model.layers.28.self_attn.k_proj.weight": "model-00001-of-00002.safetensors",
|
||||
"llm.model.layers.28.self_attn.o_proj.weight": "model-00001-of-00002.safetensors",
|
||||
"llm.model.layers.28.self_attn.q_proj.bias": "model-00001-of-00002.safetensors",
|
||||
"llm.model.layers.28.self_attn.q_proj.weight": "model-00001-of-00002.safetensors",
|
||||
"llm.model.layers.28.self_attn.v_proj.bias": "model-00001-of-00002.safetensors",
|
||||
"llm.model.layers.28.self_attn.v_proj.weight": "model-00001-of-00002.safetensors",
|
||||
"llm.model.layers.29.input_layernorm.weight": "model-00002-of-00002.safetensors",
|
||||
"llm.model.layers.29.mlp.down_proj.weight": "model-00002-of-00002.safetensors",
|
||||
"llm.model.layers.29.mlp.gate_proj.weight": "model-00002-of-00002.safetensors",
|
||||
"llm.model.layers.29.mlp.up_proj.weight": "model-00002-of-00002.safetensors",
|
||||
"llm.model.layers.29.post_attention_layernorm.weight": "model-00002-of-00002.safetensors",
|
||||
"llm.model.layers.29.self_attn.k_proj.bias": "model-00002-of-00002.safetensors",
|
||||
"llm.model.layers.29.self_attn.k_proj.weight": "model-00002-of-00002.safetensors",
|
||||
"llm.model.layers.29.self_attn.o_proj.weight": "model-00002-of-00002.safetensors",
|
||||
"llm.model.layers.29.self_attn.q_proj.bias": "model-00002-of-00002.safetensors",
|
||||
"llm.model.layers.29.self_attn.q_proj.weight": "model-00002-of-00002.safetensors",
|
||||
"llm.model.layers.29.self_attn.v_proj.bias": "model-00002-of-00002.safetensors",
|
||||
"llm.model.layers.29.self_attn.v_proj.weight": "model-00002-of-00002.safetensors",
|
||||
"llm.model.layers.3.input_layernorm.weight": "model-00001-of-00002.safetensors",
|
||||
"llm.model.layers.3.mlp.down_proj.weight": "model-00001-of-00002.safetensors",
|
||||
"llm.model.layers.3.mlp.gate_proj.weight": "model-00001-of-00002.safetensors",
|
||||
"llm.model.layers.3.mlp.up_proj.weight": "model-00001-of-00002.safetensors",
|
||||
"llm.model.layers.3.post_attention_layernorm.weight": "model-00001-of-00002.safetensors",
|
||||
"llm.model.layers.3.self_attn.k_proj.bias": "model-00001-of-00002.safetensors",
|
||||
"llm.model.layers.3.self_attn.k_proj.weight": "model-00001-of-00002.safetensors",
|
||||
"llm.model.layers.3.self_attn.o_proj.weight": "model-00001-of-00002.safetensors",
|
||||
"llm.model.layers.3.self_attn.q_proj.bias": "model-00001-of-00002.safetensors",
|
||||
"llm.model.layers.3.self_attn.q_proj.weight": "model-00001-of-00002.safetensors",
|
||||
"llm.model.layers.3.self_attn.v_proj.bias": "model-00001-of-00002.safetensors",
|
||||
"llm.model.layers.3.self_attn.v_proj.weight": "model-00001-of-00002.safetensors",
|
||||
"llm.model.layers.30.input_layernorm.weight": "model-00002-of-00002.safetensors",
|
||||
"llm.model.layers.30.mlp.down_proj.weight": "model-00002-of-00002.safetensors",
|
||||
"llm.model.layers.30.mlp.gate_proj.weight": "model-00002-of-00002.safetensors",
|
||||
"llm.model.layers.30.mlp.up_proj.weight": "model-00002-of-00002.safetensors",
|
||||
"llm.model.layers.30.post_attention_layernorm.weight": "model-00002-of-00002.safetensors",
|
||||
"llm.model.layers.30.self_attn.k_proj.bias": "model-00002-of-00002.safetensors",
|
||||
"llm.model.layers.30.self_attn.k_proj.weight": "model-00002-of-00002.safetensors",
|
||||
"llm.model.layers.30.self_attn.o_proj.weight": "model-00002-of-00002.safetensors",
|
||||
"llm.model.layers.30.self_attn.q_proj.bias": "model-00002-of-00002.safetensors",
|
||||
"llm.model.layers.30.self_attn.q_proj.weight": "model-00002-of-00002.safetensors",
|
||||
"llm.model.layers.30.self_attn.v_proj.bias": "model-00002-of-00002.safetensors",
|
||||
"llm.model.layers.30.self_attn.v_proj.weight": "model-00002-of-00002.safetensors",
|
||||
"llm.model.layers.31.input_layernorm.weight": "model-00002-of-00002.safetensors",
|
||||
"llm.model.layers.31.mlp.down_proj.weight": "model-00002-of-00002.safetensors",
|
||||
"llm.model.layers.31.mlp.gate_proj.weight": "model-00002-of-00002.safetensors",
|
||||
"llm.model.layers.31.mlp.up_proj.weight": "model-00002-of-00002.safetensors",
|
||||
"llm.model.layers.31.post_attention_layernorm.weight": "model-00002-of-00002.safetensors",
|
||||
"llm.model.layers.31.self_attn.k_proj.bias": "model-00002-of-00002.safetensors",
|
||||
"llm.model.layers.31.self_attn.k_proj.weight": "model-00002-of-00002.safetensors",
|
||||
"llm.model.layers.31.self_attn.o_proj.weight": "model-00002-of-00002.safetensors",
|
||||
"llm.model.layers.31.self_attn.q_proj.bias": "model-00002-of-00002.safetensors",
|
||||
"llm.model.layers.31.self_attn.q_proj.weight": "model-00002-of-00002.safetensors",
|
||||
"llm.model.layers.31.self_attn.v_proj.bias": "model-00002-of-00002.safetensors",
|
||||
"llm.model.layers.31.self_attn.v_proj.weight": "model-00002-of-00002.safetensors",
|
||||
"llm.model.layers.32.input_layernorm.weight": "model-00002-of-00002.safetensors",
|
||||
"llm.model.layers.32.mlp.down_proj.weight": "model-00002-of-00002.safetensors",
|
||||
"llm.model.layers.32.mlp.gate_proj.weight": "model-00002-of-00002.safetensors",
|
||||
"llm.model.layers.32.mlp.up_proj.weight": "model-00002-of-00002.safetensors",
|
||||
"llm.model.layers.32.post_attention_layernorm.weight": "model-00002-of-00002.safetensors",
|
||||
"llm.model.layers.32.self_attn.k_proj.bias": "model-00002-of-00002.safetensors",
|
||||
"llm.model.layers.32.self_attn.k_proj.weight": "model-00002-of-00002.safetensors",
|
||||
"llm.model.layers.32.self_attn.o_proj.weight": "model-00002-of-00002.safetensors",
|
||||
"llm.model.layers.32.self_attn.q_proj.bias": "model-00002-of-00002.safetensors",
|
||||
"llm.model.layers.32.self_attn.q_proj.weight": "model-00002-of-00002.safetensors",
|
||||
"llm.model.layers.32.self_attn.v_proj.bias": "model-00002-of-00002.safetensors",
|
||||
"llm.model.layers.32.self_attn.v_proj.weight": "model-00002-of-00002.safetensors",
|
||||
"llm.model.layers.33.input_layernorm.weight": "model-00002-of-00002.safetensors",
|
||||
"llm.model.layers.33.mlp.down_proj.weight": "model-00002-of-00002.safetensors",
|
||||
"llm.model.layers.33.mlp.gate_proj.weight": "model-00002-of-00002.safetensors",
|
||||
"llm.model.layers.33.mlp.up_proj.weight": "model-00002-of-00002.safetensors",
|
||||
"llm.model.layers.33.post_attention_layernorm.weight": "model-00002-of-00002.safetensors",
|
||||
"llm.model.layers.33.self_attn.k_proj.bias": "model-00002-of-00002.safetensors",
|
||||
"llm.model.layers.33.self_attn.k_proj.weight": "model-00002-of-00002.safetensors",
|
||||
"llm.model.layers.33.self_attn.o_proj.weight": "model-00002-of-00002.safetensors",
|
||||
"llm.model.layers.33.self_attn.q_proj.bias": "model-00002-of-00002.safetensors",
|
||||
"llm.model.layers.33.self_attn.q_proj.weight": "model-00002-of-00002.safetensors",
|
||||
"llm.model.layers.33.self_attn.v_proj.bias": "model-00002-of-00002.safetensors",
|
||||
"llm.model.layers.33.self_attn.v_proj.weight": "model-00002-of-00002.safetensors",
|
||||
"llm.model.layers.34.input_layernorm.weight": "model-00002-of-00002.safetensors",
|
||||
"llm.model.layers.34.mlp.down_proj.weight": "model-00002-of-00002.safetensors",
|
||||
"llm.model.layers.34.mlp.gate_proj.weight": "model-00002-of-00002.safetensors",
|
||||
"llm.model.layers.34.mlp.up_proj.weight": "model-00002-of-00002.safetensors",
|
||||
"llm.model.layers.34.post_attention_layernorm.weight": "model-00002-of-00002.safetensors",
|
||||
"llm.model.layers.34.self_attn.k_proj.bias": "model-00002-of-00002.safetensors",
|
||||
"llm.model.layers.34.self_attn.k_proj.weight": "model-00002-of-00002.safetensors",
|
||||
"llm.model.layers.34.self_attn.o_proj.weight": "model-00002-of-00002.safetensors",
|
||||
"llm.model.layers.34.self_attn.q_proj.bias": "model-00002-of-00002.safetensors",
|
||||
"llm.model.layers.34.self_attn.q_proj.weight": "model-00002-of-00002.safetensors",
|
||||
"llm.model.layers.34.self_attn.v_proj.bias": "model-00002-of-00002.safetensors",
|
||||
"llm.model.layers.34.self_attn.v_proj.weight": "model-00002-of-00002.safetensors",
|
||||
"llm.model.layers.35.input_layernorm.weight": "model-00002-of-00002.safetensors",
|
||||
"llm.model.layers.35.mlp.down_proj.weight": "model-00002-of-00002.safetensors",
|
||||
"llm.model.layers.35.mlp.gate_proj.weight": "model-00002-of-00002.safetensors",
|
||||
"llm.model.layers.35.mlp.up_proj.weight": "model-00002-of-00002.safetensors",
|
||||
"llm.model.layers.35.post_attention_layernorm.weight": "model-00002-of-00002.safetensors",
|
||||
"llm.model.layers.35.self_attn.k_proj.bias": "model-00002-of-00002.safetensors",
|
||||
"llm.model.layers.35.self_attn.k_proj.weight": "model-00002-of-00002.safetensors",
|
||||
"llm.model.layers.35.self_attn.o_proj.weight": "model-00002-of-00002.safetensors",
|
||||
"llm.model.layers.35.self_attn.q_proj.bias": "model-00002-of-00002.safetensors",
|
||||
"llm.model.layers.35.self_attn.q_proj.weight": "model-00002-of-00002.safetensors",
|
||||
"llm.model.layers.35.self_attn.v_proj.bias": "model-00002-of-00002.safetensors",
|
||||
"llm.model.layers.35.self_attn.v_proj.weight": "model-00002-of-00002.safetensors",
|
||||
"llm.model.layers.4.input_layernorm.weight": "model-00001-of-00002.safetensors",
|
||||
"llm.model.layers.4.mlp.down_proj.weight": "model-00001-of-00002.safetensors",
|
||||
"llm.model.layers.4.mlp.gate_proj.weight": "model-00001-of-00002.safetensors",
|
||||
"llm.model.layers.4.mlp.up_proj.weight": "model-00001-of-00002.safetensors",
|
||||
"llm.model.layers.4.post_attention_layernorm.weight": "model-00001-of-00002.safetensors",
|
||||
"llm.model.layers.4.self_attn.k_proj.bias": "model-00001-of-00002.safetensors",
|
||||
"llm.model.layers.4.self_attn.k_proj.weight": "model-00001-of-00002.safetensors",
|
||||
"llm.model.layers.4.self_attn.o_proj.weight": "model-00001-of-00002.safetensors",
|
||||
"llm.model.layers.4.self_attn.q_proj.bias": "model-00001-of-00002.safetensors",
|
||||
"llm.model.layers.4.self_attn.q_proj.weight": "model-00001-of-00002.safetensors",
|
||||
"llm.model.layers.4.self_attn.v_proj.bias": "model-00001-of-00002.safetensors",
|
||||
"llm.model.layers.4.self_attn.v_proj.weight": "model-00001-of-00002.safetensors",
|
||||
"llm.model.layers.5.input_layernorm.weight": "model-00001-of-00002.safetensors",
|
||||
"llm.model.layers.5.mlp.down_proj.weight": "model-00001-of-00002.safetensors",
|
||||
"llm.model.layers.5.mlp.gate_proj.weight": "model-00001-of-00002.safetensors",
|
||||
"llm.model.layers.5.mlp.up_proj.weight": "model-00001-of-00002.safetensors",
|
||||
"llm.model.layers.5.post_attention_layernorm.weight": "model-00001-of-00002.safetensors",
|
||||
"llm.model.layers.5.self_attn.k_proj.bias": "model-00001-of-00002.safetensors",
|
||||
"llm.model.layers.5.self_attn.k_proj.weight": "model-00001-of-00002.safetensors",
|
||||
"llm.model.layers.5.self_attn.o_proj.weight": "model-00001-of-00002.safetensors",
|
||||
"llm.model.layers.5.self_attn.q_proj.bias": "model-00001-of-00002.safetensors",
|
||||
"llm.model.layers.5.self_attn.q_proj.weight": "model-00001-of-00002.safetensors",
|
||||
"llm.model.layers.5.self_attn.v_proj.bias": "model-00001-of-00002.safetensors",
|
||||
"llm.model.layers.5.self_attn.v_proj.weight": "model-00001-of-00002.safetensors",
|
||||
"llm.model.layers.6.input_layernorm.weight": "model-00001-of-00002.safetensors",
|
||||
"llm.model.layers.6.mlp.down_proj.weight": "model-00001-of-00002.safetensors",
|
||||
"llm.model.layers.6.mlp.gate_proj.weight": "model-00001-of-00002.safetensors",
|
||||
"llm.model.layers.6.mlp.up_proj.weight": "model-00001-of-00002.safetensors",
|
||||
"llm.model.layers.6.post_attention_layernorm.weight": "model-00001-of-00002.safetensors",
|
||||
"llm.model.layers.6.self_attn.k_proj.bias": "model-00001-of-00002.safetensors",
|
||||
"llm.model.layers.6.self_attn.k_proj.weight": "model-00001-of-00002.safetensors",
|
||||
"llm.model.layers.6.self_attn.o_proj.weight": "model-00001-of-00002.safetensors",
|
||||
"llm.model.layers.6.self_attn.q_proj.bias": "model-00001-of-00002.safetensors",
|
||||
"llm.model.layers.6.self_attn.q_proj.weight": "model-00001-of-00002.safetensors",
|
||||
"llm.model.layers.6.self_attn.v_proj.bias": "model-00001-of-00002.safetensors",
|
||||
"llm.model.layers.6.self_attn.v_proj.weight": "model-00001-of-00002.safetensors",
|
||||
"llm.model.layers.7.input_layernorm.weight": "model-00001-of-00002.safetensors",
|
||||
"llm.model.layers.7.mlp.down_proj.weight": "model-00001-of-00002.safetensors",
|
||||
"llm.model.layers.7.mlp.gate_proj.weight": "model-00001-of-00002.safetensors",
|
||||
"llm.model.layers.7.mlp.up_proj.weight": "model-00001-of-00002.safetensors",
|
||||
"llm.model.layers.7.post_attention_layernorm.weight": "model-00001-of-00002.safetensors",
|
||||
"llm.model.layers.7.self_attn.k_proj.bias": "model-00001-of-00002.safetensors",
|
||||
"llm.model.layers.7.self_attn.k_proj.weight": "model-00001-of-00002.safetensors",
|
||||
"llm.model.layers.7.self_attn.o_proj.weight": "model-00001-of-00002.safetensors",
|
||||
"llm.model.layers.7.self_attn.q_proj.bias": "model-00001-of-00002.safetensors",
|
||||
"llm.model.layers.7.self_attn.q_proj.weight": "model-00001-of-00002.safetensors",
|
||||
"llm.model.layers.7.self_attn.v_proj.bias": "model-00001-of-00002.safetensors",
|
||||
"llm.model.layers.7.self_attn.v_proj.weight": "model-00001-of-00002.safetensors",
|
||||
"llm.model.layers.8.input_layernorm.weight": "model-00001-of-00002.safetensors",
|
||||
"llm.model.layers.8.mlp.down_proj.weight": "model-00001-of-00002.safetensors",
|
||||
"llm.model.layers.8.mlp.gate_proj.weight": "model-00001-of-00002.safetensors",
|
||||
"llm.model.layers.8.mlp.up_proj.weight": "model-00001-of-00002.safetensors",
|
||||
"llm.model.layers.8.post_attention_layernorm.weight": "model-00001-of-00002.safetensors",
|
||||
"llm.model.layers.8.self_attn.k_proj.bias": "model-00001-of-00002.safetensors",
|
||||
"llm.model.layers.8.self_attn.k_proj.weight": "model-00001-of-00002.safetensors",
|
||||
"llm.model.layers.8.self_attn.o_proj.weight": "model-00001-of-00002.safetensors",
|
||||
"llm.model.layers.8.self_attn.q_proj.bias": "model-00001-of-00002.safetensors",
|
||||
"llm.model.layers.8.self_attn.q_proj.weight": "model-00001-of-00002.safetensors",
|
||||
"llm.model.layers.8.self_attn.v_proj.bias": "model-00001-of-00002.safetensors",
|
||||
"llm.model.layers.8.self_attn.v_proj.weight": "model-00001-of-00002.safetensors",
|
||||
"llm.model.layers.9.input_layernorm.weight": "model-00001-of-00002.safetensors",
|
||||
"llm.model.layers.9.mlp.down_proj.weight": "model-00001-of-00002.safetensors",
|
||||
"llm.model.layers.9.mlp.gate_proj.weight": "model-00001-of-00002.safetensors",
|
||||
"llm.model.layers.9.mlp.up_proj.weight": "model-00001-of-00002.safetensors",
|
||||
"llm.model.layers.9.post_attention_layernorm.weight": "model-00001-of-00002.safetensors",
|
||||
"llm.model.layers.9.self_attn.k_proj.bias": "model-00001-of-00002.safetensors",
|
||||
"llm.model.layers.9.self_attn.k_proj.weight": "model-00001-of-00002.safetensors",
|
||||
"llm.model.layers.9.self_attn.o_proj.weight": "model-00001-of-00002.safetensors",
|
||||
"llm.model.layers.9.self_attn.q_proj.bias": "model-00001-of-00002.safetensors",
|
||||
"llm.model.layers.9.self_attn.q_proj.weight": "model-00001-of-00002.safetensors",
|
||||
"llm.model.layers.9.self_attn.v_proj.bias": "model-00001-of-00002.safetensors",
|
||||
"llm.model.layers.9.self_attn.v_proj.weight": "model-00001-of-00002.safetensors",
|
||||
"llm.model.norm.weight": "model-00002-of-00002.safetensors",
|
||||
"visual_tokenizer.backbone.preprocessor.patchifier.norm.weight": "model-00002-of-00002.safetensors",
|
||||
"visual_tokenizer.backbone.preprocessor.patchifier.proj.bias": "model-00002-of-00002.safetensors",
|
||||
"visual_tokenizer.backbone.preprocessor.patchifier.proj.weight": "model-00002-of-00002.safetensors",
|
||||
"visual_tokenizer.backbone.preprocessor.pos_embed": "model-00002-of-00002.safetensors",
|
||||
"visual_tokenizer.backbone.trunk.blocks.0.attn.proj.weight": "model-00002-of-00002.safetensors",
|
||||
"visual_tokenizer.backbone.trunk.blocks.0.attn.qkv.weight": "model-00002-of-00002.safetensors",
|
||||
"visual_tokenizer.backbone.trunk.blocks.0.mlp.fc1.weight": "model-00002-of-00002.safetensors",
|
||||
"visual_tokenizer.backbone.trunk.blocks.0.mlp.fc2.weight": "model-00002-of-00002.safetensors",
|
||||
"visual_tokenizer.backbone.trunk.blocks.0.mlp.fc3.weight": "model-00002-of-00002.safetensors",
|
||||
"visual_tokenizer.backbone.trunk.blocks.0.norm_1.weight": "model-00002-of-00002.safetensors",
|
||||
"visual_tokenizer.backbone.trunk.blocks.0.norm_2.weight": "model-00002-of-00002.safetensors",
|
||||
"visual_tokenizer.backbone.trunk.blocks.1.attn.proj.weight": "model-00002-of-00002.safetensors",
|
||||
"visual_tokenizer.backbone.trunk.blocks.1.attn.qkv.weight": "model-00002-of-00002.safetensors",
|
||||
"visual_tokenizer.backbone.trunk.blocks.1.mlp.fc1.weight": "model-00002-of-00002.safetensors",
|
||||
"visual_tokenizer.backbone.trunk.blocks.1.mlp.fc2.weight": "model-00002-of-00002.safetensors",
|
||||
"visual_tokenizer.backbone.trunk.blocks.1.mlp.fc3.weight": "model-00002-of-00002.safetensors",
|
||||
"visual_tokenizer.backbone.trunk.blocks.1.norm_1.weight": "model-00002-of-00002.safetensors",
|
||||
"visual_tokenizer.backbone.trunk.blocks.1.norm_2.weight": "model-00002-of-00002.safetensors",
|
||||
"visual_tokenizer.backbone.trunk.blocks.10.attn.proj.weight": "model-00002-of-00002.safetensors",
|
||||
"visual_tokenizer.backbone.trunk.blocks.10.attn.qkv.weight": "model-00002-of-00002.safetensors",
|
||||
"visual_tokenizer.backbone.trunk.blocks.10.mlp.fc1.weight": "model-00002-of-00002.safetensors",
|
||||
"visual_tokenizer.backbone.trunk.blocks.10.mlp.fc2.weight": "model-00002-of-00002.safetensors",
|
||||
"visual_tokenizer.backbone.trunk.blocks.10.mlp.fc3.weight": "model-00002-of-00002.safetensors",
|
||||
"visual_tokenizer.backbone.trunk.blocks.10.norm_1.weight": "model-00002-of-00002.safetensors",
|
||||
"visual_tokenizer.backbone.trunk.blocks.10.norm_2.weight": "model-00002-of-00002.safetensors",
|
||||
"visual_tokenizer.backbone.trunk.blocks.11.attn.proj.weight": "model-00002-of-00002.safetensors",
|
||||
"visual_tokenizer.backbone.trunk.blocks.11.attn.qkv.weight": "model-00002-of-00002.safetensors",
|
||||
"visual_tokenizer.backbone.trunk.blocks.11.mlp.fc1.weight": "model-00002-of-00002.safetensors",
|
||||
"visual_tokenizer.backbone.trunk.blocks.11.mlp.fc2.weight": "model-00002-of-00002.safetensors",
|
||||
"visual_tokenizer.backbone.trunk.blocks.11.mlp.fc3.weight": "model-00002-of-00002.safetensors",
|
||||
"visual_tokenizer.backbone.trunk.blocks.11.norm_1.weight": "model-00002-of-00002.safetensors",
|
||||
"visual_tokenizer.backbone.trunk.blocks.11.norm_2.weight": "model-00002-of-00002.safetensors",
|
||||
"visual_tokenizer.backbone.trunk.blocks.12.attn.proj.weight": "model-00002-of-00002.safetensors",
|
||||
"visual_tokenizer.backbone.trunk.blocks.12.attn.qkv.weight": "model-00002-of-00002.safetensors",
|
||||
"visual_tokenizer.backbone.trunk.blocks.12.mlp.fc1.weight": "model-00002-of-00002.safetensors",
|
||||
"visual_tokenizer.backbone.trunk.blocks.12.mlp.fc2.weight": "model-00002-of-00002.safetensors",
|
||||
"visual_tokenizer.backbone.trunk.blocks.12.mlp.fc3.weight": "model-00002-of-00002.safetensors",
|
||||
"visual_tokenizer.backbone.trunk.blocks.12.norm_1.weight": "model-00002-of-00002.safetensors",
|
||||
"visual_tokenizer.backbone.trunk.blocks.12.norm_2.weight": "model-00002-of-00002.safetensors",
|
||||
"visual_tokenizer.backbone.trunk.blocks.13.attn.proj.weight": "model-00002-of-00002.safetensors",
|
||||
"visual_tokenizer.backbone.trunk.blocks.13.attn.qkv.weight": "model-00002-of-00002.safetensors",
|
||||
"visual_tokenizer.backbone.trunk.blocks.13.mlp.fc1.weight": "model-00002-of-00002.safetensors",
|
||||
"visual_tokenizer.backbone.trunk.blocks.13.mlp.fc2.weight": "model-00002-of-00002.safetensors",
|
||||
"visual_tokenizer.backbone.trunk.blocks.13.mlp.fc3.weight": "model-00002-of-00002.safetensors",
|
||||
"visual_tokenizer.backbone.trunk.blocks.13.norm_1.weight": "model-00002-of-00002.safetensors",
|
||||
"visual_tokenizer.backbone.trunk.blocks.13.norm_2.weight": "model-00002-of-00002.safetensors",
|
||||
"visual_tokenizer.backbone.trunk.blocks.14.attn.proj.weight": "model-00002-of-00002.safetensors",
|
||||
"visual_tokenizer.backbone.trunk.blocks.14.attn.qkv.weight": "model-00002-of-00002.safetensors",
|
||||
"visual_tokenizer.backbone.trunk.blocks.14.mlp.fc1.weight": "model-00002-of-00002.safetensors",
|
||||
"visual_tokenizer.backbone.trunk.blocks.14.mlp.fc2.weight": "model-00002-of-00002.safetensors",
|
||||
"visual_tokenizer.backbone.trunk.blocks.14.mlp.fc3.weight": "model-00002-of-00002.safetensors",
|
||||
"visual_tokenizer.backbone.trunk.blocks.14.norm_1.weight": "model-00002-of-00002.safetensors",
|
||||
"visual_tokenizer.backbone.trunk.blocks.14.norm_2.weight": "model-00002-of-00002.safetensors",
|
||||
"visual_tokenizer.backbone.trunk.blocks.15.attn.proj.weight": "model-00002-of-00002.safetensors",
|
||||
"visual_tokenizer.backbone.trunk.blocks.15.attn.qkv.weight": "model-00002-of-00002.safetensors",
|
||||
"visual_tokenizer.backbone.trunk.blocks.15.mlp.fc1.weight": "model-00002-of-00002.safetensors",
|
||||
"visual_tokenizer.backbone.trunk.blocks.15.mlp.fc2.weight": "model-00002-of-00002.safetensors",
|
||||
"visual_tokenizer.backbone.trunk.blocks.15.mlp.fc3.weight": "model-00002-of-00002.safetensors",
|
||||
"visual_tokenizer.backbone.trunk.blocks.15.norm_1.weight": "model-00002-of-00002.safetensors",
|
||||
"visual_tokenizer.backbone.trunk.blocks.15.norm_2.weight": "model-00002-of-00002.safetensors",
|
||||
"visual_tokenizer.backbone.trunk.blocks.16.attn.proj.weight": "model-00002-of-00002.safetensors",
|
||||
"visual_tokenizer.backbone.trunk.blocks.16.attn.qkv.weight": "model-00002-of-00002.safetensors",
|
||||
"visual_tokenizer.backbone.trunk.blocks.16.mlp.fc1.weight": "model-00002-of-00002.safetensors",
|
||||
"visual_tokenizer.backbone.trunk.blocks.16.mlp.fc2.weight": "model-00002-of-00002.safetensors",
|
||||
"visual_tokenizer.backbone.trunk.blocks.16.mlp.fc3.weight": "model-00002-of-00002.safetensors",
|
||||
"visual_tokenizer.backbone.trunk.blocks.16.norm_1.weight": "model-00002-of-00002.safetensors",
|
||||
"visual_tokenizer.backbone.trunk.blocks.16.norm_2.weight": "model-00002-of-00002.safetensors",
|
||||
"visual_tokenizer.backbone.trunk.blocks.17.attn.proj.weight": "model-00002-of-00002.safetensors",
|
||||
"visual_tokenizer.backbone.trunk.blocks.17.attn.qkv.weight": "model-00002-of-00002.safetensors",
|
||||
"visual_tokenizer.backbone.trunk.blocks.17.mlp.fc1.weight": "model-00002-of-00002.safetensors",
|
||||
"visual_tokenizer.backbone.trunk.blocks.17.mlp.fc2.weight": "model-00002-of-00002.safetensors",
|
||||
"visual_tokenizer.backbone.trunk.blocks.17.mlp.fc3.weight": "model-00002-of-00002.safetensors",
|
||||
"visual_tokenizer.backbone.trunk.blocks.17.norm_1.weight": "model-00002-of-00002.safetensors",
|
||||
"visual_tokenizer.backbone.trunk.blocks.17.norm_2.weight": "model-00002-of-00002.safetensors",
|
||||
"visual_tokenizer.backbone.trunk.blocks.18.attn.proj.weight": "model-00002-of-00002.safetensors",
|
||||
"visual_tokenizer.backbone.trunk.blocks.18.attn.qkv.weight": "model-00002-of-00002.safetensors",
|
||||
"visual_tokenizer.backbone.trunk.blocks.18.mlp.fc1.weight": "model-00002-of-00002.safetensors",
|
||||
"visual_tokenizer.backbone.trunk.blocks.18.mlp.fc2.weight": "model-00002-of-00002.safetensors",
|
||||
"visual_tokenizer.backbone.trunk.blocks.18.mlp.fc3.weight": "model-00002-of-00002.safetensors",
|
||||
"visual_tokenizer.backbone.trunk.blocks.18.norm_1.weight": "model-00002-of-00002.safetensors",
|
||||
"visual_tokenizer.backbone.trunk.blocks.18.norm_2.weight": "model-00002-of-00002.safetensors",
|
||||
"visual_tokenizer.backbone.trunk.blocks.19.attn.proj.weight": "model-00002-of-00002.safetensors",
|
||||
"visual_tokenizer.backbone.trunk.blocks.19.attn.qkv.weight": "model-00002-of-00002.safetensors",
|
||||
"visual_tokenizer.backbone.trunk.blocks.19.mlp.fc1.weight": "model-00002-of-00002.safetensors",
|
||||
"visual_tokenizer.backbone.trunk.blocks.19.mlp.fc2.weight": "model-00002-of-00002.safetensors",
|
||||
"visual_tokenizer.backbone.trunk.blocks.19.mlp.fc3.weight": "model-00002-of-00002.safetensors",
|
||||
"visual_tokenizer.backbone.trunk.blocks.19.norm_1.weight": "model-00002-of-00002.safetensors",
|
||||
"visual_tokenizer.backbone.trunk.blocks.19.norm_2.weight": "model-00002-of-00002.safetensors",
|
||||
"visual_tokenizer.backbone.trunk.blocks.2.attn.proj.weight": "model-00002-of-00002.safetensors",
|
||||
"visual_tokenizer.backbone.trunk.blocks.2.attn.qkv.weight": "model-00002-of-00002.safetensors",
|
||||
"visual_tokenizer.backbone.trunk.blocks.2.mlp.fc1.weight": "model-00002-of-00002.safetensors",
|
||||
"visual_tokenizer.backbone.trunk.blocks.2.mlp.fc2.weight": "model-00002-of-00002.safetensors",
|
||||
"visual_tokenizer.backbone.trunk.blocks.2.mlp.fc3.weight": "model-00002-of-00002.safetensors",
|
||||
"visual_tokenizer.backbone.trunk.blocks.2.norm_1.weight": "model-00002-of-00002.safetensors",
|
||||
"visual_tokenizer.backbone.trunk.blocks.2.norm_2.weight": "model-00002-of-00002.safetensors",
|
||||
"visual_tokenizer.backbone.trunk.blocks.20.attn.proj.weight": "model-00002-of-00002.safetensors",
|
||||
"visual_tokenizer.backbone.trunk.blocks.20.attn.qkv.weight": "model-00002-of-00002.safetensors",
|
||||
"visual_tokenizer.backbone.trunk.blocks.20.mlp.fc1.weight": "model-00002-of-00002.safetensors",
|
||||
"visual_tokenizer.backbone.trunk.blocks.20.mlp.fc2.weight": "model-00002-of-00002.safetensors",
|
||||
"visual_tokenizer.backbone.trunk.blocks.20.mlp.fc3.weight": "model-00002-of-00002.safetensors",
|
||||
"visual_tokenizer.backbone.trunk.blocks.20.norm_1.weight": "model-00002-of-00002.safetensors",
|
||||
"visual_tokenizer.backbone.trunk.blocks.20.norm_2.weight": "model-00002-of-00002.safetensors",
|
||||
"visual_tokenizer.backbone.trunk.blocks.21.attn.proj.weight": "model-00002-of-00002.safetensors",
|
||||
"visual_tokenizer.backbone.trunk.blocks.21.attn.qkv.weight": "model-00002-of-00002.safetensors",
|
||||
"visual_tokenizer.backbone.trunk.blocks.21.mlp.fc1.weight": "model-00002-of-00002.safetensors",
|
||||
"visual_tokenizer.backbone.trunk.blocks.21.mlp.fc2.weight": "model-00002-of-00002.safetensors",
|
||||
"visual_tokenizer.backbone.trunk.blocks.21.mlp.fc3.weight": "model-00002-of-00002.safetensors",
|
||||
"visual_tokenizer.backbone.trunk.blocks.21.norm_1.weight": "model-00002-of-00002.safetensors",
|
||||
"visual_tokenizer.backbone.trunk.blocks.21.norm_2.weight": "model-00002-of-00002.safetensors",
|
||||
"visual_tokenizer.backbone.trunk.blocks.22.attn.proj.weight": "model-00002-of-00002.safetensors",
|
||||
"visual_tokenizer.backbone.trunk.blocks.22.attn.qkv.weight": "model-00002-of-00002.safetensors",
|
||||
"visual_tokenizer.backbone.trunk.blocks.22.mlp.fc1.weight": "model-00002-of-00002.safetensors",
|
||||
"visual_tokenizer.backbone.trunk.blocks.22.mlp.fc2.weight": "model-00002-of-00002.safetensors",
|
||||
"visual_tokenizer.backbone.trunk.blocks.22.mlp.fc3.weight": "model-00002-of-00002.safetensors",
|
||||
"visual_tokenizer.backbone.trunk.blocks.22.norm_1.weight": "model-00002-of-00002.safetensors",
|
||||
"visual_tokenizer.backbone.trunk.blocks.22.norm_2.weight": "model-00002-of-00002.safetensors",
|
||||
"visual_tokenizer.backbone.trunk.blocks.23.attn.proj.weight": "model-00002-of-00002.safetensors",
|
||||
"visual_tokenizer.backbone.trunk.blocks.23.attn.qkv.weight": "model-00002-of-00002.safetensors",
|
||||
"visual_tokenizer.backbone.trunk.blocks.23.mlp.fc1.weight": "model-00002-of-00002.safetensors",
|
||||
"visual_tokenizer.backbone.trunk.blocks.23.mlp.fc2.weight": "model-00002-of-00002.safetensors",
|
||||
"visual_tokenizer.backbone.trunk.blocks.23.mlp.fc3.weight": "model-00002-of-00002.safetensors",
|
||||
"visual_tokenizer.backbone.trunk.blocks.23.norm_1.weight": "model-00002-of-00002.safetensors",
|
||||
"visual_tokenizer.backbone.trunk.blocks.23.norm_2.weight": "model-00002-of-00002.safetensors",
|
||||
"visual_tokenizer.backbone.trunk.blocks.3.attn.proj.weight": "model-00002-of-00002.safetensors",
|
||||
"visual_tokenizer.backbone.trunk.blocks.3.attn.qkv.weight": "model-00002-of-00002.safetensors",
|
||||
"visual_tokenizer.backbone.trunk.blocks.3.mlp.fc1.weight": "model-00002-of-00002.safetensors",
|
||||
"visual_tokenizer.backbone.trunk.blocks.3.mlp.fc2.weight": "model-00002-of-00002.safetensors",
|
||||
"visual_tokenizer.backbone.trunk.blocks.3.mlp.fc3.weight": "model-00002-of-00002.safetensors",
|
||||
"visual_tokenizer.backbone.trunk.blocks.3.norm_1.weight": "model-00002-of-00002.safetensors",
|
||||
"visual_tokenizer.backbone.trunk.blocks.3.norm_2.weight": "model-00002-of-00002.safetensors",
|
||||
"visual_tokenizer.backbone.trunk.blocks.4.attn.proj.weight": "model-00002-of-00002.safetensors",
|
||||
"visual_tokenizer.backbone.trunk.blocks.4.attn.qkv.weight": "model-00002-of-00002.safetensors",
|
||||
"visual_tokenizer.backbone.trunk.blocks.4.mlp.fc1.weight": "model-00002-of-00002.safetensors",
|
||||
"visual_tokenizer.backbone.trunk.blocks.4.mlp.fc2.weight": "model-00002-of-00002.safetensors",
|
||||
"visual_tokenizer.backbone.trunk.blocks.4.mlp.fc3.weight": "model-00002-of-00002.safetensors",
|
||||
"visual_tokenizer.backbone.trunk.blocks.4.norm_1.weight": "model-00002-of-00002.safetensors",
|
||||
"visual_tokenizer.backbone.trunk.blocks.4.norm_2.weight": "model-00002-of-00002.safetensors",
|
||||
"visual_tokenizer.backbone.trunk.blocks.5.attn.proj.weight": "model-00002-of-00002.safetensors",
|
||||
"visual_tokenizer.backbone.trunk.blocks.5.attn.qkv.weight": "model-00002-of-00002.safetensors",
|
||||
"visual_tokenizer.backbone.trunk.blocks.5.mlp.fc1.weight": "model-00002-of-00002.safetensors",
|
||||
"visual_tokenizer.backbone.trunk.blocks.5.mlp.fc2.weight": "model-00002-of-00002.safetensors",
|
||||
"visual_tokenizer.backbone.trunk.blocks.5.mlp.fc3.weight": "model-00002-of-00002.safetensors",
|
||||
"visual_tokenizer.backbone.trunk.blocks.5.norm_1.weight": "model-00002-of-00002.safetensors",
|
||||
"visual_tokenizer.backbone.trunk.blocks.5.norm_2.weight": "model-00002-of-00002.safetensors",
|
||||
"visual_tokenizer.backbone.trunk.blocks.6.attn.proj.weight": "model-00002-of-00002.safetensors",
|
||||
"visual_tokenizer.backbone.trunk.blocks.6.attn.qkv.weight": "model-00002-of-00002.safetensors",
|
||||
"visual_tokenizer.backbone.trunk.blocks.6.mlp.fc1.weight": "model-00002-of-00002.safetensors",
|
||||
"visual_tokenizer.backbone.trunk.blocks.6.mlp.fc2.weight": "model-00002-of-00002.safetensors",
|
||||
"visual_tokenizer.backbone.trunk.blocks.6.mlp.fc3.weight": "model-00002-of-00002.safetensors",
|
||||
"visual_tokenizer.backbone.trunk.blocks.6.norm_1.weight": "model-00002-of-00002.safetensors",
|
||||
"visual_tokenizer.backbone.trunk.blocks.6.norm_2.weight": "model-00002-of-00002.safetensors",
|
||||
"visual_tokenizer.backbone.trunk.blocks.7.attn.proj.weight": "model-00002-of-00002.safetensors",
|
||||
"visual_tokenizer.backbone.trunk.blocks.7.attn.qkv.weight": "model-00002-of-00002.safetensors",
|
||||
"visual_tokenizer.backbone.trunk.blocks.7.mlp.fc1.weight": "model-00002-of-00002.safetensors",
|
||||
"visual_tokenizer.backbone.trunk.blocks.7.mlp.fc2.weight": "model-00002-of-00002.safetensors",
|
||||
"visual_tokenizer.backbone.trunk.blocks.7.mlp.fc3.weight": "model-00002-of-00002.safetensors",
|
||||
"visual_tokenizer.backbone.trunk.blocks.7.norm_1.weight": "model-00002-of-00002.safetensors",
|
||||
"visual_tokenizer.backbone.trunk.blocks.7.norm_2.weight": "model-00002-of-00002.safetensors",
|
||||
"visual_tokenizer.backbone.trunk.blocks.8.attn.proj.weight": "model-00002-of-00002.safetensors",
|
||||
"visual_tokenizer.backbone.trunk.blocks.8.attn.qkv.weight": "model-00002-of-00002.safetensors",
|
||||
"visual_tokenizer.backbone.trunk.blocks.8.mlp.fc1.weight": "model-00002-of-00002.safetensors",
|
||||
"visual_tokenizer.backbone.trunk.blocks.8.mlp.fc2.weight": "model-00002-of-00002.safetensors",
|
||||
"visual_tokenizer.backbone.trunk.blocks.8.mlp.fc3.weight": "model-00002-of-00002.safetensors",
|
||||
"visual_tokenizer.backbone.trunk.blocks.8.norm_1.weight": "model-00002-of-00002.safetensors",
|
||||
"visual_tokenizer.backbone.trunk.blocks.8.norm_2.weight": "model-00002-of-00002.safetensors",
|
||||
"visual_tokenizer.backbone.trunk.blocks.9.attn.proj.weight": "model-00002-of-00002.safetensors",
|
||||
"visual_tokenizer.backbone.trunk.blocks.9.attn.qkv.weight": "model-00002-of-00002.safetensors",
|
||||
"visual_tokenizer.backbone.trunk.blocks.9.mlp.fc1.weight": "model-00002-of-00002.safetensors",
|
||||
"visual_tokenizer.backbone.trunk.blocks.9.mlp.fc2.weight": "model-00002-of-00002.safetensors",
|
||||
"visual_tokenizer.backbone.trunk.blocks.9.mlp.fc3.weight": "model-00002-of-00002.safetensors",
|
||||
"visual_tokenizer.backbone.trunk.blocks.9.norm_1.weight": "model-00002-of-00002.safetensors",
|
||||
"visual_tokenizer.backbone.trunk.blocks.9.norm_2.weight": "model-00002-of-00002.safetensors",
|
||||
"visual_tokenizer.backbone.trunk.post_trunk_norm.weight": "model-00002-of-00002.safetensors",
|
||||
"visual_tokenizer.head.0.weight": "model-00002-of-00002.safetensors",
|
||||
"visual_tokenizer.head.1.bias": "model-00002-of-00002.safetensors",
|
||||
"visual_tokenizer.head.1.weight": "model-00002-of-00002.safetensors",
|
||||
"vte.weight": "model-00002-of-00002.safetensors"
|
||||
}
|
||||
}
|
|
@ -0,0 +1,198 @@
|
|||
# adapted from https://huggingface.co/apple/aimv2-huge-patch14-448 (modification: add gradient checkpoint support)
|
||||
from typing import Optional, Tuple, Union
|
||||
|
||||
import torch
|
||||
from .configuration_aimv2 import AIMv2Config
|
||||
from torch import nn
|
||||
from torch.nn import functional as F
|
||||
from transformers.modeling_outputs import BaseModelOutputWithNoAttention
|
||||
from transformers.modeling_utils import PreTrainedModel
|
||||
|
||||
__all__ = ["AIMv2Model"]
|
||||
|
||||
|
||||
class RMSNorm(nn.Module):
|
||||
def __init__(self, dim: int, eps: float = 1e-6):
|
||||
super().__init__()
|
||||
self.weight = nn.Parameter(torch.ones(dim))
|
||||
self.eps = eps
|
||||
|
||||
def forward(self, x: torch.Tensor) -> torch.Tensor:
|
||||
output = self._norm(x.float()).type_as(x)
|
||||
return output * self.weight
|
||||
|
||||
def extra_repr(self) -> str:
|
||||
return f"{tuple(self.weight.shape)}, eps={self.eps}"
|
||||
|
||||
def _norm(self, x: torch.Tensor) -> torch.Tensor:
|
||||
return x * torch.rsqrt(x.pow(2).mean(-1, keepdim=True) + self.eps)
|
||||
|
||||
|
||||
class AIMv2SwiGLUFFN(nn.Module):
|
||||
def __init__(self, config: AIMv2Config):
|
||||
super().__init__()
|
||||
hidden_features = config.intermediate_size
|
||||
in_features = config.hidden_size
|
||||
bias = config.use_bias
|
||||
|
||||
self.fc1 = nn.Linear(in_features, hidden_features, bias=bias)
|
||||
self.fc2 = nn.Linear(hidden_features, in_features, bias=bias)
|
||||
self.fc3 = nn.Linear(in_features, hidden_features, bias=bias)
|
||||
|
||||
def forward(self, x: torch.Tensor) -> torch.Tensor:
|
||||
x = F.silu(self.fc1(x)) * self.fc3(x)
|
||||
x = self.fc2(x)
|
||||
return x
|
||||
|
||||
|
||||
class AIMv2PatchEmbed(nn.Module):
|
||||
def __init__(self, config: AIMv2Config):
|
||||
super().__init__()
|
||||
self.proj = nn.Conv2d(
|
||||
config.num_channels,
|
||||
config.hidden_size,
|
||||
kernel_size=(config.patch_size, config.patch_size),
|
||||
stride=(config.patch_size, config.patch_size),
|
||||
)
|
||||
self.norm = RMSNorm(config.hidden_size, eps=config.rms_norm_eps)
|
||||
|
||||
def forward(self, x: torch.Tensor) -> torch.Tensor:
|
||||
x = self.proj(x).flatten(2).transpose(1, 2)
|
||||
x = self.norm(x)
|
||||
return x
|
||||
|
||||
|
||||
class AIMv2ViTPreprocessor(nn.Module):
|
||||
def __init__(self, config: AIMv2Config):
|
||||
super().__init__()
|
||||
num_patches = (config.image_size // config.patch_size) ** 2
|
||||
|
||||
self.patchifier = AIMv2PatchEmbed(config)
|
||||
self.pos_embed = nn.Parameter(torch.zeros((1, num_patches, config.hidden_size)))
|
||||
|
||||
def forward(self, x: torch.Tensor) -> torch.Tensor:
|
||||
tokens = self.patchifier(x)
|
||||
_, N, _ = tokens.shape
|
||||
pos_embed = self.pos_embed.to(tokens.device)
|
||||
tokens = tokens + pos_embed[:, :N]
|
||||
return tokens
|
||||
|
||||
|
||||
class AIMv2Attention(nn.Module):
|
||||
def __init__(self, config: AIMv2Config):
|
||||
super().__init__()
|
||||
dim = config.hidden_size
|
||||
|
||||
self.num_heads = config.num_attention_heads
|
||||
self.qkv = nn.Linear(dim, dim * 3, bias=config.qkv_bias)
|
||||
self.attn_drop = nn.Dropout(config.attention_dropout)
|
||||
self.proj = nn.Linear(dim, dim, bias=config.use_bias)
|
||||
self.proj_drop = nn.Dropout(config.projection_dropout)
|
||||
|
||||
def forward(
|
||||
self, x: torch.Tensor, mask: Optional[torch.Tensor] = None
|
||||
) -> torch.Tensor:
|
||||
B, N, C = x.shape
|
||||
qkv = (
|
||||
self.qkv(x)
|
||||
.reshape(B, N, 3, self.num_heads, C // self.num_heads)
|
||||
.permute(2, 0, 3, 1, 4)
|
||||
)
|
||||
q, k, v = qkv.unbind(0)
|
||||
|
||||
x = F.scaled_dot_product_attention(q, k, v, attn_mask=mask)
|
||||
x = x.transpose(1, 2).contiguous().reshape(B, N, C)
|
||||
x = self.proj(x)
|
||||
x = self.proj_drop(x)
|
||||
return x
|
||||
|
||||
|
||||
class AIMv2Block(nn.Module):
|
||||
def __init__(self, config: AIMv2Config):
|
||||
super().__init__()
|
||||
self.attn = AIMv2Attention(config)
|
||||
self.norm_1 = RMSNorm(config.hidden_size, eps=config.rms_norm_eps)
|
||||
self.mlp = AIMv2SwiGLUFFN(config)
|
||||
self.norm_2 = RMSNorm(config.hidden_size, eps=config.rms_norm_eps)
|
||||
|
||||
def forward(
|
||||
self, x: torch.Tensor, mask: Optional[torch.Tensor] = None
|
||||
) -> torch.Tensor:
|
||||
x = x + self.attn(self.norm_1(x), mask)
|
||||
x = x + self.mlp(self.norm_2(x))
|
||||
return x
|
||||
|
||||
|
||||
class AIMv2Transformer(nn.Module):
|
||||
def __init__(self, config: AIMv2Config):
|
||||
super().__init__()
|
||||
self.blocks = nn.ModuleList(
|
||||
[AIMv2Block(config) for _ in range(config.num_hidden_layers)]
|
||||
)
|
||||
self.post_trunk_norm = RMSNorm(config.hidden_size, eps=config.rms_norm_eps)
|
||||
self.gradient_checkpointing = False
|
||||
|
||||
def forward(
|
||||
self,
|
||||
tokens: torch.Tensor,
|
||||
mask: Optional[torch.Tensor] = None,
|
||||
output_hidden_states: bool = False,
|
||||
) -> Tuple[torch.Tensor, Optional[Tuple[torch.Tensor, ...]]]:
|
||||
hidden_states = () if output_hidden_states else None
|
||||
for block in self.blocks:
|
||||
if self.gradient_checkpointing and self.training:
|
||||
tokens = self._gradient_checkpointing_func(block.__call__, tokens, mask)
|
||||
else:
|
||||
tokens = block(tokens, mask)
|
||||
if output_hidden_states:
|
||||
hidden_states += (tokens,)
|
||||
tokens = self.post_trunk_norm(tokens)
|
||||
return tokens, hidden_states
|
||||
|
||||
|
||||
class AIMv2PretrainedModel(PreTrainedModel):
|
||||
config_class = AIMv2Config
|
||||
base_model_prefix = "aimv2"
|
||||
supports_gradient_checkpointing = True
|
||||
main_input_name = "pixel_values"
|
||||
_no_split_modules = ["AIMv2ViTPreprocessor", "AIMv2Block"]
|
||||
_supports_sdpa = True
|
||||
|
||||
|
||||
class AIMv2Model(AIMv2PretrainedModel):
|
||||
def __init__(self, config: AIMv2Config):
|
||||
super().__init__(config)
|
||||
self.preprocessor = AIMv2ViTPreprocessor(config)
|
||||
self.trunk = AIMv2Transformer(config)
|
||||
|
||||
def forward(
|
||||
self,
|
||||
pixel_values: torch.Tensor,
|
||||
mask: Optional[torch.Tensor] = None,
|
||||
output_hidden_states: Optional[bool] = None,
|
||||
return_dict: Optional[bool] = None,
|
||||
) -> Union[
|
||||
Tuple[torch.Tensor],
|
||||
Tuple[torch.Tensor, Tuple[torch.Tensor, ...]],
|
||||
BaseModelOutputWithNoAttention,
|
||||
]:
|
||||
if output_hidden_states is None:
|
||||
output_hidden_states = self.config.output_hidden_states
|
||||
if return_dict is None:
|
||||
return_dict = self.config.use_return_dict
|
||||
|
||||
x = self.preprocessor(pixel_values)
|
||||
x, hidden_states = self.trunk(
|
||||
x, mask, output_hidden_states=output_hidden_states
|
||||
)
|
||||
|
||||
if not return_dict:
|
||||
res = (x,)
|
||||
res += (hidden_states,) if output_hidden_states else ()
|
||||
return res
|
||||
|
||||
return BaseModelOutputWithNoAttention(
|
||||
last_hidden_state=x,
|
||||
hidden_states=hidden_states,
|
||||
)
|
||||
|
|
@ -0,0 +1,590 @@
|
|||
# Copyright (C) 2025 AIDC-AI
|
||||
#
|
||||
# Licensed under the Apache License, Version 2.0 (the "License");
|
||||
# you may not use this file except in compliance with the License.
|
||||
# You may obtain a copy of the License at
|
||||
# http://www.apache.org/licenses/LICENSE-2.0
|
||||
#
|
||||
# Unless required by applicable law or agreed to in writing, software
|
||||
# distributed under the License is distributed on an "AS IS" BASIS,
|
||||
# WITHOUT WARRANTIES OR CONDITIONS OF ANY KIND, either express or implied.
|
||||
#
|
||||
# See the License for the specific language governing permissions and
|
||||
# limitations under the License.
|
||||
|
||||
import logging
|
||||
import os
|
||||
import importlib.metadata
|
||||
|
||||
from packaging import version
|
||||
from importlib import import_module
|
||||
from typing import List, Callable, Union, Optional, Dict
|
||||
|
||||
import PIL.Image
|
||||
import torch
|
||||
from torch import Tensor
|
||||
from torch.nn import init
|
||||
from torch.nn.functional import softmax, gumbel_softmax, pad
|
||||
from transformers.utils import is_flash_attn_2_available
|
||||
from transformers import PreTrainedModel, AutoModel, AutoTokenizer, AutoModelForCausalLM, AutoImageProcessor
|
||||
from transformers.generation.utils import GenerateOutput
|
||||
|
||||
from .configuration_ovis import BaseVisualTokenizerConfig, Aimv2VisualTokenizerConfig
|
||||
from .configuration_ovis import OvisConfig, ConversationFormatter
|
||||
from .configuration_ovis import IGNORE_ID, IMAGE_ATOM_ID, IMAGE_INDICATOR_IDS, IMAGE_TOKEN_ID
|
||||
|
||||
# ----------------------------------------------------------------------
|
||||
# Visual Tokenizer
|
||||
# ----------------------------------------------------------------------
|
||||
class BaseVisualTokenizer(PreTrainedModel):
|
||||
base_model_prefix = "backbone"
|
||||
main_input_name = None
|
||||
_image_processor_class = None
|
||||
_image_processor_kwargs = {}
|
||||
_backbone_class = None
|
||||
_backbone_name_or_path = None
|
||||
|
||||
def __init__(self, config: BaseVisualTokenizerConfig, *inputs, **kwargs):
|
||||
super().__init__(config, *inputs, **kwargs)
|
||||
self.image_processor = AutoImageProcessor.from_pretrained(kwargs['image_processor_name_or_path'])
|
||||
self.backbone = AutoModel.from_config(self.config.backbone_config)
|
||||
head_dim = self.config.vocab_size - len(IMAGE_INDICATOR_IDS) # reserved tokens for IMAGE_INDICATORS
|
||||
self.head = torch.nn.Sequential(
|
||||
torch.nn.Linear(
|
||||
self.backbone.config.hidden_size * self.config.hidden_stride * self.config.hidden_stride, head_dim,
|
||||
bias=False
|
||||
),
|
||||
torch.nn.LayerNorm(head_dim)
|
||||
)
|
||||
|
||||
assert all((self.image_processor.do_resize,
|
||||
not getattr(self.image_processor, 'do_center_crop', False),
|
||||
self.image_processor.do_rescale,
|
||||
self.image_processor.do_normalize
|
||||
)), f"image_processor `{self.image_processor}` is not supported currently"
|
||||
|
||||
def get_backbone(self):
|
||||
return self.backbone
|
||||
|
||||
def get_image_processor(self):
|
||||
return self.image_processor
|
||||
|
||||
def mock_input(self):
|
||||
height, width = self.get_image_size()
|
||||
return torch.zeros(1, 3, height, width), self.construct_image_placeholders((1, 1))
|
||||
|
||||
def get_head(self):
|
||||
return self.head
|
||||
|
||||
def get_image_size(self):
|
||||
raise NotImplementedError
|
||||
|
||||
@staticmethod
|
||||
def construct_image_placeholders(grid):
|
||||
image_placeholders = [IMAGE_INDICATOR_IDS[0], IMAGE_ATOM_ID, IMAGE_INDICATOR_IDS[1]]
|
||||
if grid[0] * grid[1] > 1:
|
||||
for r in range(grid[0]):
|
||||
for c in range(grid[1]):
|
||||
image_placeholders.append(IMAGE_ATOM_ID)
|
||||
if c < grid[1] - 1:
|
||||
image_placeholders.append(IMAGE_INDICATOR_IDS[2])
|
||||
if r < grid[0] - 1:
|
||||
image_placeholders.append(IMAGE_INDICATOR_IDS[3])
|
||||
image_placeholders.append(IMAGE_INDICATOR_IDS[4])
|
||||
return image_placeholders
|
||||
|
||||
def preprocess_image(self, image: PIL.Image.Image, max_partition=9, covering_threshold=0.9, convert_to_rgb=True):
|
||||
def _preprocess(img: PIL.Image.Image, side):
|
||||
# first resize and preprocess
|
||||
w, h = img.size
|
||||
if w == h:
|
||||
new_width = new_height = side
|
||||
elif w > h:
|
||||
new_width = side
|
||||
new_height = int(h / w * new_width)
|
||||
else:
|
||||
new_height = side
|
||||
new_width = int(w / h * new_height)
|
||||
new_size = dict(height=new_height, width=new_width)
|
||||
pixel_values = self.image_processor.preprocess(img, size=new_size, return_tensors='pt')['pixel_values']
|
||||
|
||||
# then pad to square
|
||||
square_values = torch.zeros([1, 3, side, side], dtype=pixel_values.dtype, device=pixel_values.device)
|
||||
new_height, new_width = pixel_values.shape[2:]
|
||||
if new_height == new_width:
|
||||
square_values[:, :, :, :] = pixel_values
|
||||
elif new_height > new_width:
|
||||
from_index = (side - new_width) // 2
|
||||
square_values[:, :, :, from_index:from_index + new_width] = pixel_values
|
||||
else:
|
||||
from_index = (side - new_height) // 2
|
||||
square_values[:, :, from_index:from_index + new_height, :] = pixel_values
|
||||
|
||||
return square_values
|
||||
|
||||
def _partition(img, grid):
|
||||
w, h = img.size
|
||||
row_height = h // grid[0]
|
||||
col_width = w // grid[1]
|
||||
|
||||
partition = []
|
||||
for row in range(grid[0]):
|
||||
for col in range(grid[1]):
|
||||
left = col * col_width
|
||||
upper = row * row_height
|
||||
right = w if col == grid[1] - 1 else (col + 1) * col_width
|
||||
lower = h if row == grid[0] - 1 else (row + 1) * row_height
|
||||
partition.append((left, upper, right, lower))
|
||||
|
||||
return partition
|
||||
|
||||
def _covering_area(left, upper, right, lower, side):
|
||||
w = right - left
|
||||
h = lower - upper
|
||||
w, h = max(w, h), min(w, h)
|
||||
if w > side:
|
||||
h = h / w * side
|
||||
w = side
|
||||
return w * h
|
||||
|
||||
def _get_best_grid(img, side):
|
||||
img_area = img.size[0] * img.size[1]
|
||||
|
||||
candidate_grids = []
|
||||
for i in range(1, max_partition + 1):
|
||||
for j in range(1, max_partition + 1):
|
||||
if i * j <= max_partition:
|
||||
candidate_grids.append((i, j))
|
||||
|
||||
all_grids = []
|
||||
good_grids = []
|
||||
for grid in candidate_grids:
|
||||
partition = _partition(img, grid)
|
||||
covering_ratio = sum([_covering_area(*p, side) for p in partition]) / img_area
|
||||
assert covering_ratio <= 1.0
|
||||
all_grids.append((grid, covering_ratio))
|
||||
if covering_ratio > covering_threshold:
|
||||
good_grids.append((grid, covering_ratio))
|
||||
|
||||
if len(good_grids) > 0:
|
||||
# pick the good partition with minimum #sub_images and break the tie using covering_ratio
|
||||
return sorted(good_grids, key=lambda x: (x[0][0] * x[0][1], -x[1]))[0][0]
|
||||
else:
|
||||
# pick the partition with maximum covering_ratio and break the tie using #sub_images
|
||||
return sorted(all_grids, key=lambda x: (-x[1], x[0][0] * x[0][1]))[0][0]
|
||||
|
||||
if convert_to_rgb and image.mode != 'RGB':
|
||||
image = image.convert('RGB')
|
||||
|
||||
sides = self.get_image_size()
|
||||
if sides[0] != sides[1]:
|
||||
raise ValueError('get_image_size() returns non-square size')
|
||||
side = sides[0]
|
||||
grid = _get_best_grid(image, side)
|
||||
partition = _partition(image, grid)
|
||||
crops = [image.crop(p) for p in partition]
|
||||
if len(crops) > 1:
|
||||
crops.insert(0, image)
|
||||
pixel_values = torch.cat([_preprocess(crop, side) for crop in crops], dim=0)
|
||||
image_placeholders = self.construct_image_placeholders(grid)
|
||||
return pixel_values, image_placeholders
|
||||
|
||||
def tokenize(self, logits):
|
||||
def st_argmax(y_soft, dim): # straight-through softmax
|
||||
index = y_soft.max(dim, keepdim=True)[1]
|
||||
y_hard = torch.zeros_like(y_soft, memory_format=torch.legacy_contiguous_format).scatter_(dim, index, 1.0)
|
||||
ret = y_hard - y_soft.detach() + y_soft
|
||||
return ret
|
||||
|
||||
if self.config.tokenize_function == 'softmax':
|
||||
tokens = softmax(logits, dim=-1)
|
||||
elif self.config.tokenize_function == 'gumbel_argmax':
|
||||
tokens = gumbel_softmax(logits, tau=self.config.tau, hard=True)
|
||||
elif self.config.tokenize_function == 'st_argmax':
|
||||
tokens = st_argmax(logits, dim=-1)
|
||||
else:
|
||||
raise ValueError(
|
||||
f'Invalid `max_type`, expected softmax or gumbel_argmax or st_argmax, but got {self.config.tokenize_function}')
|
||||
return tokens
|
||||
|
||||
def encode(self, pixel_values):
|
||||
output = self.backbone(pixel_values, output_hidden_states=True, return_dict=True)
|
||||
features = output.hidden_states[-1]
|
||||
if self.config.drop_cls_token:
|
||||
features = features[:, 1:, :]
|
||||
|
||||
# merge number of `hidden_stride * hidden_stride` hidden states together to reduce token sequence length
|
||||
# e.g., for hidden_stride=2, this leads to a token length reduction: 1024 -> 256 for aimv2
|
||||
if self.config.hidden_stride > 1:
|
||||
n, l, d = features.shape # this `d` maybe different from the above `d
|
||||
sqrt_l = int(l ** 0.5)
|
||||
assert sqrt_l ** 2 == l, "The token sequence length should be a perfect square."
|
||||
features = features.reshape(n, sqrt_l, sqrt_l, d)
|
||||
pl = (self.config.hidden_stride - (sqrt_l % self.config.hidden_stride)) % self.config.hidden_stride
|
||||
features = pad(features, (0, 0, 0, pl, 0, pl), "constant", 0)
|
||||
sqrt_l += pl
|
||||
features = features.reshape(n, sqrt_l // self.config.hidden_stride, self.config.hidden_stride,
|
||||
sqrt_l // self.config.hidden_stride, self.config.hidden_stride, d)
|
||||
features = features.permute(0, 1, 3, 2, 4, 5) # [n, sqrt_l/hs, sqrt_l/hs, hs, hs, d]
|
||||
features = features.flatten(3) # [n, sqrt_l/hs, sqrt_l/hs, hs*hs*d]
|
||||
features = features.reshape(
|
||||
n, -1, self.config.hidden_stride * self.config.hidden_stride * d)
|
||||
|
||||
return features
|
||||
|
||||
def forward(self, pixel_values) -> torch.Tensor: # [BatchSize, ImageShape] -> [BatchSize, #Token, VocabSize]
|
||||
features = self.encode(pixel_values)
|
||||
logits = self.head(features)
|
||||
tokens = self.tokenize(logits)
|
||||
# tokens' shape is [BatchSize, #Token, VocabSize-5], so padding with [BatchSize, #Token, 5], after
|
||||
# which, tokens' shape should become [BatchSize, #Token, VocabSize]
|
||||
batch_size, token_len, _ = tokens.shape
|
||||
padding_tensor = torch.zeros(size=(batch_size, token_len, len(IMAGE_INDICATOR_IDS)),
|
||||
dtype=tokens.dtype,
|
||||
device=tokens.device,
|
||||
layout=tokens.layout,
|
||||
requires_grad=False)
|
||||
tokens = torch.cat((tokens, padding_tensor), dim=2)
|
||||
return tokens
|
||||
|
||||
|
||||
class Aimv2VisualTokenizer(BaseVisualTokenizer):
|
||||
config_class = Aimv2VisualTokenizerConfig
|
||||
supports_gradient_checkpointing = True
|
||||
_no_split_modules = ["AIMv2ViTPreprocessor", "AIMv2Block"]
|
||||
_image_processor_kwargs = dict(do_center_crop=False)
|
||||
|
||||
def get_image_size(self):
|
||||
height = self.image_processor.crop_size["height"]
|
||||
width = self.image_processor.crop_size["width"]
|
||||
return height, width
|
||||
|
||||
|
||||
AutoModel.register(Aimv2VisualTokenizerConfig, Aimv2VisualTokenizer)
|
||||
|
||||
|
||||
# ----------------------------------------------------------------------
|
||||
# Ovis
|
||||
# ----------------------------------------------------------------------
|
||||
class VisualEmbedding(torch.nn.Embedding):
|
||||
def forward(self, visual_tokens: Tensor) -> Tensor:
|
||||
if visual_tokens.dtype in [torch.int8, torch.int16, torch.int32, torch.int64, torch.long]:
|
||||
return super().forward(visual_tokens)
|
||||
return torch.matmul(visual_tokens, self.weight)
|
||||
|
||||
def reset_parameters(self, mean=0., std=1.) -> None:
|
||||
init.normal_(self.weight, mean=mean, std=std)
|
||||
self._fill_padding_idx_with_zero()
|
||||
|
||||
|
||||
class OvisPreTrainedModel(PreTrainedModel):
|
||||
config_class = OvisConfig
|
||||
base_model_prefix = "ovis"
|
||||
|
||||
|
||||
class Ovis(OvisPreTrainedModel):
|
||||
|
||||
def __init__(self, config: OvisConfig, *inputs, **kwargs):
|
||||
super().__init__(config, *inputs, **kwargs)
|
||||
attn_kwargs = dict()
|
||||
if self.config.llm_attn_implementation:
|
||||
if self.config.llm_attn_implementation == "flash_attention_2":
|
||||
assert (is_flash_attn_2_available() and
|
||||
version.parse(importlib.metadata.version("flash_attn")) >= version.parse("2.6.3")), \
|
||||
"Using `flash_attention_2` requires having `flash_attn>=2.6.3` installed."
|
||||
attn_kwargs["attn_implementation"] = self.config.llm_attn_implementation
|
||||
self.llm = AutoModelForCausalLM.from_config(self.config.llm_config, **attn_kwargs)
|
||||
assert self.config.hidden_size == self.llm.config.hidden_size, "hidden size mismatch"
|
||||
self.text_tokenizer = AutoTokenizer.from_pretrained(self.config.name_or_path)
|
||||
self.visual_tokenizer = AutoModel.from_config(self.config.visual_tokenizer_config,
|
||||
image_processor_name_or_path=self.config.name_or_path)
|
||||
self.vte = VisualEmbedding(
|
||||
self.config.visual_tokenizer_config.vocab_size,
|
||||
self.config.hidden_size,
|
||||
device=self.visual_tokenizer.device,
|
||||
dtype=self.visual_tokenizer.dtype
|
||||
)
|
||||
|
||||
def _merge_modules(modules_list: tuple):
|
||||
merged_modules = []
|
||||
for modules in modules_list:
|
||||
merged_modules.extend(modules if modules else [])
|
||||
return merged_modules
|
||||
|
||||
self._no_split_modules = _merge_modules((self.llm._no_split_modules, self.visual_tokenizer._no_split_modules))
|
||||
self._skip_keys_device_placement = self.llm._skip_keys_device_placement
|
||||
self._keep_in_fp32_modules = _merge_modules(
|
||||
(self.llm._keep_in_fp32_modules, self.visual_tokenizer._keep_in_fp32_modules))
|
||||
self.is_parallelizable = all((self.llm.is_parallelizable, self.visual_tokenizer.is_parallelizable))
|
||||
self.supports_gradient_checkpointing = True
|
||||
self._supports_flash_attn_2 = True
|
||||
|
||||
def get_text_tokenizer(self):
|
||||
return self.text_tokenizer
|
||||
|
||||
def get_visual_tokenizer(self):
|
||||
return self.visual_tokenizer
|
||||
|
||||
def tie_weights(self):
|
||||
if not self.config.disable_tie_weight:
|
||||
self.get_llm().tie_weights()
|
||||
|
||||
def get_llm(self):
|
||||
return self.llm
|
||||
|
||||
def get_vte(self):
|
||||
return self.vte
|
||||
|
||||
def get_wte(self):
|
||||
return self.llm.get_input_embeddings()
|
||||
|
||||
def get_conversation_formatter(self) -> ConversationFormatter:
|
||||
if getattr(self, 'conversation_formatter', None) is None:
|
||||
self.conversation_formatter = getattr(import_module(".configuration_ovis", __package__),
|
||||
self.config.conversation_formatter_class)(self.text_tokenizer)
|
||||
return self.conversation_formatter
|
||||
|
||||
def forward(
|
||||
self,
|
||||
input_ids: torch.Tensor,
|
||||
attention_mask: torch.Tensor,
|
||||
labels: Optional[torch.Tensor],
|
||||
pixel_values: List[Optional[torch.Tensor]],
|
||||
**kwargs
|
||||
):
|
||||
# assert self.training, "`forward` can only be used in training. For inference, use `generate`."
|
||||
_, inputs_embeds, labels, attention_mask = self.merge_multimodal(
|
||||
text_input_ids=input_ids,
|
||||
text_attention_masks=attention_mask,
|
||||
text_labels=labels,
|
||||
pixel_values=pixel_values
|
||||
)
|
||||
return self.llm(inputs_embeds=inputs_embeds, labels=labels, attention_mask=attention_mask, **kwargs)
|
||||
|
||||
def merge_multimodal(
|
||||
self,
|
||||
text_input_ids: torch.Tensor,
|
||||
text_attention_masks: torch.Tensor,
|
||||
text_labels: Optional[torch.Tensor],
|
||||
pixel_values: List[Optional[torch.Tensor]],
|
||||
left_padding: bool = False
|
||||
):
|
||||
input_device = text_input_ids.device
|
||||
visual_vocab_szie = self.get_visual_tokenizer().config.vocab_size
|
||||
visual_indicator_embeds = self.get_vte()(
|
||||
torch.tensor(
|
||||
list(range(visual_vocab_szie - 5, visual_vocab_szie)),
|
||||
dtype=torch.long,
|
||||
device=self.get_visual_tokenizer().device
|
||||
)
|
||||
).to(device=input_device)
|
||||
|
||||
if self.training:
|
||||
# When training, to be compatible with deepspeed zero, each sample has to include pixel_value tensor.
|
||||
# For text-only sample, one can simply use a full zero tensor as pixel_value, which will be ignored
|
||||
# (see below in this function); so, the gradient will not be affected.
|
||||
num_images = [x.shape[0] for x in pixel_values]
|
||||
visual_tokens = self.visual_tokenizer(torch.cat([x for x in pixel_values], dim=0))
|
||||
visual_embeds = torch.split(self.get_vte()(visual_tokens).to(dtype=self.dtype, device=input_device),
|
||||
split_size_or_sections=num_images, dim=0)
|
||||
visual_input_ids = torch.split(torch.argmax(visual_tokens, dim=-1).to(device=input_device),
|
||||
split_size_or_sections=num_images, dim=0)
|
||||
visual_labels = [torch.full(x.shape, IGNORE_ID, dtype=torch.long, device=input_device) for x in
|
||||
visual_input_ids]
|
||||
else:
|
||||
# When inference, sample can include only text with `None` pixel_value
|
||||
num_images = [x.shape[0] if x is not None else 0 for x in pixel_values]
|
||||
if sum(num_images) > 0:
|
||||
visual_tokens = self.visual_tokenizer(torch.cat([x for x in pixel_values if x is not None], dim=0))
|
||||
visual_embeds = torch.split(self.get_vte()(visual_tokens).to(dtype=self.dtype, device=input_device),
|
||||
split_size_or_sections=num_images, dim=0)
|
||||
visual_input_ids = torch.split(torch.argmax(visual_tokens, dim=-1).to(device=input_device),
|
||||
split_size_or_sections=num_images, dim=0)
|
||||
visual_labels = [torch.full(x.shape, IGNORE_ID, dtype=torch.long, device=input_device) for x in
|
||||
visual_input_ids]
|
||||
else:
|
||||
# just placeholders
|
||||
visual_embeds = [None] * len(num_images)
|
||||
visual_input_ids = [None] * len(num_images)
|
||||
visual_labels = [None] * len(num_images)
|
||||
# just placeholders
|
||||
if text_labels is None:
|
||||
text_labels = torch.full(text_input_ids.shape, IGNORE_ID, dtype=torch.long, device=input_device)
|
||||
|
||||
input_embeds = []
|
||||
attention_masks = []
|
||||
labels = []
|
||||
for text_input_id, text_label, text_attention_mask, visual_embed, visual_input_id, visual_label in zip(
|
||||
text_input_ids, text_labels, text_attention_masks, visual_embeds, visual_input_ids, visual_labels
|
||||
):
|
||||
placeholder_token_mask = torch.lt(text_input_id, 0)
|
||||
text_embed = self.get_wte()(torch.masked_fill(text_input_id, placeholder_token_mask, 0))
|
||||
for i, indicator_id in enumerate(IMAGE_INDICATOR_IDS):
|
||||
text_embed[text_input_id == indicator_id] = visual_indicator_embeds[i]
|
||||
image_atom_positions = torch.where(torch.eq(text_input_id, IMAGE_ATOM_ID))[0].tolist()
|
||||
if len(image_atom_positions) > 0:
|
||||
input_embed_parts = []
|
||||
attention_mask_parts = []
|
||||
label_parts = []
|
||||
prev_image_atom_position = -1
|
||||
for index, image_atom_position in enumerate(image_atom_positions):
|
||||
input_embed_parts.append(
|
||||
text_embed[prev_image_atom_position + 1:image_atom_position, :])
|
||||
label_parts.append(
|
||||
text_label[prev_image_atom_position + 1:image_atom_position])
|
||||
attention_mask_parts.append(
|
||||
text_attention_mask[prev_image_atom_position + 1:image_atom_position])
|
||||
input_embed_parts.append(visual_embed[index])
|
||||
attention_mask_parts.append(
|
||||
torch.ones_like(visual_label[index], dtype=torch.bool))
|
||||
label_parts.append(visual_label[index])
|
||||
prev_image_atom_position = image_atom_position
|
||||
if prev_image_atom_position + 1 < text_input_id.shape[0]:
|
||||
input_embed_parts.append(
|
||||
text_embed[prev_image_atom_position + 1:, :])
|
||||
attention_mask_parts.append(
|
||||
text_attention_mask[prev_image_atom_position + 1:])
|
||||
label_parts.append(
|
||||
text_label[prev_image_atom_position + 1:])
|
||||
input_embed = torch.cat(input_embed_parts, dim=0)
|
||||
attention_mask = torch.cat(attention_mask_parts, dim=0)
|
||||
label = torch.cat(label_parts, dim=0)
|
||||
else:
|
||||
input_embed = text_embed
|
||||
attention_mask = text_attention_mask
|
||||
label = text_label
|
||||
if self.training:
|
||||
# Make visual_embed & visual_indicator_embeds involved in the backward graph,
|
||||
# to be compatible with deepspeed zero and ddp.
|
||||
input_embed += torch.sum(visual_embed * 0.0) + torch.sum(visual_indicator_embeds * 0.0)
|
||||
input_embeds.append(input_embed)
|
||||
attention_masks.append(attention_mask)
|
||||
labels.append(label)
|
||||
|
||||
if self.training: # padding to self.config.multimodal_max_length for increased training speed
|
||||
padding_size = max(0, self.config.multimodal_max_length - len(input_embeds[0]))
|
||||
input_embeds[0] = torch.nn.ConstantPad2d((0, 0, 0, padding_size), 0.0)(input_embeds[0])
|
||||
attention_masks[0] = torch.nn.ConstantPad1d((0, padding_size), False)(attention_masks[0])
|
||||
labels[0] = torch.nn.ConstantPad1d((0, padding_size), IGNORE_ID)(labels[0])
|
||||
batch_input_embeds = self.pad_truncate_sequence(input_embeds, batch_first=True, padding_value=0.0, left_padding=left_padding)
|
||||
batch_attention_mask = self.pad_truncate_sequence(attention_masks, batch_first=True, padding_value=False, left_padding=left_padding)
|
||||
batch_labels = self.pad_truncate_sequence(labels, batch_first=True, padding_value=IGNORE_ID, left_padding=left_padding)
|
||||
|
||||
return visual_input_ids, batch_input_embeds, batch_labels, batch_attention_mask
|
||||
|
||||
def pad_truncate_sequence(self, sequences: List[torch.Tensor], batch_first: bool = True, padding_value: float = 0.0, left_padding: bool = False) -> torch.Tensor:
|
||||
if not left_padding:
|
||||
pad_sequence = torch.nn.utils.rnn.pad_sequence(sequences, batch_first=batch_first, padding_value=padding_value)
|
||||
return pad_sequence[:,:self.config.multimodal_max_length]
|
||||
else:
|
||||
pad_sequence = torch.nn.utils.rnn.pad_sequence([i.flip(dims=[0]) for i in sequences],batch_first=True, padding_value=padding_value).flip(dims=[1])
|
||||
return pad_sequence[:,-self.config.multimodal_max_length:]
|
||||
|
||||
def preprocess_inputs(
|
||||
self,
|
||||
text_or_conversations: Union[List[Dict], str],
|
||||
images: Optional[List[PIL.Image.Image]],
|
||||
max_partition=9,
|
||||
generation_preface='',
|
||||
return_labels=False,
|
||||
propagate_exception=True,
|
||||
frame_selector=None,
|
||||
frame_selector_kwargs=None
|
||||
):
|
||||
# convert text to conversations
|
||||
if isinstance(text_or_conversations, str):
|
||||
conversations = [{
|
||||
"from": "human",
|
||||
"value": text_or_conversations
|
||||
}]
|
||||
elif isinstance(text_or_conversations, list):
|
||||
conversations = text_or_conversations
|
||||
else:
|
||||
raise ValueError(f'Invalid type of `text_or_conversations`, expected `List[Dict]` or `str`,'
|
||||
f' but got {type(text_or_conversations)}')
|
||||
|
||||
if frame_selector is not None:
|
||||
frame_selector_kwargs = frame_selector_kwargs or {}
|
||||
conversations, images = frame_selector(conversations=conversations, frames=images, **frame_selector_kwargs)
|
||||
|
||||
# format conversations
|
||||
prompt, raw_input_ids, raw_labels = self.get_conversation_formatter().format(
|
||||
conversations, generation_preface=generation_preface)
|
||||
|
||||
# place image placeholders
|
||||
input_ids = []
|
||||
labels = []
|
||||
pixel_values = []
|
||||
invalidate_label = False
|
||||
image_token_indices = [i for i, v in enumerate(raw_input_ids) if v == IMAGE_TOKEN_ID]
|
||||
last_image_token_index = -1
|
||||
for i in range(len(image_token_indices)):
|
||||
head = 0 if i == 0 else image_token_indices[i - 1] + 1
|
||||
tail = image_token_indices[i]
|
||||
last_image_token_index = tail
|
||||
input_ids.extend(raw_input_ids[head:tail])
|
||||
labels.extend(raw_labels[head:tail])
|
||||
try:
|
||||
image = images[i]
|
||||
raw_pixel_values, image_placeholders = self.visual_tokenizer.preprocess_image(
|
||||
image, max_partition=max_partition)
|
||||
except Exception as e:
|
||||
if propagate_exception:
|
||||
raise e
|
||||
logging.exception(e)
|
||||
invalidate_label = True
|
||||
raw_pixel_values, image_placeholders = self.visual_tokenizer.mock_input()
|
||||
input_ids.extend(image_placeholders)
|
||||
labels.extend([IGNORE_ID] * len(image_placeholders))
|
||||
pixel_values.append(raw_pixel_values)
|
||||
input_ids.extend(raw_input_ids[last_image_token_index + 1:])
|
||||
labels.extend(raw_labels[last_image_token_index + 1:])
|
||||
|
||||
# return tensors
|
||||
input_ids = torch.tensor(input_ids, dtype=torch.long)
|
||||
labels = torch.tensor([IGNORE_ID] * len(labels) if invalidate_label else labels, dtype=torch.long)
|
||||
pixel_values = torch.cat(pixel_values, dim=0) if len(pixel_values) > 0 else None
|
||||
|
||||
if return_labels:
|
||||
return prompt, input_ids, pixel_values, labels
|
||||
else:
|
||||
return prompt, input_ids, pixel_values
|
||||
|
||||
def save_pretrained(
|
||||
self,
|
||||
save_directory: Union[str, os.PathLike],
|
||||
is_main_process: bool = True,
|
||||
state_dict: Optional[dict] = None,
|
||||
save_function: Callable = torch.save,
|
||||
push_to_hub: bool = False,
|
||||
max_shard_size: Union[int, str] = "5GB",
|
||||
safe_serialization: bool = True,
|
||||
variant: Optional[str] = None,
|
||||
token: Optional[Union[str, bool]] = None,
|
||||
save_peft_format: bool = True,
|
||||
**kwargs
|
||||
):
|
||||
super().save_pretrained(save_directory,
|
||||
is_main_process=is_main_process,
|
||||
state_dict=state_dict,
|
||||
save_function=save_function,
|
||||
safe_serialization=safe_serialization)
|
||||
self.get_text_tokenizer().save_pretrained(save_directory)
|
||||
self.get_visual_tokenizer().get_image_processor().save_pretrained(save_directory)
|
||||
|
||||
def generate(
|
||||
self,
|
||||
inputs: Optional[torch.Tensor] = None,
|
||||
**kwargs
|
||||
) -> Union[GenerateOutput, torch.LongTensor]:
|
||||
_, inputs_embeds, labels, attention_mask = self.merge_multimodal(
|
||||
text_input_ids=inputs,
|
||||
text_attention_masks=kwargs.pop('attention_mask'),
|
||||
text_labels=None,
|
||||
pixel_values=kwargs.pop('pixel_values'),
|
||||
left_padding=True
|
||||
)
|
||||
inputs_embeds = inputs_embeds.detach()
|
||||
torch.cuda.empty_cache()
|
||||
|
||||
return self.llm.generate(inputs=None, inputs_embeds=inputs_embeds, attention_mask=attention_mask, **kwargs)
|
|
@ -0,0 +1,27 @@
|
|||
{
|
||||
"crop_size": {
|
||||
"height": 448,
|
||||
"width": 448
|
||||
},
|
||||
"do_center_crop": false,
|
||||
"do_convert_rgb": true,
|
||||
"do_normalize": true,
|
||||
"do_rescale": true,
|
||||
"do_resize": true,
|
||||
"image_mean": [
|
||||
0.48145466,
|
||||
0.4578275,
|
||||
0.40821073
|
||||
],
|
||||
"image_processor_type": "CLIPImageProcessor",
|
||||
"image_std": [
|
||||
0.26862954,
|
||||
0.26130258,
|
||||
0.27577711
|
||||
],
|
||||
"resample": 3,
|
||||
"rescale_factor": 0.00392156862745098,
|
||||
"size": {
|
||||
"shortest_edge": 448
|
||||
}
|
||||
}
|
|
@ -0,0 +1,31 @@
|
|||
{
|
||||
"additional_special_tokens": [
|
||||
"<|im_start|>",
|
||||
"<|im_end|>",
|
||||
"<|object_ref_start|>",
|
||||
"<|object_ref_end|>",
|
||||
"<|box_start|>",
|
||||
"<|box_end|>",
|
||||
"<|quad_start|>",
|
||||
"<|quad_end|>",
|
||||
"<|vision_start|>",
|
||||
"<|vision_end|>",
|
||||
"<|vision_pad|>",
|
||||
"<|image_pad|>",
|
||||
"<|video_pad|>"
|
||||
],
|
||||
"eos_token": {
|
||||
"content": "<|im_end|>",
|
||||
"lstrip": false,
|
||||
"normalized": false,
|
||||
"rstrip": false,
|
||||
"single_word": false
|
||||
},
|
||||
"pad_token": {
|
||||
"content": "<|endoftext|>",
|
||||
"lstrip": false,
|
||||
"normalized": false,
|
||||
"rstrip": false,
|
||||
"single_word": false
|
||||
}
|
||||
}
|
Binary file not shown.
|
@ -0,0 +1,207 @@
|
|||
{
|
||||
"add_bos_token": false,
|
||||
"add_prefix_space": false,
|
||||
"added_tokens_decoder": {
|
||||
"151643": {
|
||||
"content": "<|endoftext|>",
|
||||
"lstrip": false,
|
||||
"normalized": false,
|
||||
"rstrip": false,
|
||||
"single_word": false,
|
||||
"special": true
|
||||
},
|
||||
"151644": {
|
||||
"content": "<|im_start|>",
|
||||
"lstrip": false,
|
||||
"normalized": false,
|
||||
"rstrip": false,
|
||||
"single_word": false,
|
||||
"special": true
|
||||
},
|
||||
"151645": {
|
||||
"content": "<|im_end|>",
|
||||
"lstrip": false,
|
||||
"normalized": false,
|
||||
"rstrip": false,
|
||||
"single_word": false,
|
||||
"special": true
|
||||
},
|
||||
"151646": {
|
||||
"content": "<|object_ref_start|>",
|
||||
"lstrip": false,
|
||||
"normalized": false,
|
||||
"rstrip": false,
|
||||
"single_word": false,
|
||||
"special": true
|
||||
},
|
||||
"151647": {
|
||||
"content": "<|object_ref_end|>",
|
||||
"lstrip": false,
|
||||
"normalized": false,
|
||||
"rstrip": false,
|
||||
"single_word": false,
|
||||
"special": true
|
||||
},
|
||||
"151648": {
|
||||
"content": "<|box_start|>",
|
||||
"lstrip": false,
|
||||
"normalized": false,
|
||||
"rstrip": false,
|
||||
"single_word": false,
|
||||
"special": true
|
||||
},
|
||||
"151649": {
|
||||
"content": "<|box_end|>",
|
||||
"lstrip": false,
|
||||
"normalized": false,
|
||||
"rstrip": false,
|
||||
"single_word": false,
|
||||
"special": true
|
||||
},
|
||||
"151650": {
|
||||
"content": "<|quad_start|>",
|
||||
"lstrip": false,
|
||||
"normalized": false,
|
||||
"rstrip": false,
|
||||
"single_word": false,
|
||||
"special": true
|
||||
},
|
||||
"151651": {
|
||||
"content": "<|quad_end|>",
|
||||
"lstrip": false,
|
||||
"normalized": false,
|
||||
"rstrip": false,
|
||||
"single_word": false,
|
||||
"special": true
|
||||
},
|
||||
"151652": {
|
||||
"content": "<|vision_start|>",
|
||||
"lstrip": false,
|
||||
"normalized": false,
|
||||
"rstrip": false,
|
||||
"single_word": false,
|
||||
"special": true
|
||||
},
|
||||
"151653": {
|
||||
"content": "<|vision_end|>",
|
||||
"lstrip": false,
|
||||
"normalized": false,
|
||||
"rstrip": false,
|
||||
"single_word": false,
|
||||
"special": true
|
||||
},
|
||||
"151654": {
|
||||
"content": "<|vision_pad|>",
|
||||
"lstrip": false,
|
||||
"normalized": false,
|
||||
"rstrip": false,
|
||||
"single_word": false,
|
||||
"special": true
|
||||
},
|
||||
"151655": {
|
||||
"content": "<|image_pad|>",
|
||||
"lstrip": false,
|
||||
"normalized": false,
|
||||
"rstrip": false,
|
||||
"single_word": false,
|
||||
"special": true
|
||||
},
|
||||
"151656": {
|
||||
"content": "<|video_pad|>",
|
||||
"lstrip": false,
|
||||
"normalized": false,
|
||||
"rstrip": false,
|
||||
"single_word": false,
|
||||
"special": true
|
||||
},
|
||||
"151657": {
|
||||
"content": "<tool_call>",
|
||||
"lstrip": false,
|
||||
"normalized": false,
|
||||
"rstrip": false,
|
||||
"single_word": false,
|
||||
"special": false
|
||||
},
|
||||
"151658": {
|
||||
"content": "</tool_call>",
|
||||
"lstrip": false,
|
||||
"normalized": false,
|
||||
"rstrip": false,
|
||||
"single_word": false,
|
||||
"special": false
|
||||
},
|
||||
"151659": {
|
||||
"content": "<|fim_prefix|>",
|
||||
"lstrip": false,
|
||||
"normalized": false,
|
||||
"rstrip": false,
|
||||
"single_word": false,
|
||||
"special": false
|
||||
},
|
||||
"151660": {
|
||||
"content": "<|fim_middle|>",
|
||||
"lstrip": false,
|
||||
"normalized": false,
|
||||
"rstrip": false,
|
||||
"single_word": false,
|
||||
"special": false
|
||||
},
|
||||
"151661": {
|
||||
"content": "<|fim_suffix|>",
|
||||
"lstrip": false,
|
||||
"normalized": false,
|
||||
"rstrip": false,
|
||||
"single_word": false,
|
||||
"special": false
|
||||
},
|
||||
"151662": {
|
||||
"content": "<|fim_pad|>",
|
||||
"lstrip": false,
|
||||
"normalized": false,
|
||||
"rstrip": false,
|
||||
"single_word": false,
|
||||
"special": false
|
||||
},
|
||||
"151663": {
|
||||
"content": "<|repo_name|>",
|
||||
"lstrip": false,
|
||||
"normalized": false,
|
||||
"rstrip": false,
|
||||
"single_word": false,
|
||||
"special": false
|
||||
},
|
||||
"151664": {
|
||||
"content": "<|file_sep|>",
|
||||
"lstrip": false,
|
||||
"normalized": false,
|
||||
"rstrip": false,
|
||||
"single_word": false,
|
||||
"special": false
|
||||
}
|
||||
},
|
||||
"additional_special_tokens": [
|
||||
"<|im_start|>",
|
||||
"<|im_end|>",
|
||||
"<|object_ref_start|>",
|
||||
"<|object_ref_end|>",
|
||||
"<|box_start|>",
|
||||
"<|box_end|>",
|
||||
"<|quad_start|>",
|
||||
"<|quad_end|>",
|
||||
"<|vision_start|>",
|
||||
"<|vision_end|>",
|
||||
"<|vision_pad|>",
|
||||
"<|image_pad|>",
|
||||
"<|video_pad|>"
|
||||
],
|
||||
"bos_token": null,
|
||||
"chat_template": "{%- if tools %}\n {{- '<|im_start|>system\\n' }}\n {%- if messages[0]['role'] == 'system' %}\n {{- messages[0]['content'] }}\n {%- else %}\n {{- 'You are Qwen, created by Alibaba Cloud. You are a helpful assistant.' }}\n {%- endif %}\n {{- \"\\n\\n# Tools\\n\\nYou may call one or more functions to assist with the user query.\\n\\nYou are provided with function signatures within <tools></tools> XML tags:\\n<tools>\" }}\n {%- for tool in tools %}\n {{- \"\\n\" }}\n {{- tool | tojson }}\n {%- endfor %}\n {{- \"\\n</tools>\\n\\nFor each function call, return a json object with function name and arguments within <tool_call></tool_call> XML tags:\\n<tool_call>\\n{\\\"name\\\": <function-name>, \\\"arguments\\\": <args-json-object>}\\n</tool_call><|im_end|>\\n\" }}\n{%- else %}\n {%- if messages[0]['role'] == 'system' %}\n {{- '<|im_start|>system\\n' + messages[0]['content'] + '<|im_end|>\\n' }}\n {%- else %}\n {{- '<|im_start|>system\\nYou are Qwen, created by Alibaba Cloud. You are a helpful assistant.<|im_end|>\\n' }}\n {%- endif %}\n{%- endif %}\n{%- for message in messages %}\n {%- if (message.role == \"user\") or (message.role == \"system\" and not loop.first) or (message.role == \"assistant\" and not message.tool_calls) %}\n {{- '<|im_start|>' + message.role + '\\n' + message.content + '<|im_end|>' + '\\n' }}\n {%- elif message.role == \"assistant\" %}\n {{- '<|im_start|>' + message.role }}\n {%- if message.content %}\n {{- '\\n' + message.content }}\n {%- endif %}\n {%- for tool_call in message.tool_calls %}\n {%- if tool_call.function is defined %}\n {%- set tool_call = tool_call.function %}\n {%- endif %}\n {{- '\\n<tool_call>\\n{\"name\": \"' }}\n {{- tool_call.name }}\n {{- '\", \"arguments\": ' }}\n {{- tool_call.arguments | tojson }}\n {{- '}\\n</tool_call>' }}\n {%- endfor %}\n {{- '<|im_end|>\\n' }}\n {%- elif message.role == \"tool\" %}\n {%- if (loop.index0 == 0) or (messages[loop.index0 - 1].role != \"tool\") %}\n {{- '<|im_start|>user' }}\n {%- endif %}\n {{- '\\n<tool_response>\\n' }}\n {{- message.content }}\n {{- '\\n</tool_response>' }}\n {%- if loop.last or (messages[loop.index0 + 1].role != \"tool\") %}\n {{- '<|im_end|>\\n' }}\n {%- endif %}\n {%- endif %}\n{%- endfor %}\n{%- if add_generation_prompt %}\n {{- '<|im_start|>assistant\\n' }}\n{%- endif %}\n",
|
||||
"clean_up_tokenization_spaces": false,
|
||||
"eos_token": "<|im_end|>",
|
||||
"errors": "replace",
|
||||
"model_max_length": 131072,
|
||||
"pad_token": "<|endoftext|>",
|
||||
"split_special_tokens": false,
|
||||
"tokenizer_class": "Qwen2Tokenizer",
|
||||
"unk_token": null
|
||||
}
|
File diff suppressed because one or more lines are too long
Loading…
Reference in New Issue