Compare commits
No commits in common. "6b8f016ccc1b931043b6c028d818f671ced170ce" and "e3e251a65d52d28b018a0213f3b5816f11851629" have entirely different histories.
6b8f016ccc
...
e3e251a65d
84
LICENSE
84
LICENSE
|
@ -1,84 +0,0 @@
|
|||
The glm-4-9b License
|
||||
|
||||
1. 定义
|
||||
|
||||
“许可方”是指分发其软件的 glm-4-9b 模型团队。
|
||||
“软件”是指根据本许可提供的 glm-4-9b 模型参数。
|
||||
|
||||
2. 许可授予
|
||||
|
||||
根据本许可的条款和条件,许可方特此授予您非排他性、全球性、不可转让、不可再许可、可撤销、免版税的版权许可。
|
||||
本许可允许您免费使用本仓库中的所有开源模型进行学术研究,对于希望将模型用于商业目的的用户,需在[这里](https://open.bigmodel.cn/mla/form)完成登记。经过登记的用户可以免费使用本模型进行商业活动,但必须遵守本许可的所有条款和条件。
|
||||
上述版权声明和本许可声明应包含在本软件的所有副本或重要部分中。
|
||||
如果您分发或提供 THUDM / 智谱AI 关于 glm-4 开源模型的材料(或其任何衍生作品),或使用其中任何材料(包括 glm-4 系列的所有开源模型)的产品或服务,您应:
|
||||
|
||||
(A) 随任何此类 THUDM / 智谱AI 材料提供本协议的副本;
|
||||
(B) 在相关网站、用户界面、博客文章、关于页面或产品文档上突出显示 “Built with glm-4”。
|
||||
如果您使用 THUDM / 智谱AI的 glm-4 开源模型的材料来创建、训练、微调或以其他方式改进已分发或可用的 AI 模型,您还应在任何此类 AI 模型名称的开头添加 “glm-4”。
|
||||
|
||||
3. 限制
|
||||
|
||||
您不得出于任何军事或非法目的使用、复制、修改、合并、发布、分发、复制或创建本软件的全部或部分衍生作品。
|
||||
您不得利用本软件从事任何危害国家安全和国家统一,危害社会公共利益及公序良俗,侵犯他人商业秘密、知识产权、名誉权、肖像权、财产权等权益的行为。
|
||||
您在使用中应遵循使用地所适用的法律法规政策、道德规范等要求。
|
||||
|
||||
4. 免责声明
|
||||
|
||||
本软件“按原样”提供,不提供任何明示或暗示的保证,包括但不限于对适销性、特定用途的适用性和非侵权性的保证。
|
||||
在任何情况下,作者或版权持有人均不对任何索赔、损害或其他责任负责,无论是在合同诉讼、侵权行为还是其他方面,由软件或软件的使用或其他交易引起、由软件引起或与之相关
|
||||
软件。
|
||||
|
||||
5. 责任限制
|
||||
|
||||
除适用法律禁止的范围外,在任何情况下且根据任何法律理论,无论是基于侵权行为、疏忽、合同、责任或其他原因,任何许可方均不对您承担任何直接、间接、特殊、偶然、示范性、
|
||||
或间接损害,或任何其他商业损失,即使许可人已被告知此类损害的可能性。
|
||||
|
||||
6. 争议解决
|
||||
|
||||
本许可受中华人民共和国法律管辖并按其解释。 因本许可引起的或与本许可有关的任何争议应提交北京市海淀区人民法院。
|
||||
请注意,许可证可能会更新到更全面的版本。 有关许可和版权的任何问题,请通过 license@zhipuai.cn 与我们联系。
|
||||
|
||||
1. Definitions
|
||||
|
||||
“Licensor” means the glm-4-9b Model Team that distributes its Software.
|
||||
“Software” means the glm-4-9b model parameters made available under this license.
|
||||
|
||||
2. License
|
||||
|
||||
Under the terms and conditions of this license, the Licensor hereby grants you a non-exclusive, worldwide, non-transferable, non-sublicensable, revocable, royalty-free copyright license.
|
||||
This license allows you to use all open source models in this repository for free for academic research. For users who wish to use the models for commercial purposes, please do so [here](https://open.bigmodel.cn/mla/form)
|
||||
Complete registration. Registered users are free to use this model for commercial activities, but must comply with all terms and conditions of this license.
|
||||
The copyright notice and this license notice shall be included in all copies or substantial portions of the Software.
|
||||
If you distribute or provide THUDM / Zhipu AI materials on the glm-4 open source model (or any derivative works thereof), or products or services that use any materials therein (including all open source models of the glm-4 series), you should:
|
||||
|
||||
(A) Provide a copy of this Agreement with any such THUDM/Zhipu AI Materials;
|
||||
(B) Prominently display "Built with glm-4" on the relevant website, user interface, blog post, related page or product documentation.
|
||||
If you use materials from THUDM/Zhipu AI's glm-4 model to create, train, operate, or otherwise improve assigned or available AI models, you should also add "glm-4" to the beginning of any such AI model name.
|
||||
|
||||
3. Restrictions
|
||||
|
||||
You are not allowed to use, copy, modify, merge, publish, distribute, copy or create all or part of the derivative works of this software for any military or illegal purposes.
|
||||
You are not allowed to use this software to engage in any behavior that endangers national security and unity, endangers social public interests and public order, infringes on the rights and interests of others such as trade secrets, intellectual property rights, reputation rights, portrait rights, and property rights.
|
||||
You should comply with the applicable laws, regulations, policies, ethical standards, and other requirements in the place of use during use.
|
||||
|
||||
4. Disclaimer
|
||||
|
||||
THE SOFTWARE IS PROVIDED "AS IS", WITHOUT WARRANTY OF ANY KIND, EXPRESS OR IMPLIED, INCLUDING BUT NOT LIMITED TO THE
|
||||
WARRANTIES OF MERCHANTABILITY, FITNESS FOR A PARTICULAR PURPOSE AND NONINFRINGEMENT. IN NO EVENT SHALL THE AUTHORS OR
|
||||
COPYRIGHT HOLDERS BE LIABLE FOR ANY CLAIM, DAMAGES OR OTHER LIABILITY, WHETHER IN AN ACTION OF CONTRACT, TORT OR
|
||||
OTHERWISE, ARISING FROM, OUT OF OR IN CONNECTION WITH THE SOFTWARE OR THE USE OR OTHER DEALINGS IN THE SOFTWARE.
|
||||
|
||||
5. Limitation of Liability
|
||||
|
||||
EXCEPT TO THE EXTENT PROHIBITED BY APPLICABLE LAW, IN NO EVENT AND UNDER NO LEGAL THEORY, WHETHER BASED IN TORT,
|
||||
NEGLIGENCE, CONTRACT, LIABILITY, OR OTHERWISE WILL ANY LICENSOR BE LIABLE TO YOU FOR ANY DIRECT, INDIRECT, SPECIAL,
|
||||
INCIDENTAL, EXEMPLARY, OR CONSEQUENTIAL DAMAGES, OR ANY OTHER COMMERCIAL LOSSES, EVEN IF THE LICENSOR HAS BEEN ADVISED
|
||||
OF THE POSSIBILITY OF SUCH DAMAGES.
|
||||
|
||||
6. Dispute Resolution
|
||||
|
||||
This license shall be governed and construed in accordance with the laws of People’s Republic of China. Any dispute
|
||||
arising from or in connection with this License shall be submitted to Haidian District People's Court in Beijing.
|
||||
|
||||
Note that the license is subject to update to a more comprehensive version. For any questions related to the license and
|
||||
copyright, please contact us at license@zhipuai.cn.
|
171
README.md
171
README.md
|
@ -1,170 +1,3 @@
|
|||
---
|
||||
license: other
|
||||
license_name: glm-4
|
||||
license_link: https://modelscope.cn/models/ZhipuAI/glm-4-9b-chat/file/view/master?fileName=LICENSE&status=0
|
||||
language:
|
||||
- zh
|
||||
- en
|
||||
tags:
|
||||
- glm
|
||||
- chatglm
|
||||
- thudm
|
||||
inference: false
|
||||
---
|
||||
# glm4_9b_chat
|
||||
|
||||
# GLM-4-9B-Chat
|
||||
|
||||
Read this in [English](https://modelscope.cn/models/ZhipuAI/glm-4-9b-chat/file/view/master?fileName=README_en.md&status=1).
|
||||
|
||||
请注意,GLM-4-9B 系列对话模型 (不含视觉模型) 实现已经被 Hugging Face transformers 合并并标准化,自 `4.46.0` 版本起,
|
||||
本仓库模型可能无法正常使用。 请移步适配最新 transformers 的 [模型仓库](https://modelscope.cn/models/ZhipuAI/glm-4-9b-chat-hf) 使用。
|
||||
|
||||
## 模型介绍
|
||||
|
||||
GLM-4-9B 是智谱 AI 推出的最新一代预训练模型 GLM-4 系列中的开源版本。
|
||||
在语义、数学、推理、代码和知识等多方面的数据集测评中,GLM-4-9B 及其人类偏好对齐的版本 GLM-4-9B-Chat 均表现出较高的性能。
|
||||
除了能进行多轮对话,GLM-4-9B-Chat 还具备网页浏览、代码执行、自定义工具调用(Function Call)和长文本推理(支持最大 128K
|
||||
上下文)等高级功能。
|
||||
本代模型增加了多语言支持,支持包括日语,韩语,德语在内的 26 种语言。我们还推出了支持 1M 上下文长度(约 200 万中文字符)的模型。
|
||||
|
||||
## 评测结果
|
||||
|
||||
我们在一些经典任务上对 GLM-4-9B-Chat 模型进行了评测,并得到了如下的结果:
|
||||
|
||||
| Model | AlignBench-v2 | MT-Bench | IFEval | MMLU | C-Eval | GSM8K | MATH | HumanEval | NCB |
|
||||
|:--------------------|:-------------:|:--------:|:------:|:----:|:------:|:-----:|:----:|:---------:|:----:|
|
||||
| Llama-3-8B-Instruct | 5.12 | 8.00 | 68.58 | 68.4 | 51.3 | 79.6 | 30.0 | 62.2 | 24.7 |
|
||||
| ChatGLM3-6B | 3.97 | 5.50 | 28.1 | 66.4 | 69.0 | 72.3 | 25.7 | 58.5 | 11.3 |
|
||||
| GLM-4-9B-Chat | 6.61 | 8.35 | 69.0 | 72.4 | 75.6 | 79.6 | 50.6 | 71.8 | 32.2 |
|
||||
|
||||
### 长文本
|
||||
|
||||
在 1M 的上下文长度下进行[大海捞针实验](https://github.com/LargeWorldModel/LWM/blob/main/scripts/eval_needle.py),结果如下:
|
||||
|
||||
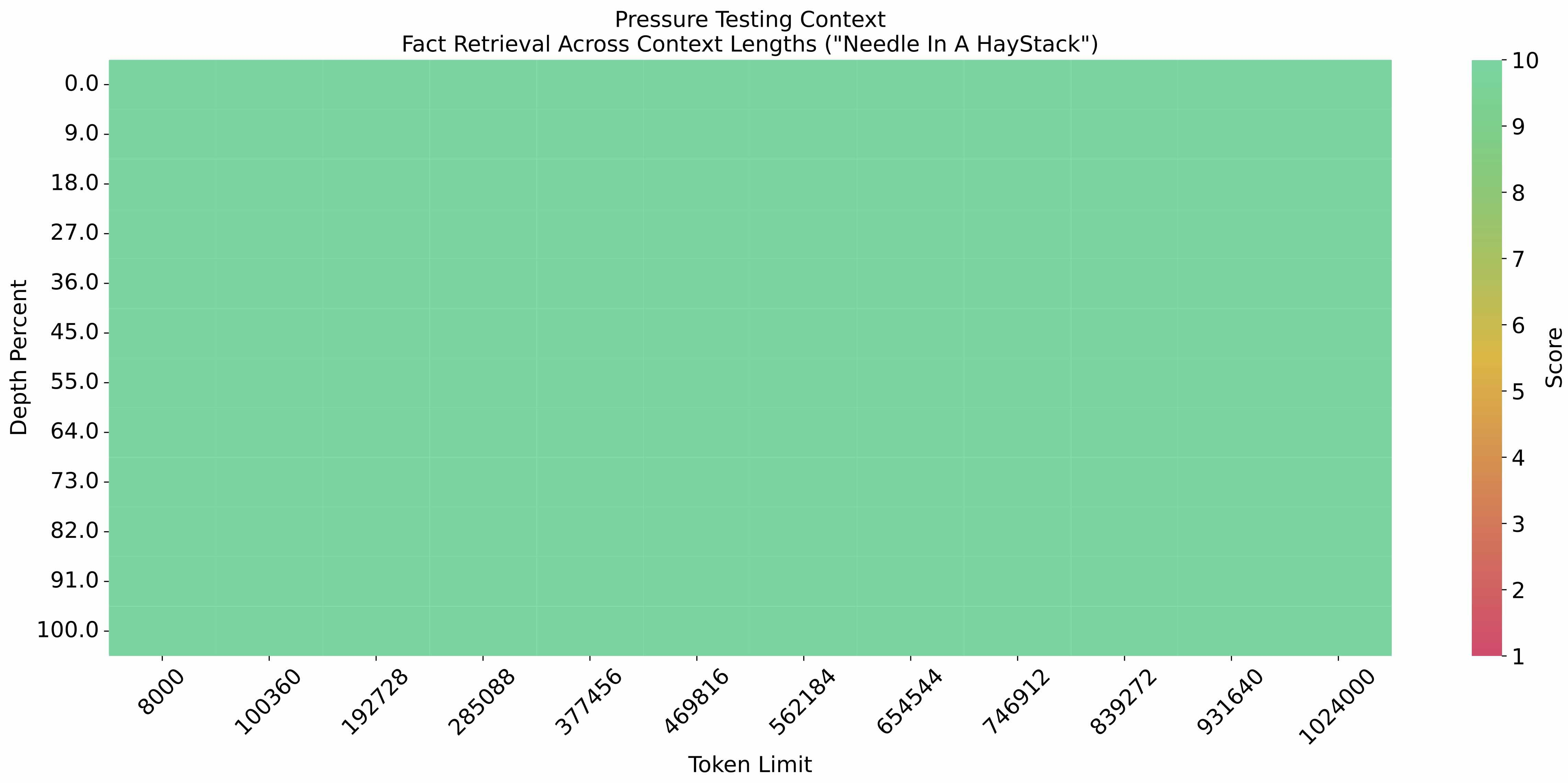
|
||||
|
||||
在 LongBench-Chat 上对长文本能力进行了进一步评测,结果如下:
|
||||
|
||||
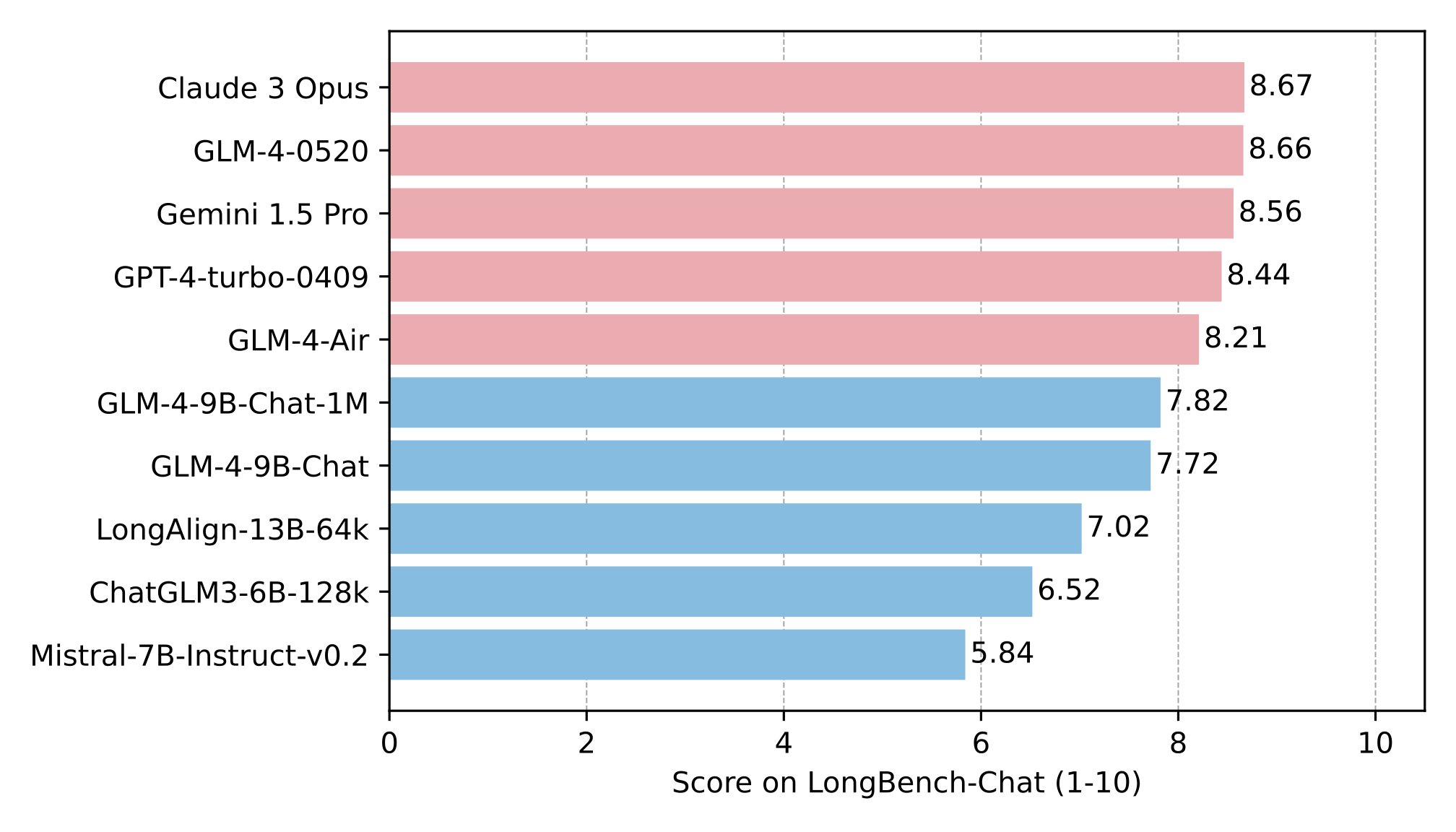
|
||||
|
||||
### 多语言能力
|
||||
|
||||
在六个多语言数据集上对 GLM-4-9B-Chat 和 Llama-3-8B-Instruct 进行了测试,测试结果及数据集对应选取语言如下表
|
||||
|
||||
| Dataset | Llama-3-8B-Instruct | GLM-4-9B-Chat | Languages
|
||||
|:------------|:-------------------:|:-------------:|:----------------------------------------------------------------------------------------------:|
|
||||
| M-MMLU | 49.6 | 56.6 | all
|
||||
| FLORES | 25.0 | 28.8 | ru, es, de, fr, it, pt, pl, ja, nl, ar, tr, cs, vi, fa, hu, el, ro, sv, uk, fi, ko, da, bg, no
|
||||
| MGSM | 54.0 | 65.3 | zh, en, bn, de, es, fr, ja, ru, sw, te, th
|
||||
| XWinograd | 61.7 | 73.1 | zh, en, fr, jp, ru, pt
|
||||
| XStoryCloze | 84.7 | 90.7 | zh, en, ar, es, eu, hi, id, my, ru, sw, te
|
||||
| XCOPA | 73.3 | 80.1 | zh, et, ht, id, it, qu, sw, ta, th, tr, vi
|
||||
|
||||
### 工具调用能力
|
||||
|
||||
我们在 [Berkeley Function Calling Leaderboard](https://github.com/ShishirPatil/gorilla/tree/main/berkeley-function-call-leaderboard)
|
||||
上进行了测试并得到了以下结果:
|
||||
|
||||
| Model | Overall Acc. | AST Summary | Exec Summary | Relevance |
|
||||
|:-----------------------|:------------:|:-----------:|:------------:|:---------:|
|
||||
| Llama-3-8B-Instruct | 58.88 | 59.25 | 70.01 | 45.83 |
|
||||
| gpt-4-turbo-2024-04-09 | 81.24 | 82.14 | 78.61 | 88.75 |
|
||||
| ChatGLM3-6B | 57.88 | 62.18 | 69.78 | 5.42 |
|
||||
| GLM-4-9B-Chat | 81.00 | 80.26 | 84.40 | 87.92 |
|
||||
|
||||
**本仓库是 GLM-4-9B-Chat 的模型仓库,支持`128K`上下文长度。**
|
||||
|
||||
## 运行模型
|
||||
|
||||
**更多推理代码和依赖信息,请访问我们的 [github](https://github.com/THUDM/GLM-4)。**
|
||||
|
||||
**请严格按照[依赖](https://github.com/THUDM/GLM-4/blob/main/basic_demo/requirements.txt)安装,否则无法正常运行。**
|
||||
|
||||
### 使用 transformers 后端进行推理:
|
||||
|
||||
```python
|
||||
import torch
|
||||
from transformers import AutoModelForCausalLM, AutoTokenizer
|
||||
|
||||
device = "cuda"
|
||||
|
||||
tokenizer = AutoTokenizer.from_pretrained("THUDM/glm-4-9b-chat", trust_remote_code=True)
|
||||
|
||||
query = "你好"
|
||||
|
||||
inputs = tokenizer.apply_chat_template([{"role": "user", "content": query}],
|
||||
add_generation_prompt=True,
|
||||
tokenize=True,
|
||||
return_tensors="pt",
|
||||
return_dict=True
|
||||
)
|
||||
|
||||
inputs = inputs.to(device)
|
||||
model = AutoModelForCausalLM.from_pretrained(
|
||||
"THUDM/glm-4-9b-chat",
|
||||
torch_dtype=torch.bfloat16,
|
||||
low_cpu_mem_usage=True,
|
||||
trust_remote_code=True
|
||||
).to(device).eval()
|
||||
|
||||
gen_kwargs = {"max_length": 2500, "do_sample": True, "top_k": 1}
|
||||
with torch.no_grad():
|
||||
outputs = model.generate(**inputs, **gen_kwargs)
|
||||
outputs = outputs[:, inputs['input_ids'].shape[1]:]
|
||||
print(tokenizer.decode(outputs[0], skip_special_tokens=True))
|
||||
```
|
||||
|
||||
使用 vLLM后端进行推理:
|
||||
|
||||
```python
|
||||
from transformers import AutoTokenizer
|
||||
from vllm import LLM, SamplingParams
|
||||
|
||||
# GLM-4-9B-Chat-1M
|
||||
# max_model_len, tp_size = 1048576, 4
|
||||
|
||||
# GLM-4-9B-Chat
|
||||
# 如果遇见 OOM 现象,建议减少max_model_len,或者增加tp_size
|
||||
max_model_len, tp_size = 131072, 1
|
||||
model_name = "THUDM/glm-4-9b-chat"
|
||||
prompt = [{"role": "user", "content": "你好"}]
|
||||
|
||||
tokenizer = AutoTokenizer.from_pretrained(model_name, trust_remote_code=True)
|
||||
llm = LLM(
|
||||
model=model_name,
|
||||
tensor_parallel_size=tp_size,
|
||||
max_model_len=max_model_len,
|
||||
trust_remote_code=True,
|
||||
enforce_eager=True,
|
||||
# GLM-4-9B-Chat-1M 如果遇见 OOM 现象,建议开启下述参数
|
||||
# enable_chunked_prefill=True,
|
||||
# max_num_batched_tokens=8192
|
||||
)
|
||||
stop_token_ids = [151329, 151336, 151338]
|
||||
sampling_params = SamplingParams(temperature=0.95, max_tokens=1024, stop_token_ids=stop_token_ids)
|
||||
|
||||
inputs = tokenizer.apply_chat_template(prompt, tokenize=False, add_generation_prompt=True)
|
||||
outputs = llm.generate(prompts=inputs, sampling_params=sampling_params)
|
||||
|
||||
print(outputs[0].outputs[0].text)
|
||||
```
|
||||
|
||||
## 协议
|
||||
|
||||
GLM-4
|
||||
模型的权重的使用则需要遵循 [LICENSE](https://modelscope.cn/models/ZhipuAI/glm-4-9b-chat/file/view/master?fileName=LICENSE&status=0)。
|
||||
|
||||
## 引用
|
||||
|
||||
如果你觉得我们的工作有帮助的话,请考虑引用下列论文。
|
||||
|
||||
```
|
||||
@misc{glm2024chatglm,
|
||||
title={ChatGLM: A Family of Large Language Models from GLM-130B to GLM-4 All Tools},
|
||||
author={Team GLM and Aohan Zeng and Bin Xu and Bowen Wang and Chenhui Zhang and Da Yin and Diego Rojas and Guanyu Feng and Hanlin Zhao and Hanyu Lai and Hao Yu and Hongning Wang and Jiadai Sun and Jiajie Zhang and Jiale Cheng and Jiayi Gui and Jie Tang and Jing Zhang and Juanzi Li and Lei Zhao and Lindong Wu and Lucen Zhong and Mingdao Liu and Minlie Huang and Peng Zhang and Qinkai Zheng and Rui Lu and Shuaiqi Duan and Shudan Zhang and Shulin Cao and Shuxun Yang and Weng Lam Tam and Wenyi Zhao and Xiao Liu and Xiao Xia and Xiaohan Zhang and Xiaotao Gu and Xin Lv and Xinghan Liu and Xinyi Liu and Xinyue Yang and Xixuan Song and Xunkai Zhang and Yifan An and Yifan Xu and Yilin Niu and Yuantao Yang and Yueyan Li and Yushi Bai and Yuxiao Dong and Zehan Qi and Zhaoyu Wang and Zhen Yang and Zhengxiao Du and Zhenyu Hou and Zihan Wang},
|
||||
year={2024},
|
||||
eprint={2406.12793},
|
||||
archivePrefix={arXiv},
|
||||
primaryClass={id='cs.CL' full_name='Computation and Language' is_active=True alt_name='cmp-lg' in_archive='cs' is_general=False description='Covers natural language processing. Roughly includes material in ACM Subject Class I.2.7. Note that work on artificial languages (programming languages, logics, formal systems) that does not explicitly address natural-language issues broadly construed (natural-language processing, computational linguistics, speech, text retrieval, etc.) is not appropriate for this area.'}
|
||||
}
|
||||
```
|
||||
glm4 9b
|
168
README_en.md
168
README_en.md
|
@ -1,168 +0,0 @@
|
|||
# GLM-4-9B-Chat
|
||||
|
||||
Please note that the GLM-4-9B series conversational models (excluding vision models) have been merged and standardized
|
||||
in Hugging Face transformers since version `4.46.0`. The models in this repository are no longer supported. Please refer
|
||||
to the [model repository](https://modelscope.cn/models/ZhipuAI/glm-4-9b-chat-hf) for the latest transformers-compatible
|
||||
models.
|
||||
|
||||
## Model Introduction
|
||||
|
||||
GLM-4-9B is the open-source version of the latest generation of pre-trained models in the GLM-4 series launched by Zhipu
|
||||
AI. In the evaluation of data sets in semantics, mathematics, reasoning, code, and knowledge, **GLM-4-9B**
|
||||
and its human preference-aligned version **GLM-4-9B-Chat** have shown superior performance beyond Llama-3-8B. In
|
||||
addition to multi-round conversations, GLM-4-9B-Chat also has advanced features such as web browsing, code execution,
|
||||
custom tool calls (Function Call), and long text
|
||||
reasoning (supporting up to 128K context). This generation of models has added multi-language support, supporting 26
|
||||
languages including Japanese, Korean, and German. We have also launched the **GLM-4-9B-Chat-1M** model that supports 1M
|
||||
context length (about 2 million Chinese characters) and the multimodal model GLM-4V-9B based on GLM-4-9B.
|
||||
**GLM-4V-9B** possesses dialogue capabilities in both Chinese and English at a high resolution of 1120*1120.
|
||||
In various multimodal evaluations, including comprehensive abilities in Chinese and English, perception & reasoning,
|
||||
text recognition, and chart understanding, GLM-4V-9B demonstrates superior performance compared to
|
||||
GPT-4-turbo-2024-04-09, Gemini 1.0 Pro, Qwen-VL-Max, and Claude 3 Opus.
|
||||
|
||||
## Benchmark
|
||||
|
||||
We evaluated the GLM-4-9B-Chat model on some classic tasks and obtained the following results:
|
||||
|
||||
| Model | AlignBench-v2 | MT-Bench | IFEval | MMLU | C-Eval | GSM8K | MATH | HumanEval | NCB |
|
||||
|:--------------------|:-------------:|:--------:|:------:|:----:|:------:|:-----:|:----:|:---------:|:----:|
|
||||
| Llama-3-8B-Instruct | 5.12 | 8.00 | 68.58 | 68.4 | 51.3 | 79.6 | 30.0 | 62.2 | 24.7 |
|
||||
| ChatGLM3-6B | 3.97 | 5.50 | 28.1 | 66.4 | 69.0 | 72.3 | 25.7 | 58.5 | 11.3 |
|
||||
| GLM-4-9B-Chat | 6.61 | 8.35 | 69.0 | 72.4 | 75.6 | 79.6 | 50.6 | 71.8 | 32.2 |
|
||||
|
||||
### Long Context
|
||||
|
||||
The [eval_needle experiment](https://github.com/LargeWorldModel/LWM/blob/main/scripts/eval_needle.py) was conducted with
|
||||
a context length of 1M, and the results are as follows:
|
||||
|
||||
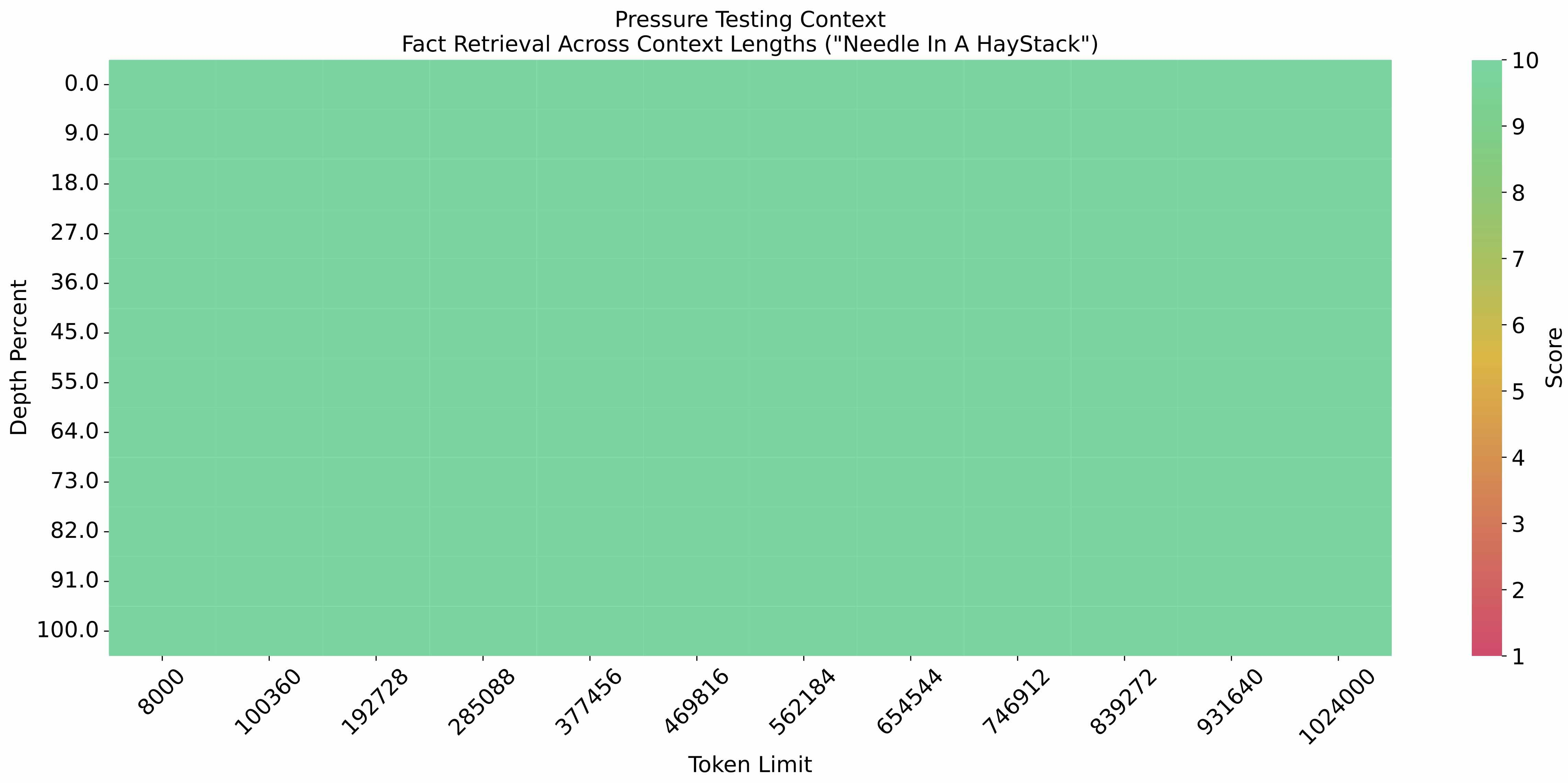
|
||||
|
||||
The long text capability was further evaluated on LongBench, and the results are as follows:
|
||||
|
||||
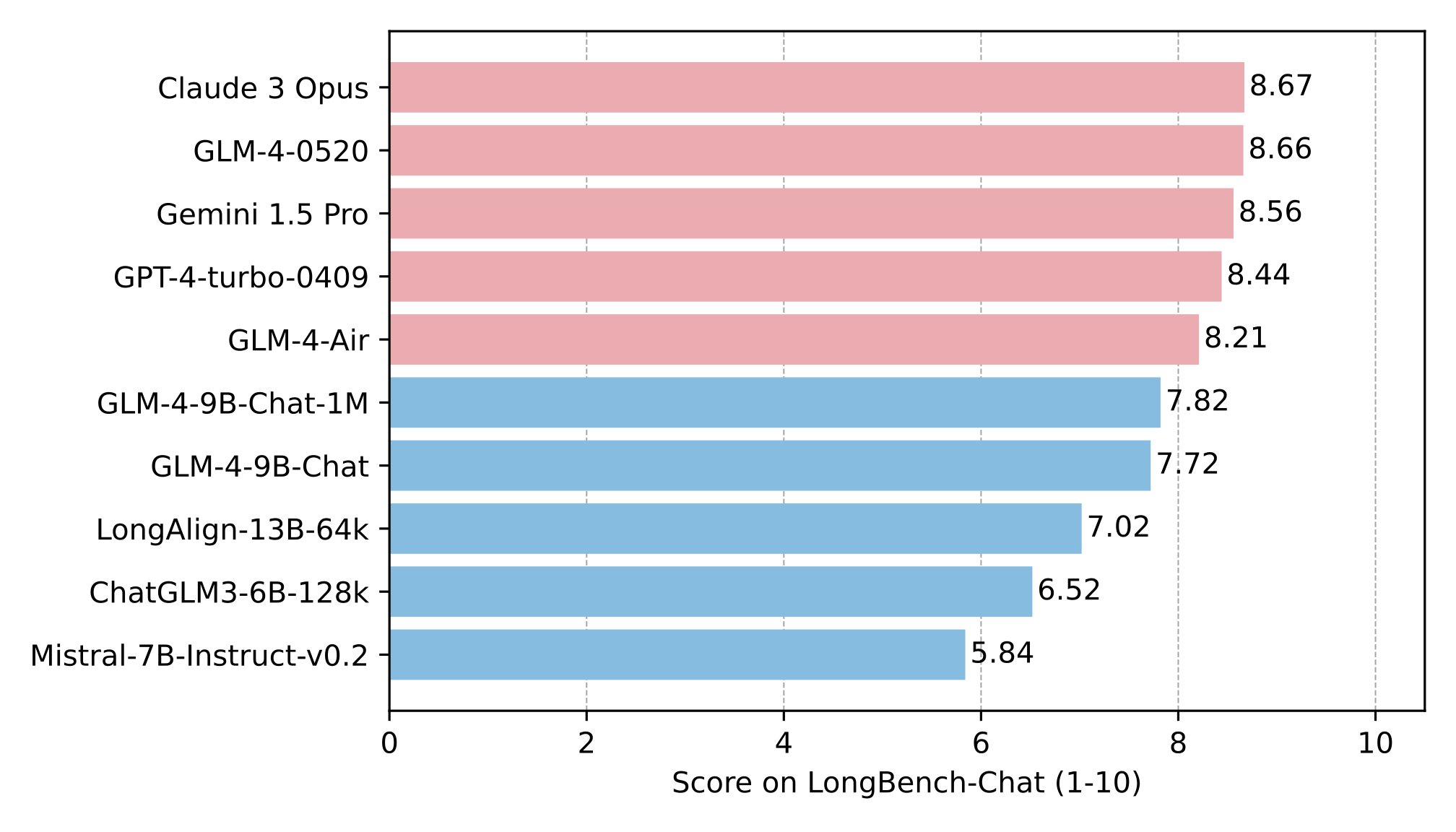
|
||||
|
||||
### Multi Language
|
||||
|
||||
The tests for GLM-4-9B-Chat and Llama-3-8B-Instruct are conducted on six multilingual datasets. The test results and the
|
||||
corresponding languages selected for each dataset are shown in the table below:
|
||||
|
||||
| Dataset | Llama-3-8B-Instruct | GLM-4-9B-Chat | Languages |
|
||||
|:------------|:-------------------:|:-------------:|:----------------------------------------------------------------------------------------------:|
|
||||
| M-MMLU | 49.6 | 56.6 | all |
|
||||
| FLORES | 25.0 | 28.8 | ru, es, de, fr, it, pt, pl, ja, nl, ar, tr, cs, vi, fa, hu, el, ro, sv, uk, fi, ko, da, bg, no |
|
||||
| MGSM | 54.0 | 65.3 | zh, en, bn, de, es, fr, ja, ru, sw, te, th |
|
||||
| XWinograd | 61.7 | 73.1 | zh, en, fr, jp, ru, pt |
|
||||
| XStoryCloze | 84.7 | 90.7 | zh, en, ar, es, eu, hi, id, my, ru, sw, te |
|
||||
| XCOPA | 73.3 | 80.1 | zh, et, ht, id, it, qu, sw, ta, th, tr, vi |
|
||||
|
||||
### Function Call
|
||||
|
||||
Tested
|
||||
on [Berkeley Function Calling Leaderboard](https://github.com/ShishirPatil/gorilla/tree/main/berkeley-function-call-leaderboard).
|
||||
|
||||
| Model | Overall Acc. | AST Summary | Exec Summary | Relevance |
|
||||
|:-----------------------|:------------:|:-----------:|:------------:|:---------:|
|
||||
| Llama-3-8B-Instruct | 58.88 | 59.25 | 70.01 | 45.83 |
|
||||
| gpt-4-turbo-2024-04-09 | 81.24 | 82.14 | 78.61 | 88.75 |
|
||||
| ChatGLM3-6B | 57.88 | 62.18 | 69.78 | 5.42 |
|
||||
| GLM-4-9B-Chat | 81.00 | 80.26 | 84.40 | 87.92 |
|
||||
|
||||
**This repository is the model repository of GLM-4-9B-Chat, supporting `128K` context length.**
|
||||
|
||||
## Quick Start
|
||||
|
||||
**For more inference code and requirements, please visit our [github page](https://github.com/THUDM/GLM-4).**
|
||||
|
||||
**Please strictly follow the [dependencies](https://github.com/THUDM/GLM-4/blob/main/basic_demo/requirements.txt) to
|
||||
install, otherwise it will not run properly**
|
||||
|
||||
### Use the following method to quickly call the GLM-4-9B-Chat language model
|
||||
|
||||
Use the transformers backend for inference:
|
||||
|
||||
```python
|
||||
import torch
|
||||
from transformers import AutoModelForCausalLM, AutoTokenizer
|
||||
|
||||
device = "cuda"
|
||||
|
||||
tokenizer = AutoTokenizer.from_pretrained("THUDM/glm-4-9b-chat", trust_remote_code=True)
|
||||
|
||||
query = "Hello"
|
||||
|
||||
inputs = tokenizer.apply_chat_template([{"role": "user", "content": query}],
|
||||
add_generation_prompt=True,
|
||||
tokenize=True,
|
||||
return_tensors="pt",
|
||||
return_dict=True
|
||||
)
|
||||
|
||||
inputs = inputs.to(device)
|
||||
model = AutoModelForCausalLM.from_pretrained(
|
||||
"THUDM/glm-4-9b-chat",
|
||||
torch_dtype=torch.bfloat16,
|
||||
low_cpu_mem_usage=True,
|
||||
trust_remote_code=True
|
||||
).to(device).eval()
|
||||
|
||||
gen_kwargs = {"max_length": 2500, "do_sample": True, "top_k": 1}
|
||||
with torch.no_grad():
|
||||
outputs = model.generate(**inputs, **gen_kwargs)
|
||||
outputs = outputs[:, inputs['input_ids'].shape[1]:]
|
||||
print(tokenizer.decode(outputs[0], skip_special_tokens=True))
|
||||
```
|
||||
|
||||
Use the vLLM backend for inference:
|
||||
|
||||
```python
|
||||
from transformers import AutoTokenizer
|
||||
from vllm import LLM, SamplingParams
|
||||
|
||||
# GLM-4-9B-Chat-1M
|
||||
# max_model_len, tp_size = 1048576, 4
|
||||
|
||||
# GLM-4-9B-Chat
|
||||
# If you encounter OOM, it is recommended to reduce max_model_len or increase tp_size
|
||||
max_model_len, tp_size = 131072, 1
|
||||
model_name = "THUDM/glm-4-9b-chat"
|
||||
prompt = [{"role": "user", "content": "hello"}]
|
||||
|
||||
tokenizer = AutoTokenizer.from_pretrained(model_name, trust_remote_code=True)
|
||||
llm = LLM(
|
||||
model=model_name,
|
||||
tensor_parallel_size=tp_size,
|
||||
max_model_len=max_model_len,
|
||||
trust_remote_code=True,
|
||||
enforce_eager=True,
|
||||
# GLM-4-9B-Chat-1M If you encounter OOM phenomenon, it is recommended to enable the following parameters
|
||||
# enable_chunked_prefill=True,
|
||||
# max_num_batched_tokens=8192
|
||||
)
|
||||
stop_token_ids = [151329, 151336, 151338]
|
||||
sampling_params = SamplingParams(temperature=0.95, max_tokens=1024, stop_token_ids=stop_token_ids)
|
||||
|
||||
inputs = tokenizer.apply_chat_template(prompt, tokenize=False, add_generation_prompt=True)
|
||||
outputs = llm.generate(prompts=inputs, sampling_params=sampling_params)
|
||||
|
||||
print(outputs[0].outputs[0].text)
|
||||
```
|
||||
|
||||
## LICENSE
|
||||
|
||||
The weights of the GLM-4 model are available under the terms
|
||||
of [LICENSE](https://modelscope.cn/models/ZhipuAI/glm-4-9b-chat/file/view/master?fileName=LICENSE&status=0).
|
||||
|
||||
## Citations
|
||||
|
||||
If you find our work useful, please consider citing the following paper.
|
||||
|
||||
```
|
||||
@misc{glm2024chatglm,
|
||||
title={ChatGLM: A Family of Large Language Models from GLM-130B to GLM-4 All Tools},
|
||||
author={Team GLM and Aohan Zeng and Bin Xu and Bowen Wang and Chenhui Zhang and Da Yin and Diego Rojas and Guanyu Feng and Hanlin Zhao and Hanyu Lai and Hao Yu and Hongning Wang and Jiadai Sun and Jiajie Zhang and Jiale Cheng and Jiayi Gui and Jie Tang and Jing Zhang and Juanzi Li and Lei Zhao and Lindong Wu and Lucen Zhong and Mingdao Liu and Minlie Huang and Peng Zhang and Qinkai Zheng and Rui Lu and Shuaiqi Duan and Shudan Zhang and Shulin Cao and Shuxun Yang and Weng Lam Tam and Wenyi Zhao and Xiao Liu and Xiao Xia and Xiaohan Zhang and Xiaotao Gu and Xin Lv and Xinghan Liu and Xinyi Liu and Xinyue Yang and Xixuan Song and Xunkai Zhang and Yifan An and Yifan Xu and Yilin Niu and Yuantao Yang and Yueyan Li and Yushi Bai and Yuxiao Dong and Zehan Qi and Zhaoyu Wang and Zhen Yang and Zhengxiao Du and Zhenyu Hou and Zihan Wang},
|
||||
year={2024},
|
||||
eprint={2406.12793},
|
||||
archivePrefix={arXiv},
|
||||
primaryClass={id='cs.CL' full_name='Computation and Language' is_active=True alt_name='cmp-lg' in_archive='cs' is_general=False description='Covers natural language processing. Roughly includes material in ACM Subject Class I.2.7. Note that work on artificial languages (programming languages, logics, formal systems) that does not explicitly address natural-language issues broadly construed (natural-language processing, computational linguistics, speech, text retrieval, etc.) is not appropriate for this area.'}
|
||||
}
|
||||
```
|
45
config.json
45
config.json
|
@ -1,45 +0,0 @@
|
|||
{
|
||||
"_name_or_path": "THUDM/glm-4-9b-chat",
|
||||
"model_type": "chatglm",
|
||||
"architectures": [
|
||||
"ChatGLMModel"
|
||||
],
|
||||
"auto_map": {
|
||||
"AutoConfig": "configuration_chatglm.ChatGLMConfig",
|
||||
"AutoModel": "modeling_chatglm.ChatGLMForConditionalGeneration",
|
||||
"AutoModelForCausalLM": "modeling_chatglm.ChatGLMForConditionalGeneration",
|
||||
"AutoModelForSeq2SeqLM": "modeling_chatglm.ChatGLMForConditionalGeneration",
|
||||
"AutoModelForSequenceClassification": "modeling_chatglm.ChatGLMForSequenceClassification"
|
||||
},
|
||||
"add_bias_linear": false,
|
||||
"add_qkv_bias": true,
|
||||
"apply_query_key_layer_scaling": true,
|
||||
"apply_residual_connection_post_layernorm": false,
|
||||
"attention_dropout": 0.0,
|
||||
"attention_softmax_in_fp32": true,
|
||||
"attn_implementation": "sdpa",
|
||||
"bias_dropout_fusion": true,
|
||||
"ffn_hidden_size": 13696,
|
||||
"fp32_residual_connection": false,
|
||||
"hidden_dropout": 0.0,
|
||||
"hidden_size": 4096,
|
||||
"kv_channels": 128,
|
||||
"layernorm_epsilon": 1.5625e-07,
|
||||
"multi_query_attention": true,
|
||||
"multi_query_group_num": 2,
|
||||
"num_attention_heads": 32,
|
||||
"num_hidden_layers": 40,
|
||||
"num_layers": 40,
|
||||
"rope_ratio": 500,
|
||||
"original_rope": true,
|
||||
"padded_vocab_size": 151552,
|
||||
"post_layer_norm": true,
|
||||
"rmsnorm": true,
|
||||
"seq_length": 131072,
|
||||
"use_cache": true,
|
||||
"torch_dtype": "bfloat16",
|
||||
"transformers_version": "4.44.0",
|
||||
"tie_word_embeddings": false,
|
||||
"eos_token_id": [151329, 151336, 151338],
|
||||
"pad_token_id": 151329
|
||||
}
|
|
@ -1 +0,0 @@
|
|||
{"framework":"Pytorch","task":"nli"}
|
|
@ -1,58 +0,0 @@
|
|||
from transformers import PretrainedConfig
|
||||
|
||||
|
||||
class ChatGLMConfig(PretrainedConfig):
|
||||
model_type = "chatglm"
|
||||
|
||||
def __init__(
|
||||
self,
|
||||
num_layers=28,
|
||||
padded_vocab_size=65024,
|
||||
hidden_size=4096,
|
||||
ffn_hidden_size=13696,
|
||||
kv_channels=128,
|
||||
num_attention_heads=32,
|
||||
seq_length=2048,
|
||||
hidden_dropout=0.0,
|
||||
classifier_dropout=None,
|
||||
attention_dropout=0.0,
|
||||
layernorm_epsilon=1e-5,
|
||||
rmsnorm=True,
|
||||
apply_residual_connection_post_layernorm=False,
|
||||
post_layer_norm=True,
|
||||
add_bias_linear=False,
|
||||
add_qkv_bias=False,
|
||||
bias_dropout_fusion=True,
|
||||
multi_query_attention=False,
|
||||
multi_query_group_num=1,
|
||||
rope_ratio=1,
|
||||
apply_query_key_layer_scaling=True,
|
||||
attention_softmax_in_fp32=True,
|
||||
fp32_residual_connection=False,
|
||||
**kwargs
|
||||
):
|
||||
self.num_layers = num_layers
|
||||
self.vocab_size = padded_vocab_size
|
||||
self.padded_vocab_size = padded_vocab_size
|
||||
self.hidden_size = hidden_size
|
||||
self.ffn_hidden_size = ffn_hidden_size
|
||||
self.kv_channels = kv_channels
|
||||
self.num_attention_heads = num_attention_heads
|
||||
self.seq_length = seq_length
|
||||
self.hidden_dropout = hidden_dropout
|
||||
self.classifier_dropout = classifier_dropout
|
||||
self.attention_dropout = attention_dropout
|
||||
self.layernorm_epsilon = layernorm_epsilon
|
||||
self.rmsnorm = rmsnorm
|
||||
self.apply_residual_connection_post_layernorm = apply_residual_connection_post_layernorm
|
||||
self.post_layer_norm = post_layer_norm
|
||||
self.add_bias_linear = add_bias_linear
|
||||
self.add_qkv_bias = add_qkv_bias
|
||||
self.bias_dropout_fusion = bias_dropout_fusion
|
||||
self.multi_query_attention = multi_query_attention
|
||||
self.multi_query_group_num = multi_query_group_num
|
||||
self.rope_ratio = rope_ratio
|
||||
self.apply_query_key_layer_scaling = apply_query_key_layer_scaling
|
||||
self.attention_softmax_in_fp32 = attention_softmax_in_fp32
|
||||
self.fp32_residual_connection = fp32_residual_connection
|
||||
super().__init__(**kwargs)
|
|
@ -1,13 +0,0 @@
|
|||
{
|
||||
"eos_token_id": [
|
||||
151329,
|
||||
151336,
|
||||
151338
|
||||
],
|
||||
"pad_token_id": 151329,
|
||||
"do_sample": true,
|
||||
"temperature": 0.8,
|
||||
"max_length": 128000,
|
||||
"top_p": 0.8,
|
||||
"transformers_version": "4.40.2"
|
||||
}
|
BIN
model-00001-of-00010.safetensors (Stored with Git LFS)
BIN
model-00001-of-00010.safetensors (Stored with Git LFS)
Binary file not shown.
BIN
model-00002-of-00010.safetensors (Stored with Git LFS)
BIN
model-00002-of-00010.safetensors (Stored with Git LFS)
Binary file not shown.
BIN
model-00003-of-00010.safetensors (Stored with Git LFS)
BIN
model-00003-of-00010.safetensors (Stored with Git LFS)
Binary file not shown.
BIN
model-00004-of-00010.safetensors (Stored with Git LFS)
BIN
model-00004-of-00010.safetensors (Stored with Git LFS)
Binary file not shown.
BIN
model-00005-of-00010.safetensors (Stored with Git LFS)
BIN
model-00005-of-00010.safetensors (Stored with Git LFS)
Binary file not shown.
BIN
model-00006-of-00010.safetensors (Stored with Git LFS)
BIN
model-00006-of-00010.safetensors (Stored with Git LFS)
Binary file not shown.
BIN
model-00007-of-00010.safetensors (Stored with Git LFS)
BIN
model-00007-of-00010.safetensors (Stored with Git LFS)
Binary file not shown.
BIN
model-00008-of-00010.safetensors (Stored with Git LFS)
BIN
model-00008-of-00010.safetensors (Stored with Git LFS)
Binary file not shown.
BIN
model-00009-of-00010.safetensors (Stored with Git LFS)
BIN
model-00009-of-00010.safetensors (Stored with Git LFS)
Binary file not shown.
BIN
model-00010-of-00010.safetensors (Stored with Git LFS)
BIN
model-00010-of-00010.safetensors (Stored with Git LFS)
Binary file not shown.
|
@ -1,291 +0,0 @@
|
|||
{
|
||||
"metadata": {
|
||||
"total_size": 18799902784
|
||||
},
|
||||
"weight_map": {
|
||||
"transformer.embedding.word_embeddings.weight": "model-00001-of-00010.safetensors",
|
||||
"transformer.encoder.final_layernorm.weight": "model-00010-of-00010.safetensors",
|
||||
"transformer.encoder.layers.0.input_layernorm.weight": "model-00001-of-00010.safetensors",
|
||||
"transformer.encoder.layers.0.mlp.dense_4h_to_h.weight": "model-00001-of-00010.safetensors",
|
||||
"transformer.encoder.layers.0.mlp.dense_h_to_4h.weight": "model-00001-of-00010.safetensors",
|
||||
"transformer.encoder.layers.0.post_attention_layernorm.weight": "model-00001-of-00010.safetensors",
|
||||
"transformer.encoder.layers.0.self_attention.dense.weight": "model-00001-of-00010.safetensors",
|
||||
"transformer.encoder.layers.0.self_attention.query_key_value.bias": "model-00001-of-00010.safetensors",
|
||||
"transformer.encoder.layers.0.self_attention.query_key_value.weight": "model-00001-of-00010.safetensors",
|
||||
"transformer.encoder.layers.1.input_layernorm.weight": "model-00001-of-00010.safetensors",
|
||||
"transformer.encoder.layers.1.mlp.dense_4h_to_h.weight": "model-00002-of-00010.safetensors",
|
||||
"transformer.encoder.layers.1.mlp.dense_h_to_4h.weight": "model-00001-of-00010.safetensors",
|
||||
"transformer.encoder.layers.1.post_attention_layernorm.weight": "model-00001-of-00010.safetensors",
|
||||
"transformer.encoder.layers.1.self_attention.dense.weight": "model-00001-of-00010.safetensors",
|
||||
"transformer.encoder.layers.1.self_attention.query_key_value.bias": "model-00001-of-00010.safetensors",
|
||||
"transformer.encoder.layers.1.self_attention.query_key_value.weight": "model-00001-of-00010.safetensors",
|
||||
"transformer.encoder.layers.10.input_layernorm.weight": "model-00003-of-00010.safetensors",
|
||||
"transformer.encoder.layers.10.mlp.dense_4h_to_h.weight": "model-00003-of-00010.safetensors",
|
||||
"transformer.encoder.layers.10.mlp.dense_h_to_4h.weight": "model-00003-of-00010.safetensors",
|
||||
"transformer.encoder.layers.10.post_attention_layernorm.weight": "model-00003-of-00010.safetensors",
|
||||
"transformer.encoder.layers.10.self_attention.dense.weight": "model-00003-of-00010.safetensors",
|
||||
"transformer.encoder.layers.10.self_attention.query_key_value.bias": "model-00003-of-00010.safetensors",
|
||||
"transformer.encoder.layers.10.self_attention.query_key_value.weight": "model-00003-of-00010.safetensors",
|
||||
"transformer.encoder.layers.11.input_layernorm.weight": "model-00003-of-00010.safetensors",
|
||||
"transformer.encoder.layers.11.mlp.dense_4h_to_h.weight": "model-00004-of-00010.safetensors",
|
||||
"transformer.encoder.layers.11.mlp.dense_h_to_4h.weight": "model-00004-of-00010.safetensors",
|
||||
"transformer.encoder.layers.11.post_attention_layernorm.weight": "model-00004-of-00010.safetensors",
|
||||
"transformer.encoder.layers.11.self_attention.dense.weight": "model-00004-of-00010.safetensors",
|
||||
"transformer.encoder.layers.11.self_attention.query_key_value.bias": "model-00004-of-00010.safetensors",
|
||||
"transformer.encoder.layers.11.self_attention.query_key_value.weight": "model-00004-of-00010.safetensors",
|
||||
"transformer.encoder.layers.12.input_layernorm.weight": "model-00004-of-00010.safetensors",
|
||||
"transformer.encoder.layers.12.mlp.dense_4h_to_h.weight": "model-00004-of-00010.safetensors",
|
||||
"transformer.encoder.layers.12.mlp.dense_h_to_4h.weight": "model-00004-of-00010.safetensors",
|
||||
"transformer.encoder.layers.12.post_attention_layernorm.weight": "model-00004-of-00010.safetensors",
|
||||
"transformer.encoder.layers.12.self_attention.dense.weight": "model-00004-of-00010.safetensors",
|
||||
"transformer.encoder.layers.12.self_attention.query_key_value.bias": "model-00004-of-00010.safetensors",
|
||||
"transformer.encoder.layers.12.self_attention.query_key_value.weight": "model-00004-of-00010.safetensors",
|
||||
"transformer.encoder.layers.13.input_layernorm.weight": "model-00004-of-00010.safetensors",
|
||||
"transformer.encoder.layers.13.mlp.dense_4h_to_h.weight": "model-00004-of-00010.safetensors",
|
||||
"transformer.encoder.layers.13.mlp.dense_h_to_4h.weight": "model-00004-of-00010.safetensors",
|
||||
"transformer.encoder.layers.13.post_attention_layernorm.weight": "model-00004-of-00010.safetensors",
|
||||
"transformer.encoder.layers.13.self_attention.dense.weight": "model-00004-of-00010.safetensors",
|
||||
"transformer.encoder.layers.13.self_attention.query_key_value.bias": "model-00004-of-00010.safetensors",
|
||||
"transformer.encoder.layers.13.self_attention.query_key_value.weight": "model-00004-of-00010.safetensors",
|
||||
"transformer.encoder.layers.14.input_layernorm.weight": "model-00004-of-00010.safetensors",
|
||||
"transformer.encoder.layers.14.mlp.dense_4h_to_h.weight": "model-00004-of-00010.safetensors",
|
||||
"transformer.encoder.layers.14.mlp.dense_h_to_4h.weight": "model-00004-of-00010.safetensors",
|
||||
"transformer.encoder.layers.14.post_attention_layernorm.weight": "model-00004-of-00010.safetensors",
|
||||
"transformer.encoder.layers.14.self_attention.dense.weight": "model-00004-of-00010.safetensors",
|
||||
"transformer.encoder.layers.14.self_attention.query_key_value.bias": "model-00004-of-00010.safetensors",
|
||||
"transformer.encoder.layers.14.self_attention.query_key_value.weight": "model-00004-of-00010.safetensors",
|
||||
"transformer.encoder.layers.15.input_layernorm.weight": "model-00004-of-00010.safetensors",
|
||||
"transformer.encoder.layers.15.mlp.dense_4h_to_h.weight": "model-00005-of-00010.safetensors",
|
||||
"transformer.encoder.layers.15.mlp.dense_h_to_4h.weight": "model-00004-of-00010.safetensors",
|
||||
"transformer.encoder.layers.15.post_attention_layernorm.weight": "model-00004-of-00010.safetensors",
|
||||
"transformer.encoder.layers.15.self_attention.dense.weight": "model-00004-of-00010.safetensors",
|
||||
"transformer.encoder.layers.15.self_attention.query_key_value.bias": "model-00004-of-00010.safetensors",
|
||||
"transformer.encoder.layers.15.self_attention.query_key_value.weight": "model-00004-of-00010.safetensors",
|
||||
"transformer.encoder.layers.16.input_layernorm.weight": "model-00005-of-00010.safetensors",
|
||||
"transformer.encoder.layers.16.mlp.dense_4h_to_h.weight": "model-00005-of-00010.safetensors",
|
||||
"transformer.encoder.layers.16.mlp.dense_h_to_4h.weight": "model-00005-of-00010.safetensors",
|
||||
"transformer.encoder.layers.16.post_attention_layernorm.weight": "model-00005-of-00010.safetensors",
|
||||
"transformer.encoder.layers.16.self_attention.dense.weight": "model-00005-of-00010.safetensors",
|
||||
"transformer.encoder.layers.16.self_attention.query_key_value.bias": "model-00005-of-00010.safetensors",
|
||||
"transformer.encoder.layers.16.self_attention.query_key_value.weight": "model-00005-of-00010.safetensors",
|
||||
"transformer.encoder.layers.17.input_layernorm.weight": "model-00005-of-00010.safetensors",
|
||||
"transformer.encoder.layers.17.mlp.dense_4h_to_h.weight": "model-00005-of-00010.safetensors",
|
||||
"transformer.encoder.layers.17.mlp.dense_h_to_4h.weight": "model-00005-of-00010.safetensors",
|
||||
"transformer.encoder.layers.17.post_attention_layernorm.weight": "model-00005-of-00010.safetensors",
|
||||
"transformer.encoder.layers.17.self_attention.dense.weight": "model-00005-of-00010.safetensors",
|
||||
"transformer.encoder.layers.17.self_attention.query_key_value.bias": "model-00005-of-00010.safetensors",
|
||||
"transformer.encoder.layers.17.self_attention.query_key_value.weight": "model-00005-of-00010.safetensors",
|
||||
"transformer.encoder.layers.18.input_layernorm.weight": "model-00005-of-00010.safetensors",
|
||||
"transformer.encoder.layers.18.mlp.dense_4h_to_h.weight": "model-00005-of-00010.safetensors",
|
||||
"transformer.encoder.layers.18.mlp.dense_h_to_4h.weight": "model-00005-of-00010.safetensors",
|
||||
"transformer.encoder.layers.18.post_attention_layernorm.weight": "model-00005-of-00010.safetensors",
|
||||
"transformer.encoder.layers.18.self_attention.dense.weight": "model-00005-of-00010.safetensors",
|
||||
"transformer.encoder.layers.18.self_attention.query_key_value.bias": "model-00005-of-00010.safetensors",
|
||||
"transformer.encoder.layers.18.self_attention.query_key_value.weight": "model-00005-of-00010.safetensors",
|
||||
"transformer.encoder.layers.19.input_layernorm.weight": "model-00005-of-00010.safetensors",
|
||||
"transformer.encoder.layers.19.mlp.dense_4h_to_h.weight": "model-00005-of-00010.safetensors",
|
||||
"transformer.encoder.layers.19.mlp.dense_h_to_4h.weight": "model-00005-of-00010.safetensors",
|
||||
"transformer.encoder.layers.19.post_attention_layernorm.weight": "model-00005-of-00010.safetensors",
|
||||
"transformer.encoder.layers.19.self_attention.dense.weight": "model-00005-of-00010.safetensors",
|
||||
"transformer.encoder.layers.19.self_attention.query_key_value.bias": "model-00005-of-00010.safetensors",
|
||||
"transformer.encoder.layers.19.self_attention.query_key_value.weight": "model-00005-of-00010.safetensors",
|
||||
"transformer.encoder.layers.2.input_layernorm.weight": "model-00002-of-00010.safetensors",
|
||||
"transformer.encoder.layers.2.mlp.dense_4h_to_h.weight": "model-00002-of-00010.safetensors",
|
||||
"transformer.encoder.layers.2.mlp.dense_h_to_4h.weight": "model-00002-of-00010.safetensors",
|
||||
"transformer.encoder.layers.2.post_attention_layernorm.weight": "model-00002-of-00010.safetensors",
|
||||
"transformer.encoder.layers.2.self_attention.dense.weight": "model-00002-of-00010.safetensors",
|
||||
"transformer.encoder.layers.2.self_attention.query_key_value.bias": "model-00002-of-00010.safetensors",
|
||||
"transformer.encoder.layers.2.self_attention.query_key_value.weight": "model-00002-of-00010.safetensors",
|
||||
"transformer.encoder.layers.20.input_layernorm.weight": "model-00005-of-00010.safetensors",
|
||||
"transformer.encoder.layers.20.mlp.dense_4h_to_h.weight": "model-00006-of-00010.safetensors",
|
||||
"transformer.encoder.layers.20.mlp.dense_h_to_4h.weight": "model-00006-of-00010.safetensors",
|
||||
"transformer.encoder.layers.20.post_attention_layernorm.weight": "model-00005-of-00010.safetensors",
|
||||
"transformer.encoder.layers.20.self_attention.dense.weight": "model-00005-of-00010.safetensors",
|
||||
"transformer.encoder.layers.20.self_attention.query_key_value.bias": "model-00005-of-00010.safetensors",
|
||||
"transformer.encoder.layers.20.self_attention.query_key_value.weight": "model-00005-of-00010.safetensors",
|
||||
"transformer.encoder.layers.21.input_layernorm.weight": "model-00006-of-00010.safetensors",
|
||||
"transformer.encoder.layers.21.mlp.dense_4h_to_h.weight": "model-00006-of-00010.safetensors",
|
||||
"transformer.encoder.layers.21.mlp.dense_h_to_4h.weight": "model-00006-of-00010.safetensors",
|
||||
"transformer.encoder.layers.21.post_attention_layernorm.weight": "model-00006-of-00010.safetensors",
|
||||
"transformer.encoder.layers.21.self_attention.dense.weight": "model-00006-of-00010.safetensors",
|
||||
"transformer.encoder.layers.21.self_attention.query_key_value.bias": "model-00006-of-00010.safetensors",
|
||||
"transformer.encoder.layers.21.self_attention.query_key_value.weight": "model-00006-of-00010.safetensors",
|
||||
"transformer.encoder.layers.22.input_layernorm.weight": "model-00006-of-00010.safetensors",
|
||||
"transformer.encoder.layers.22.mlp.dense_4h_to_h.weight": "model-00006-of-00010.safetensors",
|
||||
"transformer.encoder.layers.22.mlp.dense_h_to_4h.weight": "model-00006-of-00010.safetensors",
|
||||
"transformer.encoder.layers.22.post_attention_layernorm.weight": "model-00006-of-00010.safetensors",
|
||||
"transformer.encoder.layers.22.self_attention.dense.weight": "model-00006-of-00010.safetensors",
|
||||
"transformer.encoder.layers.22.self_attention.query_key_value.bias": "model-00006-of-00010.safetensors",
|
||||
"transformer.encoder.layers.22.self_attention.query_key_value.weight": "model-00006-of-00010.safetensors",
|
||||
"transformer.encoder.layers.23.input_layernorm.weight": "model-00006-of-00010.safetensors",
|
||||
"transformer.encoder.layers.23.mlp.dense_4h_to_h.weight": "model-00006-of-00010.safetensors",
|
||||
"transformer.encoder.layers.23.mlp.dense_h_to_4h.weight": "model-00006-of-00010.safetensors",
|
||||
"transformer.encoder.layers.23.post_attention_layernorm.weight": "model-00006-of-00010.safetensors",
|
||||
"transformer.encoder.layers.23.self_attention.dense.weight": "model-00006-of-00010.safetensors",
|
||||
"transformer.encoder.layers.23.self_attention.query_key_value.bias": "model-00006-of-00010.safetensors",
|
||||
"transformer.encoder.layers.23.self_attention.query_key_value.weight": "model-00006-of-00010.safetensors",
|
||||
"transformer.encoder.layers.24.input_layernorm.weight": "model-00006-of-00010.safetensors",
|
||||
"transformer.encoder.layers.24.mlp.dense_4h_to_h.weight": "model-00006-of-00010.safetensors",
|
||||
"transformer.encoder.layers.24.mlp.dense_h_to_4h.weight": "model-00006-of-00010.safetensors",
|
||||
"transformer.encoder.layers.24.post_attention_layernorm.weight": "model-00006-of-00010.safetensors",
|
||||
"transformer.encoder.layers.24.self_attention.dense.weight": "model-00006-of-00010.safetensors",
|
||||
"transformer.encoder.layers.24.self_attention.query_key_value.bias": "model-00006-of-00010.safetensors",
|
||||
"transformer.encoder.layers.24.self_attention.query_key_value.weight": "model-00006-of-00010.safetensors",
|
||||
"transformer.encoder.layers.25.input_layernorm.weight": "model-00006-of-00010.safetensors",
|
||||
"transformer.encoder.layers.25.mlp.dense_4h_to_h.weight": "model-00007-of-00010.safetensors",
|
||||
"transformer.encoder.layers.25.mlp.dense_h_to_4h.weight": "model-00007-of-00010.safetensors",
|
||||
"transformer.encoder.layers.25.post_attention_layernorm.weight": "model-00007-of-00010.safetensors",
|
||||
"transformer.encoder.layers.25.self_attention.dense.weight": "model-00007-of-00010.safetensors",
|
||||
"transformer.encoder.layers.25.self_attention.query_key_value.bias": "model-00007-of-00010.safetensors",
|
||||
"transformer.encoder.layers.25.self_attention.query_key_value.weight": "model-00007-of-00010.safetensors",
|
||||
"transformer.encoder.layers.26.input_layernorm.weight": "model-00007-of-00010.safetensors",
|
||||
"transformer.encoder.layers.26.mlp.dense_4h_to_h.weight": "model-00007-of-00010.safetensors",
|
||||
"transformer.encoder.layers.26.mlp.dense_h_to_4h.weight": "model-00007-of-00010.safetensors",
|
||||
"transformer.encoder.layers.26.post_attention_layernorm.weight": "model-00007-of-00010.safetensors",
|
||||
"transformer.encoder.layers.26.self_attention.dense.weight": "model-00007-of-00010.safetensors",
|
||||
"transformer.encoder.layers.26.self_attention.query_key_value.bias": "model-00007-of-00010.safetensors",
|
||||
"transformer.encoder.layers.26.self_attention.query_key_value.weight": "model-00007-of-00010.safetensors",
|
||||
"transformer.encoder.layers.27.input_layernorm.weight": "model-00007-of-00010.safetensors",
|
||||
"transformer.encoder.layers.27.mlp.dense_4h_to_h.weight": "model-00007-of-00010.safetensors",
|
||||
"transformer.encoder.layers.27.mlp.dense_h_to_4h.weight": "model-00007-of-00010.safetensors",
|
||||
"transformer.encoder.layers.27.post_attention_layernorm.weight": "model-00007-of-00010.safetensors",
|
||||
"transformer.encoder.layers.27.self_attention.dense.weight": "model-00007-of-00010.safetensors",
|
||||
"transformer.encoder.layers.27.self_attention.query_key_value.bias": "model-00007-of-00010.safetensors",
|
||||
"transformer.encoder.layers.27.self_attention.query_key_value.weight": "model-00007-of-00010.safetensors",
|
||||
"transformer.encoder.layers.28.input_layernorm.weight": "model-00007-of-00010.safetensors",
|
||||
"transformer.encoder.layers.28.mlp.dense_4h_to_h.weight": "model-00007-of-00010.safetensors",
|
||||
"transformer.encoder.layers.28.mlp.dense_h_to_4h.weight": "model-00007-of-00010.safetensors",
|
||||
"transformer.encoder.layers.28.post_attention_layernorm.weight": "model-00007-of-00010.safetensors",
|
||||
"transformer.encoder.layers.28.self_attention.dense.weight": "model-00007-of-00010.safetensors",
|
||||
"transformer.encoder.layers.28.self_attention.query_key_value.bias": "model-00007-of-00010.safetensors",
|
||||
"transformer.encoder.layers.28.self_attention.query_key_value.weight": "model-00007-of-00010.safetensors",
|
||||
"transformer.encoder.layers.29.input_layernorm.weight": "model-00007-of-00010.safetensors",
|
||||
"transformer.encoder.layers.29.mlp.dense_4h_to_h.weight": "model-00008-of-00010.safetensors",
|
||||
"transformer.encoder.layers.29.mlp.dense_h_to_4h.weight": "model-00007-of-00010.safetensors",
|
||||
"transformer.encoder.layers.29.post_attention_layernorm.weight": "model-00007-of-00010.safetensors",
|
||||
"transformer.encoder.layers.29.self_attention.dense.weight": "model-00007-of-00010.safetensors",
|
||||
"transformer.encoder.layers.29.self_attention.query_key_value.bias": "model-00007-of-00010.safetensors",
|
||||
"transformer.encoder.layers.29.self_attention.query_key_value.weight": "model-00007-of-00010.safetensors",
|
||||
"transformer.encoder.layers.3.input_layernorm.weight": "model-00002-of-00010.safetensors",
|
||||
"transformer.encoder.layers.3.mlp.dense_4h_to_h.weight": "model-00002-of-00010.safetensors",
|
||||
"transformer.encoder.layers.3.mlp.dense_h_to_4h.weight": "model-00002-of-00010.safetensors",
|
||||
"transformer.encoder.layers.3.post_attention_layernorm.weight": "model-00002-of-00010.safetensors",
|
||||
"transformer.encoder.layers.3.self_attention.dense.weight": "model-00002-of-00010.safetensors",
|
||||
"transformer.encoder.layers.3.self_attention.query_key_value.bias": "model-00002-of-00010.safetensors",
|
||||
"transformer.encoder.layers.3.self_attention.query_key_value.weight": "model-00002-of-00010.safetensors",
|
||||
"transformer.encoder.layers.30.input_layernorm.weight": "model-00008-of-00010.safetensors",
|
||||
"transformer.encoder.layers.30.mlp.dense_4h_to_h.weight": "model-00008-of-00010.safetensors",
|
||||
"transformer.encoder.layers.30.mlp.dense_h_to_4h.weight": "model-00008-of-00010.safetensors",
|
||||
"transformer.encoder.layers.30.post_attention_layernorm.weight": "model-00008-of-00010.safetensors",
|
||||
"transformer.encoder.layers.30.self_attention.dense.weight": "model-00008-of-00010.safetensors",
|
||||
"transformer.encoder.layers.30.self_attention.query_key_value.bias": "model-00008-of-00010.safetensors",
|
||||
"transformer.encoder.layers.30.self_attention.query_key_value.weight": "model-00008-of-00010.safetensors",
|
||||
"transformer.encoder.layers.31.input_layernorm.weight": "model-00008-of-00010.safetensors",
|
||||
"transformer.encoder.layers.31.mlp.dense_4h_to_h.weight": "model-00008-of-00010.safetensors",
|
||||
"transformer.encoder.layers.31.mlp.dense_h_to_4h.weight": "model-00008-of-00010.safetensors",
|
||||
"transformer.encoder.layers.31.post_attention_layernorm.weight": "model-00008-of-00010.safetensors",
|
||||
"transformer.encoder.layers.31.self_attention.dense.weight": "model-00008-of-00010.safetensors",
|
||||
"transformer.encoder.layers.31.self_attention.query_key_value.bias": "model-00008-of-00010.safetensors",
|
||||
"transformer.encoder.layers.31.self_attention.query_key_value.weight": "model-00008-of-00010.safetensors",
|
||||
"transformer.encoder.layers.32.input_layernorm.weight": "model-00008-of-00010.safetensors",
|
||||
"transformer.encoder.layers.32.mlp.dense_4h_to_h.weight": "model-00008-of-00010.safetensors",
|
||||
"transformer.encoder.layers.32.mlp.dense_h_to_4h.weight": "model-00008-of-00010.safetensors",
|
||||
"transformer.encoder.layers.32.post_attention_layernorm.weight": "model-00008-of-00010.safetensors",
|
||||
"transformer.encoder.layers.32.self_attention.dense.weight": "model-00008-of-00010.safetensors",
|
||||
"transformer.encoder.layers.32.self_attention.query_key_value.bias": "model-00008-of-00010.safetensors",
|
||||
"transformer.encoder.layers.32.self_attention.query_key_value.weight": "model-00008-of-00010.safetensors",
|
||||
"transformer.encoder.layers.33.input_layernorm.weight": "model-00008-of-00010.safetensors",
|
||||
"transformer.encoder.layers.33.mlp.dense_4h_to_h.weight": "model-00008-of-00010.safetensors",
|
||||
"transformer.encoder.layers.33.mlp.dense_h_to_4h.weight": "model-00008-of-00010.safetensors",
|
||||
"transformer.encoder.layers.33.post_attention_layernorm.weight": "model-00008-of-00010.safetensors",
|
||||
"transformer.encoder.layers.33.self_attention.dense.weight": "model-00008-of-00010.safetensors",
|
||||
"transformer.encoder.layers.33.self_attention.query_key_value.bias": "model-00008-of-00010.safetensors",
|
||||
"transformer.encoder.layers.33.self_attention.query_key_value.weight": "model-00008-of-00010.safetensors",
|
||||
"transformer.encoder.layers.34.input_layernorm.weight": "model-00008-of-00010.safetensors",
|
||||
"transformer.encoder.layers.34.mlp.dense_4h_to_h.weight": "model-00009-of-00010.safetensors",
|
||||
"transformer.encoder.layers.34.mlp.dense_h_to_4h.weight": "model-00009-of-00010.safetensors",
|
||||
"transformer.encoder.layers.34.post_attention_layernorm.weight": "model-00008-of-00010.safetensors",
|
||||
"transformer.encoder.layers.34.self_attention.dense.weight": "model-00008-of-00010.safetensors",
|
||||
"transformer.encoder.layers.34.self_attention.query_key_value.bias": "model-00008-of-00010.safetensors",
|
||||
"transformer.encoder.layers.34.self_attention.query_key_value.weight": "model-00008-of-00010.safetensors",
|
||||
"transformer.encoder.layers.35.input_layernorm.weight": "model-00009-of-00010.safetensors",
|
||||
"transformer.encoder.layers.35.mlp.dense_4h_to_h.weight": "model-00009-of-00010.safetensors",
|
||||
"transformer.encoder.layers.35.mlp.dense_h_to_4h.weight": "model-00009-of-00010.safetensors",
|
||||
"transformer.encoder.layers.35.post_attention_layernorm.weight": "model-00009-of-00010.safetensors",
|
||||
"transformer.encoder.layers.35.self_attention.dense.weight": "model-00009-of-00010.safetensors",
|
||||
"transformer.encoder.layers.35.self_attention.query_key_value.bias": "model-00009-of-00010.safetensors",
|
||||
"transformer.encoder.layers.35.self_attention.query_key_value.weight": "model-00009-of-00010.safetensors",
|
||||
"transformer.encoder.layers.36.input_layernorm.weight": "model-00009-of-00010.safetensors",
|
||||
"transformer.encoder.layers.36.mlp.dense_4h_to_h.weight": "model-00009-of-00010.safetensors",
|
||||
"transformer.encoder.layers.36.mlp.dense_h_to_4h.weight": "model-00009-of-00010.safetensors",
|
||||
"transformer.encoder.layers.36.post_attention_layernorm.weight": "model-00009-of-00010.safetensors",
|
||||
"transformer.encoder.layers.36.self_attention.dense.weight": "model-00009-of-00010.safetensors",
|
||||
"transformer.encoder.layers.36.self_attention.query_key_value.bias": "model-00009-of-00010.safetensors",
|
||||
"transformer.encoder.layers.36.self_attention.query_key_value.weight": "model-00009-of-00010.safetensors",
|
||||
"transformer.encoder.layers.37.input_layernorm.weight": "model-00009-of-00010.safetensors",
|
||||
"transformer.encoder.layers.37.mlp.dense_4h_to_h.weight": "model-00009-of-00010.safetensors",
|
||||
"transformer.encoder.layers.37.mlp.dense_h_to_4h.weight": "model-00009-of-00010.safetensors",
|
||||
"transformer.encoder.layers.37.post_attention_layernorm.weight": "model-00009-of-00010.safetensors",
|
||||
"transformer.encoder.layers.37.self_attention.dense.weight": "model-00009-of-00010.safetensors",
|
||||
"transformer.encoder.layers.37.self_attention.query_key_value.bias": "model-00009-of-00010.safetensors",
|
||||
"transformer.encoder.layers.37.self_attention.query_key_value.weight": "model-00009-of-00010.safetensors",
|
||||
"transformer.encoder.layers.38.input_layernorm.weight": "model-00009-of-00010.safetensors",
|
||||
"transformer.encoder.layers.38.mlp.dense_4h_to_h.weight": "model-00009-of-00010.safetensors",
|
||||
"transformer.encoder.layers.38.mlp.dense_h_to_4h.weight": "model-00009-of-00010.safetensors",
|
||||
"transformer.encoder.layers.38.post_attention_layernorm.weight": "model-00009-of-00010.safetensors",
|
||||
"transformer.encoder.layers.38.self_attention.dense.weight": "model-00009-of-00010.safetensors",
|
||||
"transformer.encoder.layers.38.self_attention.query_key_value.bias": "model-00009-of-00010.safetensors",
|
||||
"transformer.encoder.layers.38.self_attention.query_key_value.weight": "model-00009-of-00010.safetensors",
|
||||
"transformer.encoder.layers.39.input_layernorm.weight": "model-00009-of-00010.safetensors",
|
||||
"transformer.encoder.layers.39.mlp.dense_4h_to_h.weight": "model-00010-of-00010.safetensors",
|
||||
"transformer.encoder.layers.39.mlp.dense_h_to_4h.weight": "model-00010-of-00010.safetensors",
|
||||
"transformer.encoder.layers.39.post_attention_layernorm.weight": "model-00010-of-00010.safetensors",
|
||||
"transformer.encoder.layers.39.self_attention.dense.weight": "model-00010-of-00010.safetensors",
|
||||
"transformer.encoder.layers.39.self_attention.query_key_value.bias": "model-00010-of-00010.safetensors",
|
||||
"transformer.encoder.layers.39.self_attention.query_key_value.weight": "model-00010-of-00010.safetensors",
|
||||
"transformer.encoder.layers.4.input_layernorm.weight": "model-00002-of-00010.safetensors",
|
||||
"transformer.encoder.layers.4.mlp.dense_4h_to_h.weight": "model-00002-of-00010.safetensors",
|
||||
"transformer.encoder.layers.4.mlp.dense_h_to_4h.weight": "model-00002-of-00010.safetensors",
|
||||
"transformer.encoder.layers.4.post_attention_layernorm.weight": "model-00002-of-00010.safetensors",
|
||||
"transformer.encoder.layers.4.self_attention.dense.weight": "model-00002-of-00010.safetensors",
|
||||
"transformer.encoder.layers.4.self_attention.query_key_value.bias": "model-00002-of-00010.safetensors",
|
||||
"transformer.encoder.layers.4.self_attention.query_key_value.weight": "model-00002-of-00010.safetensors",
|
||||
"transformer.encoder.layers.5.input_layernorm.weight": "model-00002-of-00010.safetensors",
|
||||
"transformer.encoder.layers.5.mlp.dense_4h_to_h.weight": "model-00002-of-00010.safetensors",
|
||||
"transformer.encoder.layers.5.mlp.dense_h_to_4h.weight": "model-00002-of-00010.safetensors",
|
||||
"transformer.encoder.layers.5.post_attention_layernorm.weight": "model-00002-of-00010.safetensors",
|
||||
"transformer.encoder.layers.5.self_attention.dense.weight": "model-00002-of-00010.safetensors",
|
||||
"transformer.encoder.layers.5.self_attention.query_key_value.bias": "model-00002-of-00010.safetensors",
|
||||
"transformer.encoder.layers.5.self_attention.query_key_value.weight": "model-00002-of-00010.safetensors",
|
||||
"transformer.encoder.layers.6.input_layernorm.weight": "model-00002-of-00010.safetensors",
|
||||
"transformer.encoder.layers.6.mlp.dense_4h_to_h.weight": "model-00003-of-00010.safetensors",
|
||||
"transformer.encoder.layers.6.mlp.dense_h_to_4h.weight": "model-00003-of-00010.safetensors",
|
||||
"transformer.encoder.layers.6.post_attention_layernorm.weight": "model-00002-of-00010.safetensors",
|
||||
"transformer.encoder.layers.6.self_attention.dense.weight": "model-00002-of-00010.safetensors",
|
||||
"transformer.encoder.layers.6.self_attention.query_key_value.bias": "model-00002-of-00010.safetensors",
|
||||
"transformer.encoder.layers.6.self_attention.query_key_value.weight": "model-00002-of-00010.safetensors",
|
||||
"transformer.encoder.layers.7.input_layernorm.weight": "model-00003-of-00010.safetensors",
|
||||
"transformer.encoder.layers.7.mlp.dense_4h_to_h.weight": "model-00003-of-00010.safetensors",
|
||||
"transformer.encoder.layers.7.mlp.dense_h_to_4h.weight": "model-00003-of-00010.safetensors",
|
||||
"transformer.encoder.layers.7.post_attention_layernorm.weight": "model-00003-of-00010.safetensors",
|
||||
"transformer.encoder.layers.7.self_attention.dense.weight": "model-00003-of-00010.safetensors",
|
||||
"transformer.encoder.layers.7.self_attention.query_key_value.bias": "model-00003-of-00010.safetensors",
|
||||
"transformer.encoder.layers.7.self_attention.query_key_value.weight": "model-00003-of-00010.safetensors",
|
||||
"transformer.encoder.layers.8.input_layernorm.weight": "model-00003-of-00010.safetensors",
|
||||
"transformer.encoder.layers.8.mlp.dense_4h_to_h.weight": "model-00003-of-00010.safetensors",
|
||||
"transformer.encoder.layers.8.mlp.dense_h_to_4h.weight": "model-00003-of-00010.safetensors",
|
||||
"transformer.encoder.layers.8.post_attention_layernorm.weight": "model-00003-of-00010.safetensors",
|
||||
"transformer.encoder.layers.8.self_attention.dense.weight": "model-00003-of-00010.safetensors",
|
||||
"transformer.encoder.layers.8.self_attention.query_key_value.bias": "model-00003-of-00010.safetensors",
|
||||
"transformer.encoder.layers.8.self_attention.query_key_value.weight": "model-00003-of-00010.safetensors",
|
||||
"transformer.encoder.layers.9.input_layernorm.weight": "model-00003-of-00010.safetensors",
|
||||
"transformer.encoder.layers.9.mlp.dense_4h_to_h.weight": "model-00003-of-00010.safetensors",
|
||||
"transformer.encoder.layers.9.mlp.dense_h_to_4h.weight": "model-00003-of-00010.safetensors",
|
||||
"transformer.encoder.layers.9.post_attention_layernorm.weight": "model-00003-of-00010.safetensors",
|
||||
"transformer.encoder.layers.9.self_attention.dense.weight": "model-00003-of-00010.safetensors",
|
||||
"transformer.encoder.layers.9.self_attention.query_key_value.bias": "model-00003-of-00010.safetensors",
|
||||
"transformer.encoder.layers.9.self_attention.query_key_value.weight": "model-00003-of-00010.safetensors",
|
||||
"transformer.output_layer.weight": "model-00010-of-00010.safetensors",
|
||||
"transformer.rotary_pos_emb.inv_freq": "model-00001-of-00010.safetensors"
|
||||
}
|
||||
}
|
1138
modeling_chatglm.py
1138
modeling_chatglm.py
File diff suppressed because it is too large
Load Diff
|
@ -1,224 +0,0 @@
|
|||
import regex as re
|
||||
import base64
|
||||
import os
|
||||
import tiktoken
|
||||
from typing import List, Optional, Union, Dict
|
||||
from transformers import PreTrainedTokenizer
|
||||
from transformers.utils import PaddingStrategy
|
||||
from transformers.tokenization_utils_base import EncodedInput, BatchEncoding
|
||||
|
||||
|
||||
class ChatGLM4Tokenizer(PreTrainedTokenizer):
|
||||
vocab_files_names = {"vocab_file": "tokenizer.model"}
|
||||
model_input_names = ["input_ids", "attention_mask", "position_ids"]
|
||||
|
||||
def __init__(
|
||||
self,
|
||||
vocab_file,
|
||||
clean_up_tokenization_spaces=False,
|
||||
**kwargs
|
||||
):
|
||||
self.name = "GLM4Tokenizer"
|
||||
self.vocab_file = vocab_file
|
||||
pat_str = "(?i:'s|'t|'re|'ve|'m|'ll|'d)|[^\\r\\n\\p{L}\\p{N}]?\\p{L}+|\\p{N}{1,3}| ?[^\\s\\p{L}\\p{N}]+[\\r\\n]*|\\s*[\\r\\n]+|\\s+(?!\\S)|\\s+"
|
||||
self.pat_str = re.compile(pat_str)
|
||||
|
||||
mergeable_ranks = {}
|
||||
with open(vocab_file) as f:
|
||||
for line in f:
|
||||
token, rank = line.strip().split()
|
||||
rank = int(rank)
|
||||
token = base64.b64decode(token)
|
||||
mergeable_ranks[token] = rank
|
||||
|
||||
self.mergeable_ranks = mergeable_ranks
|
||||
|
||||
self.tokenizer = tiktoken.Encoding(
|
||||
name="my_tokenizer",
|
||||
pat_str=pat_str,
|
||||
mergeable_ranks=mergeable_ranks,
|
||||
special_tokens={}
|
||||
)
|
||||
self.decoder = {rank: token for token, rank in mergeable_ranks.items()}
|
||||
self.n_words = len(self.decoder)
|
||||
|
||||
super().__init__(
|
||||
clean_up_tokenization_spaces=clean_up_tokenization_spaces,
|
||||
**kwargs
|
||||
)
|
||||
|
||||
@property
|
||||
def vocab_size(self):
|
||||
return self.n_words
|
||||
|
||||
def get_vocab(self):
|
||||
""" Returns vocab as a dict """
|
||||
vocab = {self._convert_id_to_token(i): i for i in range(self.vocab_size)}
|
||||
vocab.update(self.added_tokens_encoder)
|
||||
return vocab
|
||||
|
||||
def convert_tokens_to_string(self, tokens: List[Union[bytes, str, int]]) -> str:
|
||||
"""
|
||||
Converts a sequence of tokens in a single string.
|
||||
"""
|
||||
text = ""
|
||||
temp = b""
|
||||
for t in tokens:
|
||||
if isinstance(t, int):
|
||||
t = chr(t)
|
||||
if isinstance(t, str):
|
||||
if temp:
|
||||
text += temp.decode("utf-8", errors="replace")
|
||||
elif isinstance(t, bytes):
|
||||
temp += t
|
||||
else:
|
||||
raise TypeError("token should only be of type int, bytes or str")
|
||||
if temp:
|
||||
text += temp.decode("utf-8", errors="replace")
|
||||
return text
|
||||
|
||||
def _tokenize(self, text, **kwargs):
|
||||
tokens = []
|
||||
ids = self.tokenizer.encode(text)
|
||||
for t in ids:
|
||||
tokens.append(self.decoder[t])
|
||||
return tokens
|
||||
|
||||
def _convert_token_to_id(self, token):
|
||||
""" Converts a token (str) in an id using the vocab. """
|
||||
return self.mergeable_ranks[token]
|
||||
|
||||
def _convert_id_to_token(self, index):
|
||||
"""Converts an index (integer) in a token (str) using the vocab."""
|
||||
return self.decoder.get(index, "")
|
||||
|
||||
def save_vocabulary(self, save_directory, filename_prefix=None):
|
||||
"""
|
||||
Save the vocabulary and special tokens file to a directory.
|
||||
|
||||
Args:
|
||||
save_directory (`str`):
|
||||
The directory in which to save the vocabulary.
|
||||
filename_prefix (`str`, *optional*):
|
||||
An optional prefix to add to the named of the saved files.
|
||||
|
||||
Returns:
|
||||
`Tuple(str)`: Paths to the files saved.
|
||||
"""
|
||||
if os.path.isdir(save_directory):
|
||||
vocab_file = os.path.join(
|
||||
save_directory, self.vocab_files_names["vocab_file"]
|
||||
)
|
||||
else:
|
||||
vocab_file = save_directory
|
||||
|
||||
with open(self.vocab_file, 'rb') as fin:
|
||||
proto_str = fin.read()
|
||||
|
||||
with open(vocab_file, "wb") as writer:
|
||||
writer.write(proto_str)
|
||||
|
||||
return (vocab_file,)
|
||||
|
||||
def get_prefix_tokens(self):
|
||||
prefix_tokens = [self.convert_tokens_to_ids("[gMASK]"), self.convert_tokens_to_ids("<sop>")]
|
||||
return prefix_tokens
|
||||
|
||||
def build_single_message(self, role, metadata, message, tokenize=True):
|
||||
assert role in ["system", "user", "assistant", "observation"], role
|
||||
if tokenize:
|
||||
role_tokens = [self.convert_tokens_to_ids(f"<|{role}|>")] + self.tokenizer.encode(f"{metadata}\n",
|
||||
disallowed_special=())
|
||||
message_tokens = self.tokenizer.encode(message, disallowed_special=())
|
||||
tokens = role_tokens + message_tokens
|
||||
return tokens
|
||||
else:
|
||||
return str(f"<|{role}|>{metadata}\n{message}")
|
||||
|
||||
def build_inputs_with_special_tokens(
|
||||
self, token_ids_0: List[int], token_ids_1: Optional[List[int]] = None
|
||||
) -> List[int]:
|
||||
"""
|
||||
Build model inputs from a sequence or a pair of sequence for sequence classification tasks by concatenating and
|
||||
adding special tokens. A BERT sequence has the following format:
|
||||
|
||||
- single sequence: `[CLS] X [SEP]`
|
||||
- pair of sequences: `[CLS] A [SEP] B [SEP]`
|
||||
|
||||
Args:
|
||||
token_ids_0 (`List[int]`):
|
||||
List of IDs to which the special tokens will be added.
|
||||
token_ids_1 (`List[int]`, *optional*):
|
||||
Optional second list of IDs for sequence pairs.
|
||||
|
||||
Returns:
|
||||
`List[int]`: List of [input IDs](../glossary#input-ids) with the appropriate special tokens.
|
||||
"""
|
||||
prefix_tokens = self.get_prefix_tokens()
|
||||
token_ids_0 = prefix_tokens + token_ids_0
|
||||
if token_ids_1 is not None:
|
||||
token_ids_0 = token_ids_0 + token_ids_1 + [self.convert_tokens_to_ids("<eos>")]
|
||||
return token_ids_0
|
||||
|
||||
def _pad(
|
||||
self,
|
||||
encoded_inputs: Union[Dict[str, EncodedInput], BatchEncoding],
|
||||
max_length: Optional[int] = None,
|
||||
padding_side: str = "left",
|
||||
padding_strategy: PaddingStrategy = PaddingStrategy.DO_NOT_PAD,
|
||||
pad_to_multiple_of: Optional[int] = None,
|
||||
return_attention_mask: Optional[bool] = None,
|
||||
) -> dict:
|
||||
"""
|
||||
Pad encoded inputs (on left/right and up to predefined length or max length in the batch)
|
||||
|
||||
Args:
|
||||
encoded_inputs:
|
||||
Dictionary of tokenized inputs (`List[int]`) or batch of tokenized inputs (`List[List[int]]`).
|
||||
max_length: maximum length of the returned list and optionally padding length (see below).
|
||||
Will truncate by taking into account the special tokens.
|
||||
padding_strategy: PaddingStrategy to use for padding.
|
||||
|
||||
- PaddingStrategy.LONGEST Pad to the longest sequence in the batch
|
||||
- PaddingStrategy.MAX_LENGTH: Pad to the max length (default)
|
||||
- PaddingStrategy.DO_NOT_PAD: Do not pad
|
||||
The tokenizer padding sides are defined in self.padding_side:
|
||||
|
||||
- 'left': pads on the left of the sequences
|
||||
- 'right': pads on the right of the sequences
|
||||
pad_to_multiple_of: (optional) Integer if set will pad the sequence to a multiple of the provided value.
|
||||
This is especially useful to enable the use of Tensor Core on NVIDIA hardware with compute capability
|
||||
`>= 7.5` (Volta).
|
||||
return_attention_mask:
|
||||
(optional) Set to False to avoid returning attention mask (default: set to model specifics)
|
||||
"""
|
||||
# Load from model defaults
|
||||
|
||||
required_input = encoded_inputs[self.model_input_names[0]]
|
||||
seq_length = len(required_input)
|
||||
|
||||
if padding_strategy == PaddingStrategy.LONGEST:
|
||||
max_length = len(required_input)
|
||||
|
||||
if max_length is not None and pad_to_multiple_of is not None and (max_length % pad_to_multiple_of != 0):
|
||||
max_length = ((max_length // pad_to_multiple_of) + 1) * pad_to_multiple_of
|
||||
|
||||
needs_to_be_padded = padding_strategy != PaddingStrategy.DO_NOT_PAD and len(required_input) != max_length
|
||||
|
||||
# Initialize attention mask if not present.
|
||||
if "attention_mask" not in encoded_inputs:
|
||||
encoded_inputs["attention_mask"] = [1] * seq_length
|
||||
|
||||
if "position_ids" not in encoded_inputs:
|
||||
encoded_inputs["position_ids"] = list(range(seq_length))
|
||||
|
||||
if needs_to_be_padded:
|
||||
difference = max_length - len(required_input)
|
||||
|
||||
if "attention_mask" in encoded_inputs:
|
||||
encoded_inputs["attention_mask"] = [0] * difference + encoded_inputs["attention_mask"]
|
||||
if "position_ids" in encoded_inputs:
|
||||
encoded_inputs["position_ids"] = [0] * difference + encoded_inputs["position_ids"]
|
||||
encoded_inputs[self.model_input_names[0]] = [self.pad_token_id] * difference + required_input
|
||||
|
||||
return encoded_inputs
|
BIN
tokenizer.model (Stored with Git LFS)
BIN
tokenizer.model (Stored with Git LFS)
Binary file not shown.
|
@ -1,134 +0,0 @@
|
|||
{
|
||||
"auto_map": {
|
||||
"AutoTokenizer": [
|
||||
"tokenization_chatglm.ChatGLM4Tokenizer",
|
||||
null
|
||||
]
|
||||
},
|
||||
"added_tokens_decoder": {
|
||||
"151329": {
|
||||
"content": "<|endoftext|>",
|
||||
"lstrip": false,
|
||||
"normalized": false,
|
||||
"rstrip": false,
|
||||
"single_word": false,
|
||||
"special": true
|
||||
},
|
||||
"151330": {
|
||||
"content": "[MASK]",
|
||||
"lstrip": false,
|
||||
"normalized": false,
|
||||
"rstrip": false,
|
||||
"single_word": false,
|
||||
"special": true
|
||||
},
|
||||
"151331": {
|
||||
"content": "[gMASK]",
|
||||
"lstrip": false,
|
||||
"normalized": false,
|
||||
"rstrip": false,
|
||||
"single_word": false,
|
||||
"special": true
|
||||
},
|
||||
"151332": {
|
||||
"content": "[sMASK]",
|
||||
"lstrip": false,
|
||||
"normalized": false,
|
||||
"rstrip": false,
|
||||
"single_word": false,
|
||||
"special": true
|
||||
},
|
||||
"151333": {
|
||||
"content": "<sop>",
|
||||
"lstrip": false,
|
||||
"normalized": false,
|
||||
"rstrip": false,
|
||||
"single_word": false,
|
||||
"special": true
|
||||
},
|
||||
"151334": {
|
||||
"content": "<eop>",
|
||||
"lstrip": false,
|
||||
"normalized": false,
|
||||
"rstrip": false,
|
||||
"single_word": false,
|
||||
"special": true
|
||||
},
|
||||
"151335": {
|
||||
"content": "<|system|>",
|
||||
"lstrip": false,
|
||||
"normalized": false,
|
||||
"rstrip": false,
|
||||
"single_word": false,
|
||||
"special": true
|
||||
},
|
||||
"151336": {
|
||||
"content": "<|user|>",
|
||||
"lstrip": false,
|
||||
"normalized": false,
|
||||
"rstrip": false,
|
||||
"single_word": false,
|
||||
"special": true
|
||||
},
|
||||
"151337": {
|
||||
"content": "<|assistant|>",
|
||||
"lstrip": false,
|
||||
"normalized": false,
|
||||
"rstrip": false,
|
||||
"single_word": false,
|
||||
"special": true
|
||||
},
|
||||
"151338": {
|
||||
"content": "<|observation|>",
|
||||
"lstrip": false,
|
||||
"normalized": false,
|
||||
"rstrip": false,
|
||||
"single_word": false,
|
||||
"special": true
|
||||
},
|
||||
"151339": {
|
||||
"content": "<|begin_of_image|>",
|
||||
"lstrip": false,
|
||||
"normalized": false,
|
||||
"rstrip": false,
|
||||
"single_word": false,
|
||||
"special": true
|
||||
},
|
||||
"151340": {
|
||||
"content": "<|end_of_image|>",
|
||||
"lstrip": false,
|
||||
"normalized": false,
|
||||
"rstrip": false,
|
||||
"single_word": false,
|
||||
"special": true
|
||||
},
|
||||
"151341": {
|
||||
"content": "<|begin_of_video|>",
|
||||
"lstrip": false,
|
||||
"normalized": false,
|
||||
"rstrip": false,
|
||||
"single_word": false,
|
||||
"special": true
|
||||
},
|
||||
"151342": {
|
||||
"content": "<|end_of_video|>",
|
||||
"lstrip": false,
|
||||
"normalized": false,
|
||||
"rstrip": false,
|
||||
"single_word": false,
|
||||
"special": true
|
||||
}
|
||||
},
|
||||
"additional_special_tokens": ["<|endoftext|>", "[MASK]", "[gMASK]", "[sMASK]", "<sop>", "<eop>", "<|system|>",
|
||||
"<|user|>", "<|assistant|>", "<|observation|>", "<|begin_of_image|>", "<|end_of_image|>",
|
||||
"<|begin_of_video|>", "<|end_of_video|>"],
|
||||
"clean_up_tokenization_spaces": false,
|
||||
"chat_template": "[gMASK]<sop>{% for item in messages %}{% if item['tools'] is defined %}<|system|>\n你是一个名为 GLM-4 的人工智能助手。你是基于智谱AI训练的语言模型 GLM-4 模型开发的,你的任务是针对用户的问题和要求提供适当的答复和支持。\n\n# 可用工具{% set tools = item['tools'] %}{% for tool in tools %}{% if tool['type'] == 'function' %}\n\n## {{ tool['function']['name'] }}\n\n{{ tool['function'] | tojson(indent=4) }}\n在调用上述函数时,请使用 Json 格式表示调用的参数。{% elif tool['type'] == 'python' %}\n\n## python\n\n当你向 `python` 发送包含 Python 代码的消息时,该代码将会在一个有状态的 Jupyter notebook 环境中执行。\n`python` 返回代码执行的输出,或在执行 60 秒后返回超时。\n`/mnt/data` 将会持久化存储你的文件。在此会话中,`python` 无法访问互联网。不要使用 `python` 进行任何网络请求或者在线 API 调用,这些在线内容的访问将不会成功。{% elif tool['type'] == 'simple_browser' %}\n\n## simple_browser\n\n你可以使用 `simple_browser` 工具。该工具支持以下函数:\n`search(query: str, recency_days: int)`:使用搜索引擎进行查询并显示结果,可以使用 `recency_days` 参数控制搜索内容的时效性。\n`mclick(ids: list[int])`:获取一系列指定 id 的页面内容。每次调用时,须选择3-10个页面。选择多个角度的页面,同时尽可能选择可信任的信息来源。考虑到部分页面是无法加载的,你也可以多打开一些可能有用的页面而不用担心内容过多。\n`open_url(url: str)`:打开指定的 URL。\n\n使用 `【{引用 id}†{引用文本}】` 来引用内容。\n\n操作步骤:1. 使用 `search` 来获得信息列表; 2. 使用 `mclick` 来获取指定 ID 页面的内容; 3. 根据获得的内容进行回复。在回复中应当引用信息来源。\n 如果用户提供了 URL,也可以用 `open_url` 直接打开页面。\n如果初次搜索结果没有找到合适的信息,也可以再次使用 `search` 进行搜索。{% elif tool['type'] == 'cogview' %}\n\n## cogview\n\n如果用户的请求中包含了对图像的描述,你可以使用 `cogview` 来生成图像并展示给用户。你需要向 `cogview` 发送图像描述,规则:\n- 发送给 `cogview` 的消息必须使用英语。用户的中文描述必须完全翻译为英语。\n- 应当尽可能详细地描述图像生成的需求,需求描述约 100 英文单词。\n- 保持用户原始描述的意图。不要虚构内容或者没见过的人物。\n- 如无特殊说明,所在地为中国,持有中国立场并遵循中国社会主义价值观。{% endif %}{% endfor %}{% endif %}{% if item['content'] %}<|{{ item['role'] }}|>{{ item['metadata'] }}\n{{ item['content'] }}{% endif %}{% endfor %}{% if add_generation_prompt %}<|assistant|>{% endif %}",
|
||||
"do_lower_case": false,
|
||||
"eos_token": "<|endoftext|>",
|
||||
"pad_token": "<|endoftext|>",
|
||||
"model_max_length": 128000,
|
||||
"padding_side": "left",
|
||||
"remove_space": false,
|
||||
"tokenizer_class": "ChatGLM4Tokenizer"
|
||||
}
|
Loading…
Reference in New Issue