tags |
license |
widget |
image-to-text |
image-captioning |
|
apache-2.0 |
|
nlpconnect/vit-gpt2-image-captioning
This is an image captioning model trained by @ydshieh in flax this is pytorch version of this.
The Illustrated Image Captioning using transformers
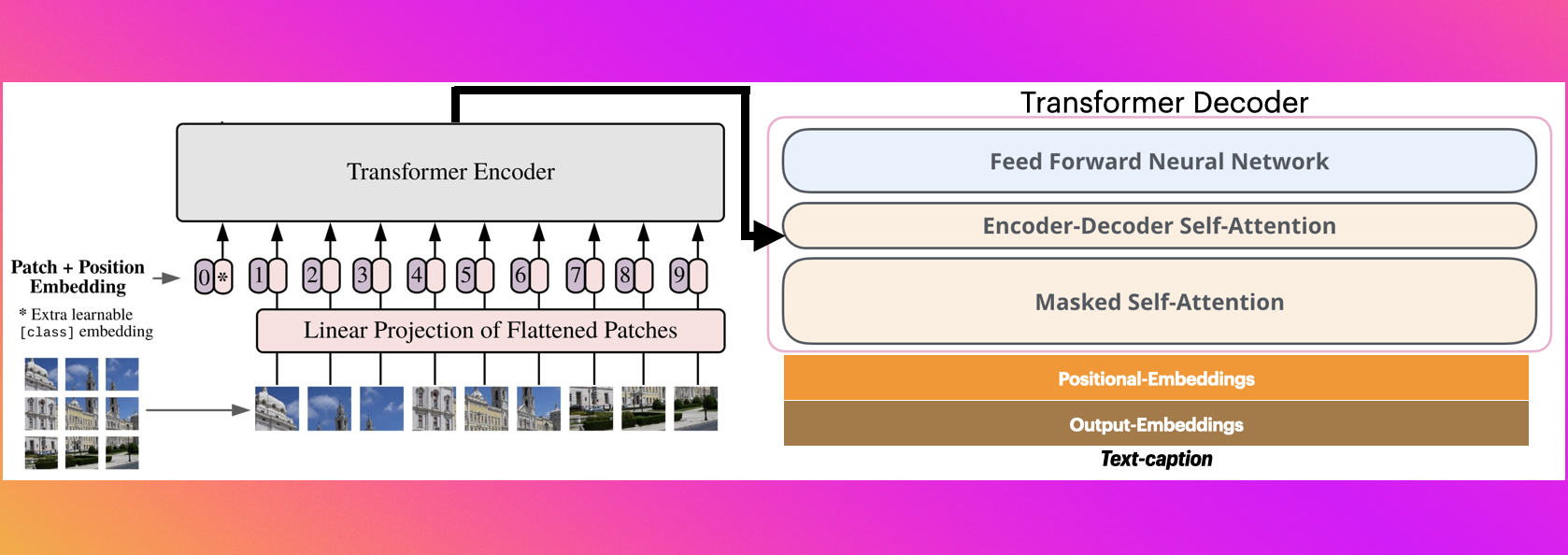
Sample running code
from transformers import VisionEncoderDecoderModel, ViTImageProcessor, AutoTokenizer
import torch
from PIL import Image
model = VisionEncoderDecoderModel.from_pretrained("nlpconnect/vit-gpt2-image-captioning")
feature_extractor = ViTImageProcessor.from_pretrained("nlpconnect/vit-gpt2-image-captioning")
tokenizer = AutoTokenizer.from_pretrained("nlpconnect/vit-gpt2-image-captioning")
device = torch.device("cuda" if torch.cuda.is_available() else "cpu")
model.to(device)
max_length = 16
num_beams = 4
gen_kwargs = {"max_length": max_length, "num_beams": num_beams}
def predict_step(image_paths):
images = []
for image_path in image_paths:
i_image = Image.open(image_path)
if i_image.mode != "RGB":
i_image = i_image.convert(mode="RGB")
images.append(i_image)
pixel_values = feature_extractor(images=images, return_tensors="pt").pixel_values
pixel_values = pixel_values.to(device)
output_ids = model.generate(pixel_values, **gen_kwargs)
preds = tokenizer.batch_decode(output_ids, skip_special_tokens=True)
preds = [pred.strip() for pred in preds]
return preds
predict_step(['doctor.e16ba4e4.jpg']) # ['a woman in a hospital bed with a woman in a hospital bed']
Sample running code using transformers pipeline
from transformers import pipeline
image_to_text = pipeline("image-to-text", model="nlpconnect/vit-gpt2-image-captioning")
image_to_text("https://ankur3107.github.io/assets/images/image-captioning-example.png")
# [{'generated_text': 'a soccer game with a player jumping to catch the ball '}]
Contact for any help